Online first
Short communication
Published online: 2024-07-08
Wavelet transform analysis reveals differences between patients with impaired LVEF and healthy individuals
Abstract
Not available
References
- Savarese G, Becher PM, Lund LH, et al. Global burden of heart failure: a comprehensive and updated review of epidemiology. Cardiovasc Res. 2023; 118(17): 3272–3287.
- Lippi G, Sanchis-Gomar F. Global epidemiology and future trends of heart failure. AME Medical Journal. 2020; 5(15).
- Kaufmann D, Szwoch M, Kwiatkowska J, et al. Global longitudinal strain can predict heart failure exacerbation in stable outpatients with ischemic left ventricular systolic dysfunction. PLoS One. 2019; 14(12): e0225829.
- Gruszecki M, Lancaster G, Stefanovska A, et al. Human subarachnoid space width oscillations in the resting state. Sci Rep. 2018; 8(1): 3057.
- Holme NL, Zilakos I, Elstad M, et al. Cerebral blood flow response to cardiorespiratory oscillations in healthy humans. Auton Neurosci. 2023; 245.
- Lancaster G, Iatsenko D, Pidde A, et al. Surrogate data for hypothesis testing of physical systems. Physics Reports. 2018; 748: 1–60.
- Bernjak A, Stefanovska A, McClintock PVE, et al. Coherence between fluctuations in blood flow and oxygen saturation. Fluctuation and Noise Letters. 2012; 11(1).
- Levy BI. Artery changes with aging: degeneration or adaptation? Dialog Cardiovas Med. 2001; 6: 104–111.
- Sciomer S, Moscucci F, Salvioni E, et al. Role of gender, age and BMI in prognosis of heart failure. Eur J Prev Cardiol. 2020; 27(2_suppl): 46–51.
- Powell-Wiley TM, Poirier P, Burke LE, et al. American Heart Association Council on Lifestyle and Cardiometabolic Health; Council on Cardiovascular and Stroke Nursing; Council on Clinical Cardiology; Council on Epidemiology and Prevention; and Stroke Council. Obesity and cardiovascular disease: a scientific statement from the American Heart Association. Circulation. 2021; 143(21): e984–e1010.
- Biscay F, Arini PD, Rincón Soler AI, et al. Classification of ischemic and non-ischemic cardiac events in Holter recordings based on the continuous wavelet transform. Med Biol Eng Comput. 2020; 58(5): 1069–1078.
- Rocha T, Paredes S, Carvalho P, et al. A wavelet-based approach for time series pattern detection and events prediction applied to telemonitoring data. Annu Int Conf IEEE Eng Med Biol Soc. 2011; 2011: 6037–6040.
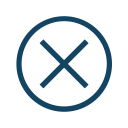