An overview of the role of artificial intelligence in palliative care: a quasi-systematic review
Abstract
Background: Recently, there has been a dramatic increase in research on the use of artificial intelligence (AI) in medicine, uncovering new areas for its application. However, palliative care continues to make limited use of these tools, despite promising results from various models that could significantly improve the quality of palliative care and optimize health resources. This review aims to summarize the current literature on applying AI techniques, with particular focus on machine learning (ML), in palliative care practice, and to analyze their performance rates and usability.
Methods: Quasi-systematic review; PubMed and Scopus databases were searched utilizing selected MeSH terms.
Results: A total of 17 sources were included in the review. The literature used ML for mortality forecast (n = 8), predicting demands, nonvisible symptoms, and delirium (n = 3), identification of phases in palliative care status (n = 1), communication and information supply (n = 4), clinical decision support system (n = 1). Most analyzed techniques achieved good performance rates, however, communication skills and providing reliable information in the field of palliative care were still insufficient.
Conclusions: Machine learning in palliative care is mainly used to predict mortality, however, other forecasts are gradually being introduced. AI-based models are used as clinical decision support and in the assessment of a patient’s palliative care status. Another potentially important future role of AI is in communication and presenting information to patients, provided certain improvements are made to existing models.
Keywords: artificial intelligencemachine learningpalliative carepalliative medicine
References
- Gero JS, Sudweeks F. Artificial intelligence in design ’96. Springer Netherlands, Dordrecht 1996: 1.
- Esteva A, Robicquet A, Ramsundar B, et al. A guide to deep learning in healthcare. Nat Med. 2019; 25(1): 24–29.
- Karpathakis K, Morley J, Floridi L. A justifiable investment in AI for healthcare: aligning ambition with reality. Minds Machin. 2024; 34(4): 38.
- Sarma G, Kashya H, Medhi P, et al. Unravelling the landscape of image-guided radiotherapy: a comprehensive overview. Palliat Med Pract. 2024.
- Windisch P, Hertler C, Blum D, et al. Leveraging advances in artificial intelligence to improve the quality and timing of palliative care. Cancers (Basel). 2020; 12(5): 1149.
- Wicki S, Clark IC, Amann M, et al. Acceptance of digital health technologies in palliative care patients. Palliat Med Rep. 2024; 5(1): 34–42.
- Page MJ, McKenzie JE, Bossuyt PM, et al. The PRISMA 2020 statement: an updated guideline for reporting systematic reviews. BMJ. 2021; 372(71).
- Blanes-Selva V, Doñate-Martínez A, Linklater G, et al. Responsive and minimalist app based on explainable AI to assess palliative care needs during bedside consultations on older patients. Sustainability. 2021; 13(17): 9844.
- Blanes-Selva V, Doñate-Martínez A, Linklater G, et al. Complementary frailty and mortality prediction models on older patients as a tool for assessing palliative care needs. Health Informatics J. 2022; 28(2): 14604582221092592.
- Wang L, Sha L, Lakin JR, et al. Development and validation of a deep learning algorithm for mortality prediction in selecting patients with dementia for earlier palliative care interventions. JAMA Netw Open. 2019; 2(7): e196972.
- Zhang H, Li Y, McConnell W. Predicting potential palliative care beneficiaries for health plans: a generalized machine learning pipeline. J Biomed Inform. 2021; 123: 103922.
- Sandham MH, Hedgecock EA, Siegert RJ, et al. Intelligent palliative care based on patient-reported outcome measures. J Pain Symptom Manage. 2022; 63(5): 747–757.
- Mori M, Yamaguchi T, Maeda I, et al. Diagnostic models for impending death in terminally ill cancer patients: a multicenter cohort study. Cancer Med. 2021; 10(22): 7988–7995.
- Gajra A, Zettler ME, Miller KA, et al. Impact of augmented intelligence on utilization of palliative care services in a real-world oncology setting. JCO Oncol Pract. 2022; 18(1): e80–e88.
- Liu JH, Shih CY, Huang HL, et al. Evaluating the potential of machine learning and wearable devices in end-of-life care in predicting 7-day death events among patients with terminal cancer: cohort study. J Med Internet Res. 2023; 25: e47366.
- Zhuang Q, Zhang AY, Cong RS, et al. Towards proactive palliative care in oncology: developing an explainable EHR-based machine learning model for mortality risk prediction. BMC Palliat Care. 2024; 23(1): 124.
- Soltani M, Farahmand M, Pourghaderi AR. Machine learning-based demand forecasting in cancer palliative care home hospitalization. J Biomed Inform. 2022; 130: 104075.
- Shimada K, Tsuneto S. Novel method for predicting nonvisible symptoms using machine learning in cancer palliative care. Sci Rep. 2023; 13(1): 12088.
- Kim YuJ, Lee H, Woo HoG, et al. Machine learning-based model to predict delirium in patients with advanced cancer treated with palliative care: a multicenter, patient-based registry cohort. Sci Rep. 2024; 14(1): 11503.
- Srivastava R, Srivastava S. Can Artificial Intelligence aid communication? Considering the possibilities of GPT-3 in palliative care. Indian J Palliat Care. 2023; 29(4): 418–425.
- Gondode PG, Mahor V, Rani D, et al. Debunking palliative care myths: assessing the performance of artificial intelligence chatbots (ChatGPT vs. Google gemini). Indian J Palliat Care. 2024; 30(3): 284–287.
- Hancı V, Ergün B, Gül Ş, et al. Assessment of readability, reliability, and quality of ChatGPT®, BARD®, Gemini®, Copilot®, Perplexity® responses on palliative care. Medicine (Baltimore). 2024; 103(33): e39305.
- Lazris D, Schenker Y, Thomas TH. AI-generated content in cancer symptom management: a comparative analysis between chatgpt and NCCN. J Pain Symptom Manage. 2024; 68(4): e303–e311.
- Blanes-Selva V, Asensio-Cuesta S, Doñate-Martínez A, et al. User-centred design of a clinical decision support system for palliative care: Insights from healthcare professionals. Digit Health. 2023; 9: 20552076221150735.
- Kamarudin AN, Cox T, Kolamunnage-Dona R. Time-dependent ROC curve analysis in medical research: current methods and applications. BMC Med Res Methodol. 2017; 17(1): 53.
- Çorbacıoğlu ŞK, Aksel G. Receiver operating characteristic curve analysis in diagnostic accuracy studies: a guide to interpreting the area under the curve value. Turk J Emerg Med. 2023; 23(4): 195–198.
- Lau F, Downing GM, Lesperance M, et al. Use of palliative performance scale in end-of-life prognostication. J Palliat Med. 2006; 9(5): 1066–1075.
- Temel JS, Greer JA, Muzikansky A, et al. Early palliative care for patients with metastatic non-small-cell lung cancer. N Engl J Med. 2010; 363(8): 733–742.
- Parsons MW, Dietrich J. Assessment and management of cognitive changes in patients with cancer. Cancer. 2019; 125(12): 1958–1962.
- Sands MB, Wee I, Agar M, et al. The detection of delirium in admitted oncology patients: a scoping review. Eur Geriatr Med. 2022; 13(1): 33–51.
- Back AL. Patient-Clinician communication issues in palliative care for patients with advanced cancer. J Clin Oncol. 2020; 38(9): 866–876.
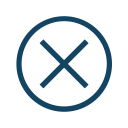