Association between cognitive impairment and risk of atrial fibrillation: The Atherosclerosis Risk in Communities study
Abstract
Background: Atrial fibrillation (AF) is reportedly a risk factor for cognitive impairment. Interestingly, recent studies have emphasized that impaired cognition is probably an initiating factor of cardiovascular disease. Thus, we aimed to explore the association between impaired cognition and the risk of AF, and clarify the potential mechanisms. Methods: Participants of visit 2 (1991–1993) in the Atherosclerosis Risk in Communities study were included. Global cognition z-scores and factor scores were calculated using the word fluency, delayed word recall, and digit symbol substitution tests. AF incidents were diagnosed by electrocardiography and inpatient records. The association of cognitive decline with AF risk and left atrial volume index (LAVI) was explored using Cox proportional hazards and linear regression models, respectively. Results: During the median follow-up of 18.2 ± 6.2 years, 2056/11,675 (17.6%) participants developed AF. Participants in the lowest quartile of global cognition z- and factor scores had a higher risk of AF (hazard ratio [HR]: 1.271, 95% confidence interval [CI]: 1.094–1.477, p = 0.002; HR: 1.305, 95% CI: 1.110–1.535, p = 0.001, respectively) than those in the highest quartile. Global cognition z- and factor scores were negatively correlated with the LAVI (B: –0.411, 95% CI: –0.749 to –0.074, p = 0.017; B: –0.425, 95% CI: –0.833 to –0.017, p = 0.041, respectively). Conclusions: Cognitive decline is significantly associated with a higher risk of AF, with atrial remodeling being a potential mechanism. Our results extend previous findings of the brain-heart axis and indicate the effects of cognitive injury on cardiac function and structure. Registration: URL: https://www.clinicaltrials.gov; unique identifier: NCT00005131
Keywords: cognitive functionatrial fibrillationatrial remodelingstrokeAtherosclerosis Risk in Communities study
References
- Dai H, Zhang Q, Much AA, et al. Global, regional, and national prevalence, incidence, mortality, and risk factors for atrial fibrillation, 1990-2017: results from the Global Burden of Disease Study 2017. Eur Heart J Qual Care Clin Outcomes. 2021; 7(6): 574–582.
- Go AS, Hylek EM, Phillips KA, et al. Prevalence of diagnosed atrial fibrillation in adults: national implications for rhythm management and stroke prevention: the AnTicoagulation and Risk Factors in Atrial Fibrillation (ATRIA) Study. JAMA. 2001; 285(18): 2370–2375.
- Wolf PA, Abbott RD, Kannel WB. Atrial fibrillation as an independent risk factor for stroke: the Framingham Study. Stroke. 1991; 22(8): 983–988.
- Boyle PM, Del Álamo JC, Akoum N. Fibrosis, atrial fibrillation and stroke: clinical updates and emerging mechanistic models. Heart. 2021; 107(2): 99–105.
- Magnani JW, Norby FL, Agarwal SK, et al. Racial Differences in Atrial Fibrillation-Related Cardiovascular Disease and Mortality: The Atherosclerosis Risk in Communities (ARIC) Study. JAMA Cardiol. 2016; 1(4): 433–441.
- Wang TJ, Larson MG, Levy D, et al. Temporal relations of atrial fibrillation and congestive heart failure and their joint influence on mortality: the Framingham Heart Study. Circulation. 2003; 107(23): 2920–2925.
- Haegeli LM, Calkins H. Catheter ablation of atrial fibrillation: an update. Eur Heart J. 2014; 35(36): 2454–2459.
- Weerasooriya R, Khairy P, Litalien J, et al. Catheter ablation for atrial fibrillation: are results maintained at 5 years of follow-up? J Am Coll Cardiol. 2011; 57(2): 160–166.
- Harvey P. Domains of cognition and their assessment. Dialogues Cin Neurosci. 2019; 21(3): 227–237.
- Eshkoor SA, Hamid TA, Mun CY, et al. Mild cognitive impairment and its management in older people. Clin Interv Aging. 2015; 10: 687–693.
- Singh-Manoux A, Fayosse A, Sabia S, et al. Atrial fibrillation as a risk factor for cognitive decline and dementia. Eur Heart J. 2017; 38(34): 2612–2618.
- Chen LY, Norby FL, Gottesman RF, et al. Association of Atrial Fibrillation With Cognitive Decline and Dementia Over 20 Years: The ARIC-NCS (Atherosclerosis Risk in Communities Neurocognitive Study). J Am Heart Assoc. 2018; 7(6).
- Bressler J, Knopman DS, Sharrett AR, et al. Incident Heart Failure and Cognitive Decline: The Atherosclerosis Risk in Communities Study. J Card Fail. 2017; 23(1): 47–55.
- Singh-Manoux A, Sabia S, Lajnef M, et al. History of coronary heart disease and cognitive performance in midlife: the Whitehall II study. Eur Heart J. 2008; 29(17): 2100–2107.
- Sposato LA, Hilz MJ, Aspberg S, et al. Post-Stroke cardiovascular complications and neurogenic cardiac injury: JACC state-of-the-art review. J Am Coll Cardiol. 2020; 76(23): 2768–2785.
- Lee M, Oh JuH, Lee KB, et al. Clinical and echocardiographic characteristics of acute cardiac dysfunction associated with acute brain hemorrhage - difference from takotsubo cardiomyopathy. Circ J. 2016; 80(9): 2026–2032.
- Adeoye AM, Ogah OS, Ovbiagele B, et al. Prevalence and prognostic features of ECG abnormalities in acute stroke: findings from the SIREN study among africans. Glob Heart. 2017; 12(2): 99–105.
- Li D, Jia Yu, Yu J, et al. Cognition impairment and risk of subclinical cardiovascular disease in older adults: The atherosclerosis risk in communities study. Front Aging Neurosci. 2022; 14: 889543.
- Nattel S, Burstein B, Dobrev D. Atrial remodeling and atrial fibrillation: mechanisms and implications. Circ Arrhythm Electrophysiol. 2008; 1(1): 62–73.
- Zhu L, Fratiglioni L, Guo Z, et al. Incidence of stroke in relation to cognitive function and dementia in the Kungsholmen Project. Neurology. 2000; 54(11): 2103–2107.
- Rajan KB, Aggarwal NT, Wilson RS, et al. Association of cognitive functioning, incident stroke, and mortality in older adults. Stroke. 2014; 45(9): 2563–2567.
- The ARIC investigators. The Atherosclerosis Risk in Communities (ARIC) Study: design and objectives. Am J Epidemiol. 1989; 129(4): 687–702.
- Cerhan JR, Folsom AR, Mortimer JA, et al. Correlates of cognitive function in middle-aged adults. Atherosclerosis Risk in Communities (ARIC) Study Investigators. Gerontology. 1998; 44(2): 95–105.
- Magnani JW, Norby FL, Agarwal SK, et al. Incidence of atrial fibrillation in whites and African-Americans: the Atherosclerosis Risk in Communities (ARIC) study. Am Heart J. 2009; 158(1): 111–117.
- Shah AM, Cheng S, Skali H, et al. Rationale and design of a multicenter echocardiographic study to assess the relationship between cardiac structure and function and heart failure risk in a biracial cohort of community-dwelling elderly persons: the Atherosclerosis Risk in Communities study. Circ Cardiovasc Imaging. 2014; 7(1): 173–181.
- Lang RM, Badano LP, Mor-Avi V, et al. Recommendations for cardiac chamber quantification by echocardiography in adults: an update from the American Society of Echocardiography and the European Association of Cardiovascular Imaging. J Am Soc Echocardiogr. 2015; 28(1): 1–39.e14.
- Rosamond WD, Folsom AR, Chambless LE, et al. Stroke incidence and survival among middle-aged adults: 9-year follow-up of the Atherosclerosis Risk in Communities (ARIC) cohort. Stroke. 1999; 30(4): 736–743.
- Koton S, Schneider ALC, Windham BG, et al. Microvascular brain disease progression and risk of stroke: the ARIC study. Stroke. 2020; 51(11): 3264–3270.
- Stein C, Morris N, Hall N, et al. Structural equation modeling. Methods Mol Biol. 2017; 1666: 557–580.
- Hu X, Gu S, Zhen X, et al. Trends in cognitive function among chinese elderly from 1998 to 2018: an age-period-cohort analysis. Front Public Health. 2021; 9: 753671.
- Moheet A, Mangia S, Seaquist ER. Impact of diabetes on cognitive function and brain structure. Ann N Y Acad Sci. 2015; 1353: 60–71.
- Iadecola C, Gottesman RF. Neurovascular and cognitive dysfunction in hypertension. Circ Res. 2019; 124(7): 1025–1044.
- Avila JF, Vonk JMJ, Verney SP, et al. Sex/gender differences in cognitive trajectories vary as a function of race/ethnicity. Alzheimers Dement. 2019; 15(12): 1516–1523.
- Fillit H, Nash DT, Rundek T, et al. Cardiovascular risk factors and dementia. Am J Geriatr Pharmacother. 2008; 6(2): 100–118.
- Alonso A, Jacobs DR, Menotti A, et al. Cardiovascular risk factors and dementia mortality: 40 years of follow-up in the Seven Countries Study. J Neurol Sci. 2009; 280(1-2): 79–83.
- Jia L, Du Y, Chu L, et al. Prevalence, risk factors, and management of dementia and mild cognitive impairment in adults aged 60 years or older in China: a cross-sectional study. Lancet Public Health. 2020; 5(12): e661–e671.
- Kornej J, Börschel CS, Benjamin EJ, et al. Epidemiology of atrial fibrillation in the 21st century: novel methods and new insights. Circ Res. 2020; 127(1): 4–20.
- Wiberg B, Lind L, Kilander L, et al. Cognitive function and risk of stroke in elderly men. Neurology. 2010; 74(5): 379–385.
- Harris SE, Malik R, Marioni R, et al. Polygenic risk of ischemic stroke is associated with cognitive ability. Neurology. 2016; 86(7): 611–618.
- Woodford HJ, George J. Cognitive assessment in the elderly: a review of clinical methods. QJM. 2007; 100(8): 469–484.
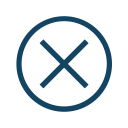