open access
Efficacy of artificial intelligence in detecting diabetic retinopathy from retinal fundus images — a systematic review
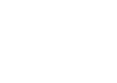
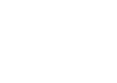
- Lithuanian University of Health Sciences, Kaunas, Lithuania
- Department of Ophthalmology, Lithuanian University of Health Sciences, Kaunas, Lithuania
open access
Abstract
Background: Diabetic retinopathy (DR) is a microvascular disorder that damages the retina’s blood vessels. This review aims to evaluate scientific literature about the efficacy of artificial intelligence (AI) in detecting diabetic retinopathy from retinal fundus images.
Material and methods: Systematic literature review was carried out following preferred reporting items for systematic reviews and meta-analyses (PRISMA) guidelines. Selected Medical Subject Headings (MeSH) keywords were used to search “PubMed” and “ScienceDirect” databases. Inclusion and exclusion criteria were developed using the patient, intervention, comparison, outcome (PICO) tool. Quality assessment of selected studies was done using a modified seven-item checklist based on the methodological index for nonrandomized studies (MINORS) criteria.
Results: 15 studies from 14 scientific publications were included in this systematic review. AI algorithms analyzed
a total of 150179 images. The AI-based algorithm’s average sensitivity (Se) was 92.58 %, ranging from 76.2% to 100%. The average specificity (Sp) was 87.22%, with the lowest of 53.16% and the highest of 98.5%. The average area under the receiver operating characteristic (AUROC) curve was 0.937, with a variation of 0.843 to 0.9905.
Conclusion: Our results show that AI-based algorithms can accurately detect DR in retinal fundus images. These systems should be considered of use in clinical practice to save time and reduce costs.
Abstract
Background: Diabetic retinopathy (DR) is a microvascular disorder that damages the retina’s blood vessels. This review aims to evaluate scientific literature about the efficacy of artificial intelligence (AI) in detecting diabetic retinopathy from retinal fundus images.
Material and methods: Systematic literature review was carried out following preferred reporting items for systematic reviews and meta-analyses (PRISMA) guidelines. Selected Medical Subject Headings (MeSH) keywords were used to search “PubMed” and “ScienceDirect” databases. Inclusion and exclusion criteria were developed using the patient, intervention, comparison, outcome (PICO) tool. Quality assessment of selected studies was done using a modified seven-item checklist based on the methodological index for nonrandomized studies (MINORS) criteria.
Results: 15 studies from 14 scientific publications were included in this systematic review. AI algorithms analyzed
a total of 150179 images. The AI-based algorithm’s average sensitivity (Se) was 92.58 %, ranging from 76.2% to 100%. The average specificity (Sp) was 87.22%, with the lowest of 53.16% and the highest of 98.5%. The average area under the receiver operating characteristic (AUROC) curve was 0.937, with a variation of 0.843 to 0.9905.
Conclusion: Our results show that AI-based algorithms can accurately detect DR in retinal fundus images. These systems should be considered of use in clinical practice to save time and reduce costs.
Keywords
ophthalmology; diabetic retinopathy; artificial intelligence; machine learning; neural networks; deep learning
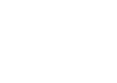
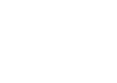
Title
Efficacy of artificial intelligence in detecting diabetic retinopathy from retinal fundus images — a systematic review
Journal
Issue
Vol 7 (2022): Continuous Publishing
Article type
Review paper
Pages
144-151
Published online
2022-09-05
Page views
3961
Article views/downloads
430
DOI
Bibliographic record
Ophthalmol J 2022;7:144-151.
Keywords
ophthalmology
diabetic retinopathy
artificial intelligence
machine learning
neural networks
deep learning
Authors
Gabrielius Dailidė
Saulius Lagunavičius
Vilma Jūratė Balčiūnienė
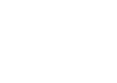
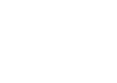
- Shukla U, Tripathy K. Diabetic Retinopathy. StatPearls, Treasure Island 2022.
- Bourne RR, Steinmetz JD, Saylan M, et al. Causes of blindness and vision impairment in 2020 and trends over 30 years, and prevalence of avoidable blindness in relation to VISION 2020: the Right to Sight: an analysis for the Global Burden of Disease Study. Lancet Glob Health. 2021; 9(2): e144–e160..
- Ting DS, Pasquale LR, Peng L, et al. Artificial intelligence and deep learning in ophthalmology. Br J Ophthalmol. 2019; 103(2): 167–175.
- WHO Global Report on Diabetes. Global Report on Diabetes.2016;978:6–86. https://sci-hub.si/https://apps.who.int/iris/handle/10665/204874%0Ahttps://apps.who.int/iris/bitstream/handle/10665/204874/WHO_NMH_NVI_16.3_eng.pdf?sequence=1%0Ahttp://www.who.int/about/licensing/copyright_form/index.html%0Ahttp://www.who.int/about/licens.
- Yau JWY, Rogers SL, Kawasaki R, et al. Meta-Analysis for Eye Disease (META-EYE) Study Group. Global prevalence and major risk factors of diabetic retinopathy. Diabetes Care. 2012; 35(3): 556–564.
- Kanasi E, Ayilavarapu S, Jones J. The aging population: demographics and the biology of aging. Periodontol 2000. 2016; 72(1): 13–18.
- Ramesh AN, Kambhampati C, Monson JRT, et al. Artificial intelligence in medicine. Ann R Coll Surg Engl. 2004; 86(5): 334–338.
- Keskinbora K, Güven F. Artificial Intelligence and Ophthalmology. Turk J Ophthalmol. 2020; 50(1): 37–43.
- Page MJ, McKenzie JE, Bossuyt PM, et al. The PRISMA 2020 statement: An updated guideline for reporting systematic reviews. PLoS Med. 2021; 18(3): e1003583–189.
- Eriksen MB, Frandsen TF. The impact of patient, intervention, comparison, outcome (PICO) as a search strategy tool on literature search quality: a systematic review. J Med Libr Assoc. 2018; 106(4): 420–431.
- Park SHo, Goo JMo, Jo CH. Receiver operating characteristic (ROC) curve: practical review for radiologists. Korean J Radiol. 2004; 5(1): 11–18.
- Obuchowski NA. Receiver operating characteristic curves and their use in radiology. Radiology. 2003; 229(1): 3–8.
- Janssens AC, Martens FK. Reflection on modern methods: Revisiting the area under the ROC Curve. Int J Epidemiol. 2020; 49(4): 1397–1403.
- Langerhuizen DWG, Janssen SJ, Mallee WH, et al. What Are the Applications and Limitations of Artificial Intelligence for Fracture Detection and Classification in Orthopaedic Trauma Imaging? A Systematic Review. Clin Orthop Relat Res. 2019; 477(11): 2482–2491.
- Pei X, Yao Xi, Yang Y, et al. Efficacy of artificial intelligence-based screening for diabetic retinopathy in type 2 diabetes mellitus patients. Diabetes Res Clin Pract. 2022; 184: 109190.
- Ming S, Xie K, Lei X, et al. Evaluation of a novel artificial intelligence-based screening system for diabetic retinopathy in community of China: a real-world study. Int Ophthalmol. 2021; 41(4): 1291–1299.
- Zhang X, Li F, Wei Q, et al. Automated detection of severe diabetic retinopathy using deep learning method. Graefes Arch Clin Exp Ophthalmol. 2022; 260(3): 849–856.
- Li F, Liu Z, Chen H, et al. Automatic Detection of Diabetic Retinopathy in Retinal Fundus Photographs Based on Deep Learning Algorithm. Transl Vis Sci Technol. 2019; 8(6): 4.
- Arenas-Cavalli JT, Abarca I, Rojas-Contreras M, et al. Clinical validation of an artificial intelligence-based diabetic retinopathy screening tool for a national health system. Eye (Lond). 2022; 36(1): 78–85.
- Roychowdhury S, Koozekanani DD, Parhi KK. DREAM: diabetic retinopathy analysis using machine learning. IEEE J Biomed Health Inform. 2014; 18(5): 1717–1728.
- Wang XN, Dai L, Li ST, et al. Automatic Grading System for Diabetic Retinopathy Diagnosis Using Deep Learning Artificial Intelligence Software. Curr Eye Res. 2020; 45(12): 1550–1555.
- Wang Y, Yu M, Hu B, et al. Deep learning-based detection and stage grading for optimising diagnosis of diabetic retinopathy. Diabetes Metab Res Rev. 2021; 37(4): e3445.
- He J, Cao T, Xu F, et al. Artificial intelligence-based screening for diabetic retinopathy at community hospital. Eye (Lond). 2020; 34(3): 572–576.
- Gargeya R, Leng T. Automated Identification of Diabetic Retinopathy Using Deep Learning. Ophthalmology. 2017; 124(7): 962–969.
- Saxena G, Verma D, Paraye A, et al. Improved and robust deep learning agent for preliminary detection of diabetic retinopathy using public datasets. Intelligence-Based Med. 2020; 3-4: 100022.
- Baget-Bernaldiz M, Pedro RA, Santos-Blanco E, et al. Testing a Deep Learning Algorithm for Detection of Diabetic Retinopathy in a Spanish Diabetic Population and with MESSIDOR Database. Diagnostics (Basel). 2021; 11(8).
- Shah P, Mishra DK, Shanmugam MP, et al. Validation of Deep Convolutional Neural Network-based algorithm for detection of diabetic retinopathy - Artificial intelligence versus clinician for screening. Indian J Ophthalmol. 2020; 68(2): 398–405.
- Cao P, Ren F, Wan C, et al. Efficient multi-kernel multi-instance learning using weakly supervised and imbalanced data for diabetic retinopathy diagnosis. Comput Med Imaging Graph. 2018; 69: 112–124.
- Zhang X, Li F, Li D, et al. Automated detection of severe diabetic retinopathy using deep learning method. Graefes Arch Clin Exp Ophthalmol. 2022; 260(3): 849–856.
- Tufail A, Rudisill C, Egan C, et al. Automated Diabetic Retinopathy Image Assessment Software: Diagnostic Accuracy and Cost-Effectiveness Compared with Human Graders. Ophthalmology. 2017; 124(3): 343–351.
- Wong TY, Bressler NM. Artificial Intelligence With Deep Learning Technology Looks Into Diabetic Retinopathy Screening. JAMA. 2016; 316(22): 2366–2367.
- Goh JK, Cheung CY, Sim SS, et al. Retinal Imaging Techniques for Diabetic Retinopathy Screening. J Diabetes Sci Technol. 2016; 10(2): 282–294.
- Sommer A, Taylor HR, Ravilla TD, et al. Council of the American Ophthalmological Society. Challenges of ophthalmic care in the developing world. JAMA Ophthalmol. 2014; 132(5): 640–644.
- Ting DS, Pasquale LR, Peng L, et al. Artificial intelligence and deep learning in ophthalmology. Br J Ophthalmol. 2019; 103(2): 167–175.
- He J, Cao T, Xu F, et al. Artificial intelligence-based screening for diabetic retinopathy at community hospital. Eye (Lond). 2020; 34(3): 572–576.
- Hassan B, Hassan T, Li Bo, et al. Deep Ensemble Learning Based Objective Grading of Macular Edema by Extracting Clinically Significant Findings from Fused Retinal Imaging Modalities. Sensors (Basel). 2019; 19(13).
- Bellemo V, Lim G, Rim TH, et al. Artificial Intelligence Screening for Diabetic Retinopathy: the Real-World Emerging Application. Curr Diab Rep. 2019; 19(9): 72.