RESEARCH PAPER
Neurologia i Neurochirurgia Polska
Polish Journal of Neurology and Neurosurgery
2023, Volume 57, no. 3, pages: 297–304
DOI: 10.5603/PJNNS.a2023.0036
Copyright © 2023 Polish Neurological Society
ISSN: 0028-3843, e-ISSN: 1897-4260
Address for correspondence: Christian F. Altmann, Parkinson-Klinik Ortenau,Kreuzbergstr. 12–16, 77709 Wolfach, Germany;
e-mail: chfaltmann@gmail.com
Received: 4.02.2022 Accepted: 13.04.2023 Early publication date: 10.05.2023
Predictors of falls in Parkinson’s disease, progressive supranuclear palsy, and multiple system atrophy:
a retrospective study
Christian F. Altmann1, Jiri Koschel1, Wolfgang H. Jost1, 2
1Parkinson-Klinik Ortenau, Wolfach, Germany
2Department of Neurology, University of Saarland, Homburg/Saar, Germany
Abstract Introduction. Recurrent falling is a major clinical milestone in Parkinsonian syndromes. It has a detrimental impact on quality of life, further prognosis, and life expectancy. Aim of the study. To improve fall management and prevention, we aimed at identifying clinical parameters predicting fall frequency. To this end, we retrospectively analysed records of fall events of patients with Parkinson’s disease (PD), or progressive supranuclear palsy (PSP), or multiple system atrophy (MSA), during their two-week inpatient stay at the Parkinson-Klinik Ortenau, Wolfach, Germany. This data served as an objective proxy for patients’ fall frequency and allowed us to estimate the impact of several demographic and clinical variables on the occurrence of falling. Material and methods. Of 2,111 patients admitted to our hospital, 1,810 presented with PD, 191 with PSP, and 110 with MSA. We employed a multiple (quasi-) poisson regression analysis to model the fall frequency as a function of various demographic variables (age at diagnosis, gender) and clinical variables (disease duration and sub-type, motor and cognitive impairment, autonomic dysfunction). Results. Statistically significant predictors for falls in PD were cognitive impairment, motor impairment, and autonomic dysfunction. In PSP, significant predictors for falls were motor and autonomic dysfunction, while in MSA only disease duration predicted falls, but with only marginal statistical significance. Conclusions. Our results stress the importance of different factors in predicting falls in the different types of Parkinsonian syndrome. Preventive interventions should address these disease-specific targets for optimal success. Key words: autonomic dysfunction, cognitive impairment, falls, multiple system atrophy, Parkinson’s disease, progressive supranuclear palsy (Neurol Neurochir Pol 2023; 57 (3): 297–304) |
Introduction
The occurrence of falling is a major clinical milestone in the progression of Parkinsonian syndromes [1]. While in progressive supranuclear palsy (PSP) falling occurs early and is a diagnostic feature [2], falls occur later in the course of Parkinson’s disease (PD). Specifically, the median time to the first fall has been reported to be 1.4 years in PSP, but 9 years in PD [3]. In multiple system atrophy (MSA), the first fall has been estimated to occur at a median of 3.5 years after diagnosis.
Alongside the occurrence of dementia [4], falling has been described as a major detrimental factor in patients with Parkinsonian syndromes, affecting their quality of life negatively [5]. A survey among Parkinsonian patients, including PD and atypical Parkinsonian syndromes, has found that after falling, 65% of patients sustained injuries. Of these, 33% sustained fractures [6]. Falling, and in particular recurrent falls and their resulting injuries, are a major risk factor for nursing home admissions [7]. The early occurrence of falls has been described as being predictive of shorter survival in both MSA and PSP [8].
Table 1. Patient demographics and clinical characteristics
Variable |
PSP |
MSA |
PD |
N |
191 |
110 |
1,810 |
Gender (female:male) |
77:114 40.3:59.7% |
65:45 59.1:40.9% |
673:1,137 37.2:62.8% |
Age at diagnosis in years; mean ± SD (range) |
68.7 ± 7.0 (47–86) |
63.1 ± 9.4 (37–84) |
64.0 ± 10.5 (30–89) |
Disease duration in years; mean ± SD (range) |
2.6 ± 2.5 (0–20) |
3.2 ± 2.9 (0–17) |
7.8 ± 5.9 (0–46) |
Previous studies have usually relied on retrospective chart reviews [3], post-hoc rating scales and questionnaires [9–11], or self-report diaries [12] to estimate fall rates in Parkinsonian syndromes. A major disadvantage of post-hoc self-reports is the possibility for recall bias. The occurrence of falls is likely to be underreported, patients and their caretakers might downplay fall events, and might only recall those falls resulting in injuries as being genuine falls.
Recording fall events of inpatients in a clinical setting has the advantage of standardised criteria as to what constitutes a fall event, and should thus provide an objective estimate of fall frequency.
In order to understand the occurrence of falls in Parkinsonian syndromes and to obtain possible insights into the mechanisms of falling, we retrospectively analysed data of 2,111 patients with PSP, MSA, or PD who were admitted as inpatients to the Parkinson-Klinik Ortenau, Wolfach, Germany between 2015 and 2020. As a part of standard clinical routine, we obtained various clinical parameters, describing the disease progression and its effects on motor, cognitive, and autonomic function. On average, patients stayed for about two weeks at our hospital, and many of them experienced fall events during that time. The aim of our data analysis was to identify predictors by modelling the fall rate at our hospital with regression analysis as a function of various demographic and clinical parameters. The fall rate observed at our hospital was thus used as an objective proxy measure for fall probability in general.
Material and methods
Participants
We retrospectively surveyed data of inpatients admitted to the Parkinson-Klinik Ortenau, Wolfach, Germany in 2015–2020. Inpatients were included in this study if they had been diagnosed with a) PSP, or b) MSA, or c) PD. PSP was diagnosed according to the criteria given in the National Institute for Neurological Disorders and Society for PSP [13] and the criteria devised by Höglinger et al. [14]. The diagnostic criteria for MSA were those of Gilman et al. [15]. The level of diagnostic certainty for PSP was “probable PSP” according to Höglinger et al. [14] and “probable MSA” following the guidelines described in Gilman et al. [15]. Diagnoses were supported by neuroimaging (magnetic-resonance imaging and positron-emission tomography), but neuropathological evidence was not available. Diagnosis of PD relied on the UK Brain Bank criteria [16] and the criteria published by Postuma et al. [17] and were sub-classified, with PSP being distinguished into PSP-Richardson’s syndrome (PSP-RS), PSP with predominant parkinsonism (PSP-P), or others (see Höglinger et al. [14] for sub-type definitions).
MSA was sub-typed according to the guidelines described by Gilman et al. [15] into MSA with predominant cerebellar ataxia (MSA-C), or MSA with predominant Parkinson motor features (MSA-P).
PD patients were classified into tremor-dominant, akinetic-rigid, or mixed motor phenotypes (see [17]).
We identified 2,111 patients fulfilling the requirements (see Table 1 for patient characteristics). All patients received medication and various therapies (physical, speech, and occupational therapy; psychosocial counselling) according to best practice. Detailed information on prescribed medication was not collected for this study sample. See the supplementary material of Altmann et al. [19] for typical prescriptions of anti-Parkinson, anti-dementia, anti-psychotic, and anti-depressant drugs in a sample of PD patients at the same clinic. The procedures used in this study were in accordance with the ethical guidelines laid down in the Declaration of Helsinki 1964, and were approved by the local ethics committee of the State Medical Chamber of Baden-Württemberg, Germany (F-2021-151).
Assessment and procedure
Data on demographics was obtained from medical records. Disease severity was assessed with the Unified PD Rating Scale (UPDRS, [20]). As measures of motor function, we analysed part III of the UPDRS and the Berg Balance Scale (BBS, Berg et al., 1989 [21]; Scherfer et al. [22]). Global cognitive function was assessed with the Montreal Cognitive Assessment (MoCA, [23]), a diagnostic tool widely used in screening for cognitive impairment in PD [24]. Frontal lobe functions were tested with the Frontal Assessment Battery (FAB; Dubois et al., 2002 [25]).
To measure autonomic function, orthostatic hypotension (OH) was tested with the modified Schellong test [26]. The UPDRS was performed by neurologists, the BBS by physical therapists, the cognitive test by neuropsychologists, and the Schellong test by medical technical assistants. All examiners were experienced in applying the tests to patients with Parkinsonian syndromes. Fall events were recorded as part of clinical routine by any staff member who witnessed the event or its outcome, and were confirmed by a neurologist. Falls were defined as “an unexpected event in which the person comes to rest on the ground, floor or lower level” [27].
Statistical analysis
Fall frequency was computed by dividing the number of falls of a patient during his or her inpatient treatment by the number of days he or she was an inpatient. In the first analysis, the distribution of fall frequencies was calculated as a function of disease duration (in years). To assess correlations among clinical parameters, Pearson’s correlation coefficients were computed and tested for significance computing a test statistic t and comparing to Student’s t distribution (degrees of freedom: n-2). An α = 0.05 was defined as a critical value for statistical significance.
An analysis of predictive factors was performed with multiple Poisson regression models, which are the canonical statistical model used for count data such as falls (see e.g. [12]). Calculation of these models was conducted in R (www.r-project.org, version 3.5.2) employing the glm function. For model selection, we followed a formal model-building procedure [28], as follows:
- We started with a Poisson regression model to explain the number of falls during the inpatient residency as a function of various variables, including the demographic factors: age at first diagnosis and gender; disease duration and sub-type (sub-types being for PSP: PSP-RS, PSP-P, and PSP-others; for MSA: MSA-C and MSA-P; and for PD: akinetic-rigid, mixed, and tremor-dominant); motor parameters: UPDRS (part III), the BBS; cognitive parameters (MoCA and FAB scores); and autonomic dysfunction: OH.
- We then tested the Poisson model for over-dispersion (i.e. for empirical variance not equal to the mean of the data) and the necessity for an additional parameter accounting for this. We tested for over-dispersion with a χ2-test, with α = 0.05 as the critical value. Over-dispersion was evident in all cases and therefore quasi-Poisson models were employed that entailed an additional coefficient by which the variance in the model is greater than the mean, as described in Ver Hoef and Boveng [29].
- We then employed backward stepwise deletion and removed all predictors from the resulting model that did not reach significance in a t-test on the regression coefficients (α = 0.05). Overall performance of the final models was evaluated with an Omnibus test and an R2 value describing the explained variance [28].
Figure 1. Histogram of falls during hospital stay as a function of disease duration for PSP, MSA, and PD patients, measured in years since diagnosis
Results
Data from 2,111 patients was analysed for this study. The patients’ clinical characteristics are set out in Table 1. Frequencies of the different sub-types of Parkinsonian syndromes are shown in Supplementary Table 1. Figure 1 depicts the fall rate as a function of disease duration. Fall rates were highest early for PSP, increased with disease duration, and showed a slight decrease in advanced disease stages (4+ years). For MSA, fall rates were higher than for PD, and for both MSA and PD fall rates increased monotonically with disease duration for the disease stages observed in this study.
We obtained several clinical parameters as potential predictors for falls. Table 2 sets out correlations among these predictors. High correlations were observed between the two cognitive parameters, i.e. between the test scores of MoCA and FAB, and for the motor parameters, i.e. between the UPDRS (part III) and the BBS. To avoid collinearity in the regression model, only one of the cognitive parameters (the MoCA test score) was considered for further analysis. Results of the regression model including the FAB instead of the MoCA are set out separately in Supplementary Table 2. The strongest correlation with disease duration was observed for the UPDRS (part III)-score, in particular for MSA patients.
We analysed factors predicting fall rates separately for the different types of Parkinsonian syndrome (PSP, MSA, and PD). Statistically significant coefficients for the final multiple (quasi)-poisson regression models are set out in Table 3. Cognitive, motor, and autonomic factors were predictive for falls in PD, whereas for PSP, only the motor and autonomic factors predicted falls significantly. Sub-types of Parkinsonian syndromes were not significantly predictive for falls in this study. However, for PSP, the factor sub-type reached marginal significance (p = 0.059), with a tendency towards a higher fall rate in Richardson’s Syndrome. The omnibus tests for the full models were significant for PSP and PD, but revealed only a marginally significant result for MSA, with disease duration as the only significant predictor for fall rate.
Our analysis was mainly focused on modelling fall frequency during the hospital stay as an inpatient. However, many previous studies have differentiated between frequent fallers and less frequent fallers [9, 11] or between fallers and non-fallers [12, 29] and have therefore represented falls as binary data. To allow for comparability with these previous reports, we show demographic and clinical parameters for fallers and non-fallers in Table 4.
Table 2. Pearson’s correlation coefficients between parameters
Parameter pair |
PSP |
MSA |
PD |
MoCA ~ FAB |
0.74*** |
0.79*** |
0.83*** |
UPDRS-III ~ BBS |
–0.58*** |
–0.66*** |
–0.60*** |
MoCA ~ disease duration |
–0.003 |
–0.08 |
–0.13*** |
FAB ~ disease duration |
0.005 |
–0.16 |
–0.14*** |
UPDRS-III ~ disease duration |
0.30*** |
0.39*** |
0.29*** |
BBS ~ disease duration |
–0.23** |
–0.24* |
–0.22*** |
OH ~ disease duration |
0.14 |
–0.02 |
0.13*** |
* — p < 0.05; ** — p < 0.01; *** — p < 0.001
Table 3. Multiple quasi-Poisson regression models
Parameter |
PSP |
MSA |
PD |
N |
146 |
110 |
1540 |
MoCA |
— |
— |
–0.059**** |
BBS |
— |
— |
–0.028**** |
UPDRS-III |
0.027*** |
— |
0.012* |
OH |
0.018* |
— |
0.012*** |
Gender |
— |
— |
— |
Sub-type |
— |
— |
— |
Age at diagnosis |
— |
— |
— |
Disease duration |
— |
0.112* |
— |
Intercept |
–4.328*** |
–3.828*** |
–2.81*** |
Dispersion parameter |
3.17 |
2.26 |
2.10 |
* — p < 0.05; ** — p < 0.01; *** — p < 0.001. Omnibus test p-values and R2: PSP p < 0.0001, R2 = 0.114; MSA p = 0.055, R2 = 0.061; PD p < 0.0001, R2 = 0.060; dispersion parameters > 1 indicate overdispersion. Please note that sample sizes (N) can deviate from those in Table 1, because not all patients received all clinical tests (e.g. some patients had no MoCA or OH score)
Table 4. Comparison of demographic and clinical parameters between non-fallers (0 falls during hospital stay) and fallers (≥ 1 fall during hospital stay)
PSP |
MSA |
PD |
||||
Falls = 0 |
Falls ≥ 1 |
Falls = 0 |
Falls ≥ 1 |
Falls = 0 |
Falls ≥ 1 |
|
N |
99 |
92 |
84 |
26 |
1,529 |
281 |
Gender (f:m) |
32:67 32:68% |
45:47 49:51% |
53:31 63:37% |
12:14 46:54% |
565:964 37:63% |
108:173 38:62% |
Sub-type* |
21:54:24 21:55:24% |
46:28:18 50:30:20% |
17:67 20:80% |
6:20 23:77% |
923:546:60 60:36:4% |
198:79:4 70:28:2% |
Age at diagnosis [years] |
69.3 ± 6.8 |
68.1 ± 7.2 |
63.1 ± 8.7 |
63.2 ± 11.6 |
63.6 ± 10.5 |
65.8 ± 9.9 |
Disease duration [years] |
2.4 ± 2.2 |
2.8 ± 2.7 |
2.9 ± 2.5 |
4.0 ± 3.8 |
7.5 ± 5.7 |
9.6 ± 6.5 |
UPDRS-III |
37.3 ± 14.7 |
42.2 ± 10.7 |
47.9 ± 14.6 |
46.8 ± 12 |
35.3 ± 12 |
42.4 ± 11.2 |
BBS |
34.8 ± 16.4 |
28.3 ± 11.9 |
27.8 ± 17.2 |
22.9 ± 12.8 |
42.8 ± 13 |
31.8 ± 14.8 |
MoCA |
20.9 ± 4.7 |
18.9 ± 5.9 |
24.2 ± 4.1 |
24.4 ± 5.5 |
22 ± 5.8 |
17.6 ± 6.5 |
FAB |
12.1 ± 3.4 |
11.9 ± 3.5 |
15.2 ± 3 |
14.8 ± 3.6 |
14.3 ± 3.7 |
11.7 ± 4.2 |
OH [ΔmmHG] |
10.5 ± 12.1 |
13.3 ± 15.1 |
31.9 ± 21.9 |
32.0 ± 21.2 |
17.8 ± 17 |
26.5 ± 21.8 |
*Sub-types for PSP: PSP-RS, PSP-P, and PSP-others; for MSA: MSA-C and MSA-P; for PD: akinetic-rigid, mixed, and tremor-dominant
Discussion
We analysed fall rates of patients with Parkinsonian syndromes based on objectively recorded fall events during hospitalisation as inpatients. While fall rates in PD were predicted by cognitive (MoCA), motor (UPDRS-III, BBS), and an autonomic parameter (OH), falls in PSP were significantly predicted by a motor (UPDRS-III) and an autonomic (OH) parameter. Falls in MSA were significantly predicted by disease duration. Gender or age at diagnosis had no predictive value in any Parkinsonian syndrome.
The occurrence of falls and associated injuries follows different time-courses and is mediated by different risk factors, depending on the syndrome [3]. Our data showed that cognitive, motor, and autonomic factors predict fall rate in PD. This is in line with previous retrospective [3], but also prospective, studies [12]. The latter study has stressed the importance of tandem gait and global cognitive performance (mini-mental state examination) as predictors for short and long-term (6 months and 3.5 years, respectively) fall frequencies. Some studies have also proposed that gender (a higher risk for females) and age at disease onset (a higher risk for older age) contribute to fall frequency [3, 6], but this was not replicated in the current study.
The peak in fall frequency for intermediate disease durations in PSP, with fewer falls in later stages of the disease, is in line with descriptions of a single patient in Brown et al. [10] who suggested that advanced immobilisation and the use of a wheelchair in the later stages of the disease might lead to fewer falls. For PSP patients, falls in our study were predicted by motor and autonomic factors, but not by cognitive status. This finding is in line with previous studies reporting motor factors such as oculomotor parameters, modified turning, bradykinesia, axial rigidity, neck dystonia, and postural stability as being predictive of fall risk [9].
Interestingly, our analysis revealed an association between orthostatic hypotension (OH) and fall risk in PSP patients. However, OH values were lowest for the PSP group (only 40/191, i.e. 20.9% of PSP patients had OH ≥ 20). A recent study has even suggested that there is no association between neurogenic OH and PSP based on data from post mortem-confirmed cases [31]. Thus, even though clinically relevant OH is rare in PSP, it might still contribute to falls as a risk factor, possibly due to mechanisms similar to those in the elderly in general [32]. The role of cognitive status as a risk factor in PSP is under debate. While some studies indicate a role of cognition in falls [3, 11], others point toward deficits of dual-tasking as a risk factor for falls [33]. A recent study with 339 patients did not find a significant association between cognitive parameters and fall risk in PSP [9].
As for MSA, only disease duration proved to be a predictor for falls in this study. While autonomic dysfunction was more severe in MSA than for PD and PSP, the OH was similar for fallers and non-fallers. This is in line with findings reported in a recent review [34] suggesting that dysautonomia does not predict falls, while motor parameters, in particular axial symptoms and early pyramidal tract signs, are associated with falls [3].
However, due to low sample sizes for MSA and PSP, we cannot rule out that in these cases statistical power was insufficient to detect subtle potential effects of cognitive or motor/autonomic parameters on fall rate. In contrast, since PD is comparatively more prevalent in the population, the sample size for PD was also larger in our study, resulting in better statistical power. Thus, for direct comparisons between the Parkinsonian syndromes, sample sizes of the atypical syndromes were possibly insufficient.
Other than that, four further factors might limit the generalisability of this study’s results:
- In this study, fall events were only counted when they occurred during an inpatient stay (of c.14 days). That means that patients with fall rates of less than one fall per two-week period were unlikely to fall during their inpatient stay, and were thus recorded as non-fallers. Therefore, a fall rate of once per six months [12] or two falls per 12 months [11] was likely not to be covered in our study. Thus, the fall rates covered in our study were in the ‘very frequent’ range.
- Recording fall events in a hospital setting has the advantage of the presence of an objective witness, very often a trained member of nursing staff, and is thus not subject to bias due to delayed recall. Nevertheless, the possibility of under-reporting still exists. In particular, patients with high fall rates might not report some actual fall events, because they perceived them to be ‘near-falls’, e.g. when no injury occurred. Moreover, PSP patients very often lack insight into their postural disorder due to cognitive impairment [35] and may therefore also underreport fall events in the absence of witnesses.
- Another factor that might distort fall rate estimates is that during a patient’s stay in a hospital, the environment is very different from that at home and might therefore lack ecological validity (e.g. see Fasano et al. [36]). Specifically, patients might experience more mobilisation and increased activity in the less familiar hospital environment, resulting in an increase of fall frequency. Then again, the hospital might be a more controlled environment with aids such as handrails and wheeled walker, possibly resulting in a lower fall frequency. Furthermore, the presence of professional staff might result in avoiding many near falls from becoming actual fall events.
- Another possible limitation of this study was the reduced variance in disease severity: patients seeking medical help as inpatients are commonly those who are already advanced enough in their disease to suffer significantly, so only a few PD patients in Hoehn & Yahr stages I–II are expected to be treated as inpatients. On the other hand, patients with a severely advanced Parkinsonian syndrome are also rarely present at a specialised Parkinson clinic, where most of the patients are in Hoehn & Yahr stages III–IV.
Interventions to prevent falls in PD have mainly focused on exercise and medication [36]. The targets for exercise-based interventions are mainly poor balance and decreased mobility as risk factors for falls. Interestingly, combining exercise targeted at both physical and cognitive factors using a virtual reality setting has been shown to positively affect the long-term (6-month follow-up) risk of falls [37]. Pharmacological interventions with an effect of reducing fall rates (besides anti-parkinsonian medication) include acetylcholinesterase inhibitors (rivastigmine), but also drugs (droxidopa) ameliorating neurogenic orthostatic hypotension [36].
Due to the retrospective nature of our study, we have not investigated the effect of prescribed medication as a risk factor for falls. However, polypharmacy, sedative, anticholinergic, and other drugs are known to affect the risk of falling for PD patients, and the elderly in general [36, 38]. Other potentially important variables, such as eye disease, sarcopenia or BMI were not investigated either, limiting the scope of the study, considering the effect of obesity on fall frequency demonstrated in previous studies on healthy elderly people [39].
Overall, the described targets to reduce falls in PD are in line with the risk factors predicting fall rate in PD in the current study. Compared to PD, interventions to reduce falls are much less established in PSP, owing to a scarcity of data [10]. Promising approaches have aimed at preventing falls with physical therapy employing treadmill or robot-assisted training [40], or reducing risk by targeting cognition and the impulsiveness typical of PSP [41]. However, the existing data is insufficient to draw valid conclusions as to the efficacy of these approaches. Similarly, therapeutic interventions specifically targeting fall risk reduction in MSA are rare; possibly, some of the strategies developed for PD may prove applicable for MSA as well [34]. Retrospective epidemiological studies can provide rough indications towards plausible targets for interventions.
Nevertheless, to develop effective and individualised therapies for Parkinsonian syndromes, detailed analysis of falls, their mechanisms and possible triggers is necessary. For instance, dual tasking during walking has been suggested as resulting in increased fall probability in PD due to cognitive-motor interaction [42]. Understanding the mechanisms of falling therefore allows for the development of targeted therapies taking into account cognitive, motor, and autonomic factors.
Conclusion
Our study evaluated the rate of falling in a sample of patients with Parkinsonian syndromes. Rather than relying on self-report, we counted falls during a two-week stay as inpatients. We identified different predictors for falls depending on the particular type of syndrome. While for PD, cognitive, motor, and autonomic factors best predicted falls, for MSA the main variable predicting falls was the duration of the disease. For PSP, both motor and autonomic dysfunction predicted falls. Thus, optimal management and prevention of falls should consider these predictors in the different types of Parkinsonian syndrome.
Acknowledgements: We are most grateful to Elmar Trutt for
helpful comments on the manuscript, to Andreas Fleiner
for helpful ideas for the study design and help with data collection, and to Tanja Fiesel, Botond Balo, and Ziad Salhani for help with data collection. Furthermore, we express our gratitude to David Emmans for proofreading this manuscript.
Conflicts of interest: WHJ is an advisor and speaker for Abbvie, Bial, Desitin, Stada, UCB, and Zambon. JK is an advisor and speaker for Desitin. CFA is an advisor for Zambon.
Funding: None.
References
- Kempster PA, O’Sullivan SS, Holton JL, et al. Relationships between age and late progression of Parkinson’s disease: a clinico-pathological study. Brain. 2010; 133(Pt 6): 1755–1762, doi: 10.1093/brain/awq059, indexed in Pubmed: 20371510.
- Wenning G, Ebersbach G, Verny M, et al. Progression of falls in postmortem-confirmed Parkinsonian disorders. Movement Disorders. 2001; 14(6): 947–950, doi: 10.1002/1531-8257(199911)14:6<947::aid-mds1006>3.0.co;2-o.
- Williams DR, Watt HC, Lees AJ. Predictors of falls and fractures in bradykinetic rigid syndromes: a retrospective study. J Neurol Neurosurg Psychiatry. 2006; 77(4): 468–473, doi: 10.1136/jnnp.2005.074070, indexed in Pubmed: 16543524.
- Vossius C, Larsen JP, Janvin C, et al. The economic impact of cognitive impairment in Parkinson’s disease. Mov Disord. 2011; 26(8): 1541––1544, doi: 10.1002/mds.23661, indexed in Pubmed: 21538519.
- Marras C, McDermott MP, Rochon PA, et al. Parkinson Study Group DATATOP Investigators. Predictors of deterioration in health-related quality of life in Parkinson’s disease: results from the DATATOP trial. Mov Disord. 2008; 23(5): 653–9; quiz 776, doi: 10.1002/mds.21853, indexed in Pubmed: 18076084.
- Wielinski CL, Erickson-Davis C, Wichmann R, et al. Falls and injuries resulting from falls among patients with Parkinson’s disease and other parkinsonian syndromes. Mov Disord. 2005; 20(4): 410–415, doi: 10.1002/mds.20347, indexed in Pubmed: 15580552.
- Tinetti ME, Williams CS. Falls, injuries due to falls, and the risk of admission to a nursing home. N Engl J Med. 1997; 337(18): 1279–1284, doi: 10.1056/NEJM199710303371806, indexed in Pubmed: 9345078.
- Glasmacher SA, Leigh PN, Saha RA. Predictors of survival in progressive supranuclear palsy and multiple system atrophy: a systematic review and meta-analysis. J Neurol Neurosurg Psychiatry. 2017; 88(5): 402–411, doi: 10.1136/jnnp-2016-314956, indexed in Pubmed: 28250027.
- Bluett B, Litvan I, Cheng S, et al. ENGENE PSP study. Understanding falls in progressive supranuclear palsy. Parkinsonism Relat Disord. 2017; 35: 75–81, doi: 10.1016/j.parkreldis.2016.12.009, indexed in Pubmed: 28007518.
- Brown FS, Rowe JB, Passamonti L, et al. Falls in Progressive Supranuclear Palsy. Mov Disord Clin Pract. 2020; 7(1): 16–24, doi: 10.1002/mdc3.12879, indexed in Pubmed: 31970205.
- Kim SL, Lee MJ, Lee MS. Cognitive dysfunction associated with falls in progressive supranuclear palsy. Gait Posture. 2014; 40(4): 605–609, doi: 10.1016/j.gaitpost.2014.07.005, indexed in Pubmed: 25088758.
- Lindholm B, Brogårdh C, Odin P, et al. Longitudinal prediction of falls and near falls frequencies in Parkinson’s disease: a prospective cohort study. J Neurol. 2021; 268(3): 997–1005, doi: 10.1007/s00415-020-10234-6, indexed in Pubmed: 32970193.
- Litvan I, Agid Y, Calne D, et al. Clinical research criteria for the diagnosis of progressive supranuclear palsy (Steele-Richardson-Olszewski syndrome): report of the NINDS-SPSP international workshop. Neurology. 1996; 47(1): 1–9, doi: 10.1212/wnl.47.1.1, indexed in Pubmed: 8710059.
- Höglinger GU, Respondek G, Stamelou M, et al. Movement Disorder Society-endorsed PSP Study Group. Clinical diagnosis of progressive supranuclear palsy: The movement disorder society criteria. Mov Disord. 2017; 32(6): 853–864, doi: 10.1002/mds.26987, indexed in Pubmed: 28467028.
- Gilman S, Wenning GK, Low PA, et al. Second consensus statement on the diagnosis of multiple system atrophy. Neurol. 2008; 71: 670–676, doi: 10.1212/wnl.0000324625.00404.15.
- Gibb WR, Lees AJ. The relevance of the Lewy body to the pathogenesis of idiopathic Parkinson’s disease. J Neurol Neurosurg Psychiatry. 1988; 51(6): 745–752, doi: 10.1136/jnnp.51.6.745, indexed in Pubmed: 2841426.
- Postuma RB, Berg D, Stern M, et al. MDS clinical diagnostic criteria for Parkinson’s disease. Mov Disord. 2015; 30(12): 1591–1601, doi: 10.1002/mds.26424, indexed in Pubmed: 26474316.
- Kang GA, Bronstein JM, Masterman DL, et al. Clinical characteristics in early Parkinson’s disease in a central California population-based study. Mov Disord. 2005; 20(9): 1133–1142, doi: 10.1002/mds.20513, indexed in Pubmed: 15954133.
- Altmann CF, Trubelja K, Emmans D, et al. Time-course of decline in different cognitive domains in Parkinson’s disease: a retrospective study. J Neural Transm (Vienna). 2022; 129(9): 1179–1187, doi: 10.1007/s00702-021-02441-w, indexed in Pubmed: 34817687.
- Fahn S, Elton RL. members of the UPDRS Development Committee. Unified Parkinson`s disease rating scale. In: Fahn SL, Marsden CD, Goldstein M, Calne DB. ed. Recent deve¬lopments in Parkinson’s disease. Macmillan, New York 1987: 153–163.
- Berg K. Measuring balance in the elderly: preliminary development of an instrument. Physiotherapy Canada. 1989; 41(6): 304–311, doi: 10.3138/ptc.41.6.304.
- Scherfer E, Bohls C, Freiberger E, et al. Berg-Balance-Scale - deutsche Version. Physioscience. 2006; 2(2): 59–66, doi: 10.1055/s-2006-926833.
- Nasreddine ZS, Phillips NA, Bédirian V, et al. The Montreal Cognitive Assessment, MoCA: a brief screening tool for mild cognitive impairment. J Am Geriatr Soc. 2005; 53(4): 695–699, doi: 10.1111/j.1532-5415.2005.53221.x, indexed in Pubmed: 15817019.
- Dalrymple-Alford JC, MacAskill MR, Nakas CT, et al. The MoCA: well-suited screen for cognitive impairment in Parkinson disease. Neurology. 2010; 75(19): 1717–1725, doi: 10.1212/WNL.0b013e3181fc29c9, indexed in Pubmed: 21060094.
- Dubois B, Slachevsky A, Litvan I, et al. The FAB: a Frontal Assessment Battery at bedside. Neurology. 2000; 55(11): 1621–1626, doi: 10.1212/wnl.55.11.1621, indexed in Pubmed: 11113214.
- Fanciulli A, Campese N, Wenning GK. The Schellong test: detecting orthostatic blood pressure and heart rate changes in German-speaking countries. Clin Auton Res. 2019; 29(4): 363–366, doi: 10.1007/s10286-019-00619-7, indexed in Pubmed: 31273549.
- Lamb SE, Jørstad-Stein EC, Hauer K, et al. Prevention of Falls Network Europe and Outcomes Consensus Group. Development of a common outcome data set for fall injury prevention trials: the Prevention of Falls Network Europe consensus. J Am Geriatr Soc. 2005; 53(9): 1618–1622, doi: 10.1111/j.1532-5415.2005.53455.x, indexed in Pubmed: 16137297.
- Field A, Miles J, Field Z. Discovering Statistics using R. Sage Publications, London 2012.
- Ver Hoef JM, Boveng PL. Quasi-Poisson vs. negative binomial regression: how should we model overdispersed count data? Ecology. 2007; 88(11): 2766–2772, doi: 10.1890/07-0043.1, indexed in Pubmed: 18051645.
- McKay JL, Hackney ME, Factor SA, et al. Lower Limb Rigidity Is Associated with Frequent Falls in Parkinson’s Disease. Mov Disord Clin Pract. 2019; 6(6): 446–451, doi: 10.1002/mdc3.12784, indexed in Pubmed: 31392245.
- van Gerpen JA, Al-Shaikh RH, Tipton PW, et al. Progressive supranuclear palsy is not associated with neurogenic orthostatic hypotension. Neurology. 2019; 93(14): e1339–e1347, doi: 10.1212/WNL.0000000000008197, indexed in Pubmed: 31484717.
- Ooi WL, Hossain M, Lipsitz LA. The association between orthostatic hypotension and recurrent falls in nursing home residents. Am J Med. 2000; 108(2): 106–111, doi: 10.1016/s0002-9343(99)00425-8, indexed in Pubmed: 11126303.
- Lindemann U, Nicolai S, Beische D, et al. Clinical and dual-tasking aspects in frequent and infrequent fallers with progressive supranuclear palsy. Mov Disord. 2010; 25(8): 1040–1046, doi: 10.1002/mds.23023, indexed in Pubmed: 20131396.
- Joza S, Camicioli R, Ba F. Falls in Synucleinopathies. Can J Neurol Sci. 2020; 47(1): 30–43, doi: 10.1017/cjn.2019.287, indexed in Pubmed: 31452470.
- Bluett B, Pantelyat AY, Litvan I, et al. Best Practices in the Clinical Management of Progressive Supranuclear Palsy and Corticobasal Syndrome: A Consensus Statement of the CurePSP Centers of Care. Front Neurol. 2021; 12: 694872, doi: 10.3389/fneur.2021.694872, indexed in Pubmed: 34276544.
- Fasano A, Canning CG, Hausdorff JM, et al. Falls in Parkinson’s disease: A complex and evolving picture. Mov Disord. 2017; 32(11): 1524–
–1536, doi: 10.1002/mds.27195, indexed in Pubmed: 29067726. - Mirelman A, Rochester L, Maidan I, et al. Addition of a non-immersive virtual reality component to treadmill training to reduce fall risk in older adults (V-TIME): a randomised controlled trial. Lancet. 2016; 388(10050): 1170–1182, doi: 10.1016/S0140-6736(16)31325-3, indexed in Pubmed: 27524393.
- Dhalwani NN, Fahami R, Sathanapally H, et al. Association between polypharmacy and falls in older adults: a longitudinal study from England. BMJ Open. 2017; 7(10): e016358, doi: 10.1136/bmjopen-2017-016358, indexed in Pubmed: 29042378.
- Mitchell RJ, Lord SR, Harvey LA, et al. Associations between obesity and overweight and fall risk, health status and quality of life in older people. Aust N Z J Public Health. 2014; 38(1): 13–18, doi: 10.1111/1753-6405.12152, indexed in Pubmed: 24494939.
- Clerici I, Ferrazzoli D, Maestri R, et al. Rehabilitation in progressive supranuclear palsy: Effectiveness of two multidisciplinary treatments. PLoS One. 2017; 12(2): e0170927, doi: 10.1371/journal.pone.0170927, indexed in Pubmed: 28158197.
- Rittman T, Coyle-Gilchrist ITs, Rowe JB. Managing cognition in progressive supranuclear palsy. Neurodegener Dis Manag. 2016; 6(6): 499–508, doi: 10.2217/nmt-2016-0027, indexed in Pubmed: 27879155.
- Raffegeau TE, Krehbiel LM, Kang N, et al. A meta-analysis: Parkinson’s disease and dual-task walking. Parkinsonism Relat Disord. 2019; 62: 28–35, doi: 10.1016/j.parkreldis.2018.12.012, indexed in Pubmed: 30594454.
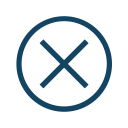