Symbol Digit Modalities Test in progressive multiple sclerosis
Abstract
Introduction. The Symbol Digit Modalities Test (SDMT) is a highly sensitive neuropsychological tool used for the assessment of information processing speed (IPS) in various neurological disorders.
State of the art. In this review, we have focused on the current knowledge regarding the use of SDMT selectively in the evaluation of progressive multiple sclerosis (PMS) patients. A literature review was performed regarding the application of SDMT in PMS, with a focus on the primary progressive and secondary progressive subtypes. Relationships of diverse disease-associated factors with SDMT have been described, including disease course, imaging findings, molecular biomarkers, treatment and others.
Clinical implications. SDMT is a very useful and easily applicable instrument in the diagnostic armamentarium of neurologists and neuropsychologists. It is especially valuable in the evaluation of PMS patients, in whom the prevalence of IPS deficits is higher than in relapsing-remitting multiple sclerosis subjects or in healthy individuals.
Future directions. An emphasis should be laid on larger study groups and differentiating between individual PMS subtypes and their separate analysis in the context of cognitive assessment.
Keywords: Symbol Digit Modalities TestSDMTprogressive multiple sclerosisinformation processing speedcognitive dysfunction
References
- Baecher-Allan C, Kaskow BJ, Weiner HL. Multiple sclerosis: mechanisms and immunotherapy. Neuron. 2018; 97(4): 742–768.
- Koch-Henriksen N, Sørensen PS. The changing demographic pattern of multiple sclerosis epidemiology. Lancet Neurol. 2010; 9(5): 520–532.
- Lublin FD, Reingold SC, Cohen JA, et al. Defining the clinical course of multiple sclerosis: the 2013 revisions. Neurology. 2014; 83(3): 278–286.
- Ontaneda D, Fox RJ. Progressive multiple sclerosis. Curr Opin Neurol. 2015; 28(3): 237–243.
- Khurana V, Sharma H, Medin J. Estimated prevalence of secondary progressive multiple sclerosis in the USA and Europe: results from a systematic literature search (P2.380). Neurology. 2018; 90(15_supplement).
- Fambiatos A, Jokubaitis V, Horakova D, et al. Risk of secondary progressive multiple sclerosis: a longitudinal study. Mult Scler. 2020; 26(1): 79–90.
- Rice GPA. The natural history of secondary progressive multiple sclerosis: observations from the London study group. Mult Scler. 2002; 8(1): 81–82.
- Cree BAC, Gourraud PA, Oksenberg JR, et al. University of California, San Francisco MS-EPIC Team:. Long-term evolution of multiple sclerosis disability in the treatment era. Ann Neurol. 2016; 80(4): 499–510.
- Diker S, Has AC, Kurne A, et al. The association of cognitive impairment with gray matter atrophy and cortical lesion load in clinically isolated syndrome. Mult Scler Relat Disord. 2016; 10: 14–21.
- Johnen A, Landmeyer NC, Bürkner PC, et al. Distinct cognitive impairments in different disease courses of multiple sclerosis: a systematic review and meta-analysis. Neurosci Biobehav Rev. 2017; 83: 568–578.
- Jongen PJ, Ter Horst AT, Brands AM. Cognitive impairment in multiple sclerosis. Minerva Med. 2012; 103(2): 73–96.
- Pitteri M, Romualdi C, Magliozzi R, et al. Cognitive impairment predicts disability progression and cortical thinning in MS: An 8-year study. Mult Scler. 2017; 23(6): 848–854.
- Beatty WW, Goodkin DE, Monson N, et al. Anterograde and retrograde amnesia in patients with chronic progressive multiple sclerosis. Arch Neurol. 1988; 45(6): 611–619.
- Baumstarck-Barrau K, Simeoni MC, Reuter F, et al. Cognitive function and quality of life in multiple sclerosis patients: a cross-sectional study. BMC Neurol. 2011; 11: 17.
- Oset M, Stasiolek M, Matysiak M. Cognitive dysfunction in the early stages of multiple sclerosis-how much and how important? Curr Neurol Neurosci Rep. 2020; 20(7): 22.
- Kizlaitienė R, Kaubrys G, Giedraitienė N, et al. Composite marker of cognitive dysfunction and brain atrophy is highly accurate in discriminating between relapsing-remitting and secondary progressive multiple sclerosis. Med Sci Monit. 2017; 23: 588–597.
- Connick P, Chandran S, Bak TH. Patterns of cognitive dysfunction in progressive MS. Behav Neurol. 2013; 27(3): 259–265.
- Ruet A, Deloire M, Charré-Morin J, et al. Cognitive impairment differs between primary progressive and relapsing-remitting MS. Neurology. 2013; 80(16): 1501–1508.
- Planche V, Gibelin M, Cregut D, et al. Cognitive impairment in a population-based study of patients with multiple sclerosis: differences between late relapsing-remitting, secondary progressive and primary progressive multiple sclerosis. Eur J Neurol. 2016; 23(2): 282–289.
- Riccitelli G, Rocca MA, Pagani E, et al. Cognitive impairment in multiple sclerosis is associated to different patterns of gray matter atrophy according to clinical phenotype. Hum Brain Mapp. 2011; 32(10): 1535–1543.
- Giovannoni G, Hawkes CH, Lechner-Scott J, et al. Multiple sclerosis is one disease. Mult Scler Relat Disord. 2022; 63.
- Ruano L, Portaccio E, Goretti B, et al. Age and disability drive cognitive impairment in multiple sclerosis across disease subtypes. Mult Scler. 2017; 23(9): 1258–1267.
- Sandry J, Simonet DV, Brandstadter R, et al. The Symbol Digit Modalities Test (SDMT) is sensitive but non-specific in MS: lexical access speed, memory, and information processing speed independently contribute to SDMT performance. Mult Scler Relat Disord. 2021; 51.
- (SDMT) Symbol Digit Modalities Test. https://www.wpspublish.com/sdmt-symbol-digit-modalities-test (01.02.2022).
- Kalb R, Beier M, Benedict RHb, et al. Recommendations for cognitive screening and management in multiple sclerosis care. Mult Scler. 2018; 24(13): 1665–1680.
- Betscher E, Guenter W, Langdon DW, et al. Polish validation of the Brief International Cognitive Assessment for Multiple Sclerosis (BICAMS battery): correlation of cognitive impairment with mood disorders and fatigue. Neurol Neurochir Pol. 2021; 55(1): 59–66.
- Johnen A, Landmeyer NC, Bürkner PC, et al. Distinct cognitive impairments in different disease courses of multiple sclerosis: a systematic review and meta-analysis. Neurosci Biobehav Rev. 2017; 83: 568–578.
- Podda J, Ponzio M, Pedullà L, et al. Predominant cognitive phenotypes in multiple sclerosis: Insights from patient-centered outcomes. Mult Scler Relat Disord. 2021; 51: 102919.
- Rodrigues DN, Paes RA, Vasconcelos CC, et al. Different cognitive profiles of Brazilian patients with relapsing-remitting and primary progressive multiple sclerosis. Arq Neuropsiquiatr. 2011; 69(4): 590–595.
- Khan A, Li Yi, Ponirakis G, et al. Corneal immune cells are increased in patients with multiple sclerosis. Transl Vis Sci Technol. 2021; 10(4).
- Prosperini L, Castelli L, Sellitto G, et al. Investigating the phenomenon of "cognitive-motor interference" in multiple sclerosis by means of dual-task posturography. Gait Posture. 2015; 41(3): 780–785.
- Wen J, Yablonskiy DA, Luo J, et al. Detection and quantification of regional cortical gray matter damage in multiple sclerosis utilizing gradient echo MRI. Neuroimage Clin. 2015; 9: 164–175.
- Huijbregts SCJ, Kalkers NF, de Sonneville LMJ, et al. Differences in cognitive impairment of relapsing remitting, secondary, and primary progressive MS. Neurology. 2004; 63(2): 335–339.
- Charalambous T, Tur C, Prados F, et al. Structural network disruption markers explain disability in multiple sclerosis. J Neurol Neurosurg Psychiatry. 2019; 90(2): 219–226.
- Ruet A, Deloire MSA, Charré-Morin J, et al. A new computerised cognitive test for the detection of information processing speed impairment in multiple sclerosis. Mult Scler. 2013; 19(12): 1665–1672.
- Lapshin H, Audet B, Feinstein A. Detecting cognitive dysfunction in a busy multiple sclerosis clinical setting: a computer generated approach. Eur J Neurol. 2014; 21(2): 281–286.
- Baumhefner RW, Tourtellotte WW, Syndulko K, et al. Quantitative multiple sclerosis plaque assessment with magnetic resonance imaging. Its correlation with clinical parameters, evoked potentials, and intra-blood-brain barrier IgG synthesis. Arch Neurol. 1990; 47(1): 19–26.
- Pérez-Miralles FC, Prefasi D, García-Merino A, et al. Brain region volumes and their relationship with disability progression and cognitive function in primary progressive multiple sclerosis. Brain Behav. 2021; 11(4): e02044.
- Colato E, Stutters J, Tur C, et al. Predicting disability progression and cognitive worsening in multiple sclerosis using patterns of grey matter volumes. J Neurol Neurosurg Psychiatry. 2021; 92(9): 995–1006.
- Koch MW, Mostert J, Repovic P, et al. MRI brain volume loss, lesion burden, and clinical outcome in secondary progressive multiple sclerosis. Mult Scler. 2022; 28(4): 561–572.
- Dineen RA, Vilisaar J, Hlinka J, et al. Disconnection as a mechanism for cognitive dysfunction in multiple sclerosis. Brain. 2009; 132(Pt 1): 239–249.
- Cocozza S, Petracca M, Mormina E, et al. Cerebellar lobule atrophy and disability in progressive MS. J Neurol Neurosurg Psychiatry. 2017; 88(12): 1065–1072.
- Raji A, Ostwaldt AC, Opfer R, et al. MRI-Based brain volumetry at a single time point complements clinical evaluation of patients with multiple sclerosis in an outpatient setting. Front Neurol. 2018; 9: 545.
- Hänninen K, Viitala M, Paavilainen T, et al. Thalamic atrophy without whole brain atrophy is associated with absence of 2-year NEDA in multiple sclerosis. Front Neurol. 2019; 10: 459.
- Tavazzi E, Bergsland N, Kuhle J, et al. A multimodal approach to assess the validity of atrophied T2-lesion volume as an MRI marker of disease progression in multiple sclerosis. J Neurol. 2020; 267(3): 802–811.
- Francis PL, Jakubovic R, O'Connor P, et al. Robust perfusion deficits in cognitively impaired patients with secondary-progressive multiple sclerosis. AJNR Am J Neuroradiol. 2013; 34(1): 62–67.
- Testud B, Delacour C, El Ahmadi AA, et al. Brain grey matter perfusion in primary progressive multiple sclerosis: mild decrease over years and regional associations with cognition and hand function. Eur J Neurol. 2022; 29(6): 1741–1752.
- Eilaghi A, Kassner A, Sitartchouk I, et al. Normal-appearing white matter permeability distinguishes poor cognitive performance in processing speed and working memory. AJNR Am J Neuroradiol. 2013; 34(11): 2119–2124.
- Solanky BS, John NA, DeAngelis F, et al. MS-SMART Investigators. NAA is a marker of disability in secondary-progressive MS: a proton MR spectroscopic imaging study. AJNR Am J Neuroradiol. 2020; 41(12): 2209–2218.
- Biernacki T, Kokas Z, Sandi D, et al. Emerging biomarkers of multiple sclerosis in the blood and the CSF: a focus on neurofilaments and therapeutic considerations. Int J Mol Sci. 2022; 23(6).
- Lincoln MR, Montpetit A, Cader MZ, et al. A predominant role for the HLA class II region in the association of the MHC region with multiple sclerosis. Nat Genet. 2005; 37(10): 1108–1112.
- Van der Walt A, Stankovich J, Bahlo M, et al. Apolipoprotein genotype does not influence MS severity, cognition, or brain atrophy. Neurology. 2009; 73(13): 1018–1025.
- Abdelhak A, Huss A, Stahmann A, et al. Explorative study of emerging blood biomarkers in progressive multiple sclerosis (EmBioProMS): Design of a prospective observational multicentre pilot study. Contemp Clin Trials Commun. 2020; 18.
- Siddiqui K, Browne RW, Benedict RHB, et al. Cholesterol pathway biomarkers are associated with neuropsychological measures in multiple sclerosis. Mult Scler Relat Disord. 2023; 69.
- Jakimovski D, Benedict RHB, Weinstock-Guttman B, et al. Visual deficits and cognitive assessment of multiple sclerosis: confounder, correlate, or both? J Neurol. 2021; 268(7): 2578–2588.
- Britze J, Frederiksen JL. Optical coherence tomography in multiple sclerosis. Eye (Lond). 2018; 32(5): 884–888.
- Nguyen J, Rothman A, Fitzgerald K, et al. Visual pathway measures are associated with neuropsychological function in multiple sclerosis. Curr Eye Res. 2018; 43(7): 941–948.
- Højsgaard Chow H, Schreiber K, Magyari M, et al. Progressive multiple sclerosis, cognitive function, and quality of life. Brain Behav. 2018; 8(2): e00875.
- W Koch M, Mostert J, Repovic P, et al. Impact of clinical outcomes and imaging measures on health-related quality of life in secondary progressive MS. Mult Scler. 2022; 28(8): 1286–1298.
- Renner A, Baetge SJ, Filser M, et al. Working ability in individuals with different disease courses of multiple sclerosis: factors beyond physical impairment. Mult Scler Relat Disord. 2020; 46.
- Andreasen AK, Iversen P, Marstrand L, et al. Structural and cognitive correlates of fatigue in progressive multiple sclerosis. Neurol Res. 2019; 41(2): 168–176.
- Gil-Perotin S, Bernad L, Reddam S, et al. Patient's perspective in clinical practice to assess and predict disability in multiple sclerosis. Sci Rep. 2022; 12(1): 18238.
- Prakash RS, Snook EM, Motl RW, et al. Aerobic fitness is associated with gray matter volume and white matter integrity in multiple sclerosis. Brain Res. 2010; 1341: 41–51.
- Sandroff BM, Motl RW, Amato MP, et al. Cardiorespiratory fitness and free-living physical activity are not associated with cognition in persons with progressive multiple sclerosis: Baseline analyses from the CogEx study. Mult Scler. 2022; 28(7): 1091–1100.
- Bombardier CH, Motl RW, Benedict RHB, et al. Exercise training and cognition in multiple sclerosis: the GET smart trial protocol. Contemp Clin Trials. 2021; 104.
- Feinstein A, Amato MP, Brichetto G, et al. CogEx Research Team. Study protocol: improving cognition in people with progressive multiple sclerosis: a multi-arm, randomized, blinded, sham-controlled trial of cognitive rehabilitation and aerobic exercise (COGEx). BMC Neurol. 2020; 20(1): 204.
- Feinstein A, Amato MP, Brichetto G, et al. CogEx Research Team. Cognitive rehabilitation and aerobic exercise for cognitive impairment in people with progressive multiple sclerosis (CogEx): a randomised, blinded, sham-controlled trial. Lancet Neurol. 2023; 22(10): 912–924.
- Chow HH, Talbot J, Marstrand L, et al. Smoking, cardiovascular risk factors and LRP2 gene variation: associations with disease severity, cognitive function and brain structure in primary progressive multiple sclerosis. Mult Scler Relat Disord. 2021; 56.
- Carotenuto A, Costabile T, Moccia M, et al. Olfactory function and cognition in relapsing-remitting and secondary-progressive multiple sclerosis. Mult Scler Relat Disord. 2019; 27: 1–6.
- Mortensen GL, Theódórsdóttir Á, Sejbæk T, et al. Patient attitudes to routine cognitive testing in multiple sclerosis. Patient Prefer Adherence. 2020; 14: 693–704.
- Benedict RHB, Tomic D, Cree BA, et al. Siponimod and cognition in secondary progressive multiple sclerosis: EXPAND secondary analyses. Neurology. 2021; 96(3): e376–e386.
- Koch MW, Mostert J, Repovic P, et al. Is the Symbol Digit Modalities Test a useful outcome in secondary progressive multiple sclerosis? Eur J Neurol. 2021; 28(6): 2115–2120.
- Ziemssen T, Hoffmann O, Klotz L, et al. Gaining first insights on secondary progressive multiple sclerosis patients treated with siponimod in clinical routine: protocol of the noninterventional study AMASIA. JMIR Res Protoc. 2020; 9(7): e19598.
- Højsgaard Chow H, Talbot J, Lundell H, et al. Dimethyl fumarate treatment of primary progressive multiple sclerosis: results of an open-label extension study. Mult Scler Relat Disord. 2023; 70: 104458.
- A multicentre, open label, single-arm, phase 3b study (CONSONANCE) to assess the effectiveness and safety of ocrelizumab in patients with primary and secondary progressive multiple sclerosis: Year 2 interim analysis - ECTRIMS-2022-poster-comi-a-multicentre-open-label-single-arm-phase-3b-study. https://medically.gene.com/global/en/unrestricted/neuroscience/ECTRIMS-2022/ectrims-2022-poster-comi-a-multicentre-open-label-singl.html (28.12.2023).
- A Study to Evaluate Ocrelizumab Treatment in Participants With Progressive Multiple Sclerosis. https://clinicaltrials.gov/study/NCT03523858#publications (28.12.203).
- Boziki M, Bakirtzis C, Sintila SA, et al. Ocrelizumab in patients with active primary progressive multiple sclerosis: clinical outcomes and immune markers of treatment response. Cells. 2022; 11(12).
- Langdon DW, Amato MP, Boringa J, et al. Recommendations for a brief international cognitive assessment for multiple sclerosis (BICAMS). Mult Scler. 2012; 18(6): 891–898.
- Signori A, Lorscheider J, Vukusic S, et al. Heterogeneity on long-term disability trajectories in patients with secondary progressive MS: a latent class analysis from Big MS Data network. Journal of Neurology, Neurosurgery & Psychiatry. 2022; 94(1): 23–30.
- Planche V, Gibelin M, Cregut D, et al. Cognitive impairment in a population-based study of patients with multiple sclerosis: differences between late relapsing-remitting, secondary progressive and primary progressive multiple sclerosis. Eur J Neurol. 2016; 23(2): 282–289.
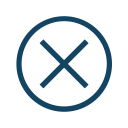