Jak sprawić by sztuczna inteligencja stała się etycznym narzędziem diagnostycznym w praktyce lekarskiej?
Streszczenie
Zastosowanie rozwiązań ze sfery sztucznej inteligencji może przyczynić się do poprawy jakości świadczeń w sektorze ochrony zdrowia. Algorytmy AI pozwalają na szybsze przetwarzanie i analizowanie danych, a co za tym idzie sprawniejszy proces diagnozowania pacjentów. Z drugiej strony rozwiązania AI nadal nie są pozbawione błędów. Ich algorytmy obarczone są uprzedzeniami, które mogą wykluczać grupy mniej uprzywilejowane w społeczeństwie. Uprzedzenia w uczeniu maszynowym, zwane także uprzedzeniami algorytmów lub uprzedzeniami AI (AI bias), to zjawisko występujące wtedy, gdy algorytm generuje wyniki, które są systematycznie uprzedzone ze względu na błędne założenia już w procesie uczenia maszynowego. Jest to sytuacja, w której prawidłowy algorytm wyklucza pewne dane lub grupę danych. Celem niniejszego artykułu jest zarysowanie problematyki etycznego zastosowania sztucznej inteligencji w sektorze medycznym ze szczególnym uwzględnieniem uprzedzeń sztucznej inteligencji. W medycynie jest to o tyle istotny problem, iż przekłada się on na jakość opieki nad pacjentami oraz tego w jaki sposób rozkładać się będą ich szanse na powrót do zdrowia. W poniższym artykule poruszone zostały zagadnienia prawne oraz sposoby klasyfikacji sztucznej inteligencji przez Unię Europejską, kwestie uprzedzenia sztucznej inteligencji oraz wiążących się z tym zagrożeń wraz z dotychczasowymi przykładami prób wdrażania sztucznej inteligencji w sektorze medycznym oraz perspektywy dla zastosowania sztucznej inteligencji w sektorze medycznym ze szczególnym naciskiem na kardiologię. Naszym wnioskiem jest, iż prace nad sztuczną inteligencją powinny być kontynuowane, a algorytmy udoskonalane. Pomimo swoich wad, jest to nadal niezwykle pomocne narzędzie diagnostyczne, które powinno być powszechnie wprowadzane do codziennej praktyki lekarzy. Niniejsza praca napisana została z zastosowaniem metody analizy i krytyki piśmiennictwa, aktów prawa krajowego i Unii Europejskiej oraz przeglądu dotychczasowych badań dotyczących zastosowania sztucznej inteligencji w medycynie.
Słowa kluczowe: Sztuczna inteligencjaSIuprzedzenie SIkardiologiauczenie maszynoweetyka
Posiadasz dostęp do tego artykułu?
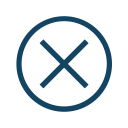