PRACE ORYGINALNE/ORIGINAL PAPERS

Unsupervised analysis of follicular thyroid tumours transcriptome by oligonucleotide microarray gene expression profiling
Analiza nienadzorowana transkryptomu nowotworów pęcherzykowych tarczycy za pomocą profilowania ekspresji genów metodą mikromacierzy oligonukleotydowych
1Department of Nuclear Medicine and Endocrine Oncology, Maria Skłodowska-Curie Memorial Cancer Centre and Institute of Oncology, Gliwice Branch, Poland
2Department of Radiotherapy and Chemotherapy, Maria Skłodowska-Curie Memorial Cancer Centre and Institute of Oncology, Gliwice Branch, Poland
3Department of Oncologic Surgery, Maria Skłodowska-Curie Memorial Cancer Centre and Institute of Oncology, Gliwice Branch, Poland
4Department of Tumour Pathology, Maria Skłodowska-Curie Memorial Cancer Centre and Institute of Oncology, Gliwice Branch, Poland
5Faculty Of Automatic Control, Electronics And Computer Science, Silesian University of Technology, Gliwice, Poland
6Division of Endocrinology and Nephrology, University of Leipzig, Germany
*All three authors (BW, AP, MJ) contributed equally

Barbara Jarząb, M. D., Ph. D. Department of Nuclear Medicine and Endocrine Oncology, Maria Skłodowska-Curie Memorial Cancer Centre and Institute of Oncology, Gliwice Branch, Wybrzeże Armii Krajowej 15, 44–101 Gliwice, Poland, tel.: +48 32 278 9339, fax: +48 32 278 9310, e-mail bjarzab@io.gliwice.pl
Abstract
Introduction: Mechanisms driving the invasiveness of follicular thyroid cancer (FTC) are not fully understood. In our study, we undertook an unsupervised analysis of the set of follicular thyroid tumours (adenomas (FTA) and carcinomas) to verify whether the malignant phenotype influences major sources of variability in our dataset.
Material and methods: The core set of samples consisted of 52 tumours (27 FTC, 25 FTA). Total RNA was analysed by oligonucleotide microarray (HG-U133 Plus 2.0). Principal Component Analysis (PCA) was applied as a main method of unsupervised analysis.
Results: An analysis of biological character of genes correlated to the first six PCs was performed. When genes correlated to the first PC were used to cluster FTC and FTA, they appeared in two branches; one, relatively enriched in adenomas, with homogenous expression of subset of genes, and the other containing mainly carcinomas, with down-regulation of these genes and heterogeneous up-regulation in a smaller cluster of transcripts. Genes highly up-regulated in adenomas included some thyroid-specific transcripts. The second cluster of genes, up-regulated in carcinomas, contained mainly immunity-related transcripts. Immune response genes were found in the first, third and sixth principal components, improving the discrimination between carcinomas and adenomas.
Conclusions: Our unsupervised analysis indicates that invasiveness of follicular tumours might be considered as the major source of variability in transcriptome analysis. However, the distance between both groups is small and the clusters are overlapping, thus, unsupervised analysis is not sufficient to properly classify them.
(Endokrynol Pol 2013; 64 (5): 328–334)
Key words: follicular thyroid carcinoma, follicular adenoma, gene expression
Streszczenie
Wstęp: Rak pęcherzykowy tarczycy (FTC) jest nowotworem którego podłoże molekularne jest mało zbadane. W podjętej analizie transkryptomu oceniono możliwość dyskryminacji raka i gruczolaka pęcherzykowego tarczycy (FTA) na podstawie badań profilu ekspresji genów metodą tzw. nienadzorowaną (tzn. na podstawie dominujących źródeł zmienności). Analizę tę prowadzono by sprawdzić czy złośliwość guza jest rzeczywiście czynnikiem dominującym dla profilu ekspresji genów w nowotworach pęcherzykowych.
Materiał i metody: Podstawowy zbiór guzów pęcherzykowych obejmował 52 próbki (27 FTC i 25 FTA), z których wyizolowano całkowity RNA i poddano badaniu na mikromacierzach HG-U133 Plus 2.0. Otrzymany zbiór normalizowano za pomocą RMA i GC-RMA. Identyfikacji głównych źródeł zmienności dokonano metodą analizy głównych składowych (PCA).
Wyniki: Analizę funkcji biologicznej genów przeprowadzono dla pierwszych 6 składowych głównych. Geny skorelowane z pierwszą składową pozwalały wyodrębnić 2 klastry próbek: jeden złożony głównie z gruczolaków, z wysoką ekspresją między innymi transkryptów tarczycowo-swoistych, drugi zaś, zawierający większość raków, wykazywał zwiększoną, ale heterogenną ekspresję genów związanych z odpowiedzią immunologiczną, a obniżoną ekspresję genów tarczycowych. Geny odpowiedzi immunologicznej stwierdzono wśród transkryptów skorelowanych przebiegiem pierwszej, trzeciej i szóstej głównej składowej; w istotny sposób wpływały one na rozróżnienie między FTC i FTA.
Wnioski: W analizie nienadzorowanej stwierdzono, że złośliwość (inwazyjność) nowotworu pęcherzykowego może być jednym z głównych źródeł zmienności w transkryptomie tych guzów. Jednak, genomiczna odległość między grupami FTC i FTA jest niewielka, a wyodrębnione w analizie nienadzorowanej klastry nakładają się, stąd sama analiza nienadzorowana nie jest wystarczającym narzędziem do celów klasyfikacji tych guzów.
(Endokrynol Pol 2013; 64 (5): 329–334)
Słowa kluczowe: rak pęcherzykowy tarczycy, gruczolak pęcherzykowy, ekspresja genów
This work was supported by the Ministry of Science and Higher Education grants nr N N401 072637, N N403 194340 and Foundation for Polish Science MPD Programme ‘Molecular Genomics, Transcriptomics and Bioinformatics in Cancer’ and Postgraduate School of Molecular Medicine (BW and TS). An author of this publication (AP) is a scholar in the SWIFT project POKL.08.02.01-24-005/10 which is cofinanced by the European Union within the European Social Fund.
Introduction
Follicular thyroid cancer is a malignant tumour believed to arise from follicular cells in the thyroid gland. Being relatively rare compared to the papillary histotype of thyroid carcinoma, follicular cancer has not yet been thoroughly investigated in regard to the molecular mechanisms driving the malignant potential [1–4].
It is of special interest, because follicular thyroid carcinoma has a benign counterpart follicular thyroid adenoma. What is relatively unique, although resulting from the high degree of differentiation in this type of cancer and occurring also in other endocrine malignancies, is that there are no cell-specific criteria differentiating the areas with malignant potential from the fully benign tumours [5, 6]. The only defining feature is the presence of invasion of tumour capsule and vessels, if it is prominent enough to be spotted by a pathologist [7, 8]. The degree of invasion defines the subtypes of disease (minimally invasive, classical, and widely invasive) and certainly influences the reliability of the assessment by a pathologist. It is possible that some thyroid adenomas are in fact follicular carcinomas with no invasion revealed by pathology examination [4].
The difficulties described above give rise to the question whether the ability of follicular cells to invade is an inherent part of their genomic programme. If so, detection of that profile in cells could lead to genomic tests which on the basis of gene expression (and possibly in future also gene mutations and other aberrations) provide the information whether the tumour is benign or malignant not relying on the presence or absence of apparent invasion areas [9]. Unfortunately, comparisons on the global scale, i.e. between the full transcriptomes, almost always provides certain differences and their validation requires large independent samples [4, 9]. However, when the genomic distance between groups is large, the positive validation in further prospective studies is much easier and often leads to clinically significant conclusions.
One method to verify genomic distance between classes of samples is so called unsupervised analysis. In our bioinformatic study, we discriminate specimens on the basis of differences which are the major source of variability in the analysed dataset and are evident without previous assumptions regarding the sample class membership. In our study, we undertake an unsupervised analysis of a set of follicular thyroid tumours (both adenomas and carcinomas) to verify whether tumour malignancy is the factor influencing major sources of variability in our dataset.
Material and methods
We analysed a dataset consisting of 82 samples, among them thyroid carcinomas (FTC), thyroid adenomas (FTA) and grossly normal thyroid tissue (N) from the lobe of thyroid opposite to the tumours. Samples were fresh-frozen collected after surgery in the following Polish and German centres: MSC Memorial Cancer Centre and Institute of Oncology in Gliwice, University of Leipzig, University of Halle, and Mainz University Hospital. The study was approved by the local ethics committees and informed consent was obtained from all patients. The core set of samples (after exclusion of technical outliers in genomic study) consisted of 52 tumours (27 FTC, 25 FTA), of which 23% were collected from men. When histological re-assessment of all samples was carried out, two specimens were re-classified as a poorly differentiated thyroid carcinoma and one as a follicular variant of papillary thyroid cancer. Nine samples, among them seven carcinomas and two adenomas, were considered as oxyphilic tumours.
Total RNA was extracted by RNEasy columns (QIAGEN). Samples were analysed by oligonucleotide microarray HG-U133 Plus 2.0 according to the manufacturer’s protocol (Affymetrix). The detailed methods are given in a parallel manuscript describing supervised analysis of the dataset (Pfeifer et al., submitted, [10]). The dataset was pre-processed with the RMA and GC-RMA methods, routine R/Bioconductor packages were applied. Principal Component Analysis (PCA) was applied as a main method of unsupervised analysis. The dataset for this analysis was centered, no scaling was applied. We also used our own procedure of unsupervised gene selection based on Singular Value Decomposition of the dataset [11]. The results of unsupervised analysis are so called Principal Components which could be visualised on X-Y plots, showing two principal components on each plot. Samples might be coloured according to the class membership, but this step is done after the procedure was carried out. The lists of genes were analysed in regard of their Gene Ontology content and hierarchical clustering based on the lists was presented. The MSigDB database (Molecular Signatures Database, Broad Institute, MIT) was also used to analyse the biological meaning of selected gene lists. For each mode, an analysis of significant gene sets, as defined by MSigDB repository and with the use of GSEA algorithm was carried out. We also performed the analysis of an independent collection of normal human tissues, as provided by GNF Institute (Genomics Institute of Novartis Research Foundation). This was done by clustering of a selected subset of GNF data (genes for each mode), and in parallel displaying the same clustering for our samples.
Results
Unsupervised analysis of the full dataset
The first step was to apply Principal Component Analysis to all the samples obtained in the study. Principal Components #1 and #2 are shown on Fig. 1A. It is evident that certain samples (mainly carcinomas, but also three adenomas) are clear outliers in gene expression profile (they have clearly different profile to the majority of samples in the analysed group). Some of these effects might be influenced by the biological differences in these samples, but probably they are partially related to the technical issues (e.g. the extent of RNA degradation). When the same analysis was repeated after exclusion of 11 outlier samples (Fig. 1B), the clear pattern of differences between “normal” thyroid tissue (sample from the lobe contralateral to the tumour, depicted in red) and thyroid adenomas as well as carcinomas (depicted in green and blue) was seen.
A

B

Figure 1. Unsupervised analysis of the whole dataset (A) and after exclusion of 11 outlier samples (B). First two principal components are presented (PC#1 on X axis and PC#2 on Y axis). Normal thyroid samples (red dots), follicular thyroid adenomas (FTA, green) and follicular carcinomas (FTC, blue) appear in three overlapping clusters
Rycina 1. Nienadzorowana analiza całego zbioru danych (A) oraz zbioru danych po wyłączeniu 11 próbek odstających (B). Pierwsze dwie główne składowe przedstawiono na wykresie (składową 1 — PC#1 na osi X, składową 2 — PC#2 na osi Y). Próbki pochodzące z utkania tarczycy niezmienionej nowotworowo zaznaczono na czerwono, gruczolaki pęcherzykowe tarczycy na zielono, a raki pęcherzykowe tarczycy na niebiesko. Wyraźne jest znaczne nakładanie się tych trzech grup badanych
First and third Principal Components discriminate between carcinomas and adenomas
In the next step, two components which best discriminated between FTC and FTA, i.e. PC#1 and PC#3, were used together to provide unsupervised insight into differences between both groups (Fig. 2). Similarly to the previous observation, there was a trend towards differences in gene expression profile of follicular carcinomas (high values of PC#1, low of PC#3) and follicular adenomas (low values of PC#1, high of PC#3). When the artificial boundary between both clusters was arbitrarily drawn (black line), still the significant number of samples were misclassified. In the case of the adenoma cluster, at least four carcinomas were dispersed within, with one additional lying close to the group boundary. Within the cluster of carcinomas, at least three adenomas were indistinguishable in an unsupervised manner, with additional three within the ‘grey zone’. With the assumption of the boundary set by us, the unsupervised classification was concordant with the class membership in 76% of samples in the ‘left’ cluster dominated by carcinomas and in 79% of ‘right’ cluster samples, dominated by adenomas. These relatively high estimates confirm the significant genomic differences between two classes, simultaneously pointing out the outlier samples.

Figure 2. Unsupervised analysis of adenoma (FTA, red) and carcinoma samples (FTC, blue) by PC#1 and PC#3, outlier samples were excluded. Potential boundary between two clusters is marked by black line, with the number of outliers provided
Rycina 2. Analiza nienadzorowana zbioru gruczolaków pęcherzykowych (FTA, zaznaczone na czerwono) oraz raków pęcherzykowych (FTC, zaznaczone na niebiesko). Wykorzystano dwie główne składowe, składową pierwszą (PC#1) i trzecią (PC#3), wyłączono próbki znacznie odstające. Zaznaczono możliwą granicę między dwoma klastrami (czarna ciągła linia), przedstawiono także liczbę próbek nie poddających się takiej próbie klasyfikacji
Unsupervised analysis of all adenomas and carcinomas in the context of tumour histology
In the third step, we used the full set of 52 carcinomas and adenomas, with no exclusions (Fig. 3). Again, we might notice the difference between two populations when PC#1 and PC#3 are considered, although less visible than in the previous figure. When the information about histological parameters of samples was used to colour the points, it became evident that some of the outlier samples showed oncocytic features of the tumour, while the majority of non-oncocytic tumours cluster were within the main group of tumours. Also, two poorly differentiated tumours showed certain features of similarity to the group of outliers, although not evident enough to be identified in an unsupervised manner. Of interest, the one follicular variant of PTC which appeared in the analysed group did not differ strongly from the remaining follicular tumours in the context of PC#1 and PC3# variability.
A

B

Figure 3. Analysis of the core set of samples (without exclusion of outliers) by Principal Component Analysis. PC#1 and PC#3 are shown. A. It is apparent that neither PC#1 nor PC#3 provides the differentiation between carcinomas and adenomas; it is necessary to use the information from both principal components. B. When the same plot was coloured according to the oxyphilic features of tumours (red), some of these tumours appear to be the outliers with very low values of PC#1. Two poorly differentiated carcinomas (black) are also showing the relatively low values of PC#1. One sample inadvertently used in the study (follicular variant of papillary thyroid carcinoma, fvPTC, green) is not significantly outlying from the remaining follicular tumours
Rycina 3. Analiza głównego zbioru próbek (tylko raki i gruczolaki pęcherzykowe, ale bez wyłączania próbek odstających) za pomocą analizy głównych składowych. Przedstawiono dwie główne składowe, składową pierwszą (PC#1) i trzecią (PC#3). A. Wyraźnie widać że ani składowa pierwsza ani trzecia samodzielnie nie pozwalają na rozróżnienie obu grup, trzeba wykorzystać obie składowe równocześnie. B. Kiedy wykorzystano informacje dot. badań histopatologicznych próbki i zaznaczono te guzy w których stwierdzano cechy oksyfilności (czerwone); część z tych guzów ma charakter silnie odstający, z niskimi wartościami PC#1. Dwa niskozróżnicowane raki tarczycy (czarne) również wykazują względnie niskie wartości PC#1 w porównaniu z resztą próbek. Jedna z trzech próbek reklasyfikowanych (rak brodawkowaty wariant pęcherzykowy) wykazuje profil względnie zbliżony do pozostałych guzów
The biological meaning of observed differences
We carried out an extensive analysis of the biological character of genes correlated to the first six Principal Components. When genes, correlated to the first PC, were used to cluster carcinoma and adenoma samples, they appeared in two clusters: one with larger homogenous expression of subset of genes, relatively enriched in adenomas, and the other with various degrees of down-regulation of these genes and heterogeneous up-regulation of smaller cluster of transcripts, see Fig. 4A; the second cluster shows the relative enrichment of carcinomas. One may note that the clustering by the major source of variability provided by the first Principal Component is related neither to the tumour batch nor to the hybridisation batch, thus, the analysed variability does not arise from the technical factors (Fig. 4A).
When the analysed clustering was shown parallel to the clustering by the same list of genes (presented in the same order) on the collection of healthy human tissues (made available by Genomics Institute of Novartis Research Foundation, Fig. 4B) it was revealed that genes highly up-regulated, mainly in adenomas, were the thyroid-specific transcripts, some of them up-regulated also in liver (deiodinases). In the opposite, the second cluster of genes, with heterogeneous expression in the dendrogram branch enriched in carcinomas, contained immunity-related transcripts like HLA-DR, DQ genes and may be a genomic indicator of tissue infiltration by lymphocytes and other immune system cells.
In a similar manner, we analysed genomic context of the next Principal Components. Immunity-related transcripts were identified as those influencing the first, third and sixth principal components. First and third principal components were similar in their genetic content, as they contained the immunityrelated genes and provided the best discrimination between carcinomas and adenomas; third PC genes were not as apparently thyroid-related as PC#1 genes. Within the PC#4 we identified a large number of genes related to mitochondrial function; we consider this component as directly related to the tumour oxyphilicity features [12]. Within the fifth principal component, there were a large number of genes related to cell cycle and proliferation. A full analysis of the data is provided as a web appendix to this article, available online at www.zmnieo.home.pl.
A

B

Figure 4. Clustering of samples and genes by the gene list of transcripts correlated to the first Principal Component (PC#1). A. Clustering of the set of follicular carcinomas (FTC, blue) and adenomas (FTA, green). Sample batch and hybridisation batch are provided by colour to demonstrate that these two are not behind the observed variability. Left cluster of samples is relatively enriched in adenomas, while the right branch of dendrograms contains a large proportion of carcinomas
Rycina 4. Klasteryzacja próbek i genów za pomocą listy transkryptów skorelowanych z pierwszą główną składową (PC#1). A. Klasteryzacja zbioru raków (FTC, niebieskie) i gruczolaków pęcherzykowych (FTA, zielone). Seria próbki oraz hybrydyzacji mikromacierzowej są również zaznaczone kolorem, nie są one ewidentnie związane z głównymi źródłami zmienności. Lewy klaster dendrogramu zawiera nieco więcej gruczolaków, podczas gdy prawa gałąź grupuje więcej raków pęcherzykowych
Discussion
In our unsupervised analysis of follicular thyroid tumours, we identified the following sources of variability: oncocytic features [12], cell cycle and proliferation genes [13], immunity-related transcripts and expression of thyroid-specific genes [14].
Most of these have been shown previously, except immunity-related transcripts. Putting these factors in order of importance is not a trivial task, although it seems evident that thyroid-specific genes are the largest and strongest factor appearing in our analysis. On the other hand, immune response seems to be the most multifactorial and complicated variable, influencing to a lesser extent at least three principal components of the analysis.
In our previous analysis of papillary thyroid cancer (PTC) and contralateral normal thyroid tissues, we applied unsupervised analysis to seek for the potential sources of variability [15]. We found that the difference between PTC and normal thyroid was prominent and could be seen as the major source of variance. We also noted that immunity-related transcripts were the other strong factor influencing the overall profile of gene expression. We hypothesised that the variable expression of these transcripts was both endogenous (coming from expression in thyreocytes) and exogenous (transcripts from the immune cells infiltrating the tumour).
In the study we found that at least in certain follicular tumours it is possible to apply unsupervised analysis and discriminate between FTC and FTA. Approx. 80% of tumours could easily be discriminated between clusters, while the remaining samples lie in between and could not be distinguished. It is certainly difficult to judge whether histopathological diagnosis of the analysed samples is accurate, and whether some of the follicular adenomas are in fact tumours with gene expression profile of cancer, but do not manifest the invasive properties. Also the opposite misclassification (falsely positive diagnosis of cancer) could influence results. Our previous studies on the accuracy of histopathological diagnosis of thyroid cancer [16] indicate that the misclassification rate is substantial, even among experienced histopathologists.
There have been a number of genomic studies dealing with the classification of follicular thyroid tumours by genomic tests [17–22]. The recent paper by Alexander et al. provides a clinically useful tool to discriminate gene expression patterns of thyrocytes even in fine-needle aspiration biopsy [23–24]. However, all the studies carried out until now do not provide the ideal classification accuracy for follicular tumours, and they claim a misclassification rate of between 5% and 30%. Thus, we undertook our study to verify the genomic distance between FTC and FTA and test whether it is large enough to provide good results of molecular classifiers. Our previous analyses [25] showed that for papillary thyroid cancer the genomic distance is high, and thus the misclassification rate might be very low. For follicular neoplasms analysed in the present study, we observed significant overlap between the two datasets and we estimate that the misclassification rate must be between 10% and 20% in an unsupervised approach.
Conclusions
In conclusion, the difference between follicular thyroid carcinoma and thyroid adenoma is moderate. Approx. 80% of samples could be discriminated in unsupervised analysis, while the remaining samples constitute the grey zone and could not be discriminated in that way. At least two principal components are necessary to provide such discrimination, confirming the multifactorial mechanisms driving the invasive potential of follicular carcinomas. Partially the expression of thyroid-specific genes and immunity-related transcripts improves the unsupervised classification of follicular tumours. The influence of oxyphilic character of some thyroid tumours might also be a strong factor appearing as a strong source of gene expression variability.
References
1. Nikiforova MN, Lynch RA, Biddinger PW et al. RAS point mutations and PAX8-PPAR gamma rearrangement in thyroid tumors: evidence for distinct molecular pathways in thyroid follicular carcinoma. J Clin Endocrinol Metab 2003; 88: 2318–2326.
2. Finley DJ, Zhu B, Fahey TJ3rd. Molecular analysis of Hurthle cell neoplasm by gene profiling. Surgery 2004; 136: 1160–1168.
3. Lubitz CC, Gallagher LA, Finley DJ et al. Molecular analysis of minimally invasive follicular carcinomas by gene profiling. Surgery 2005; 138: 1042–1048; discussion 1048–1049.
4. Cerutti JM, Oler G, Delcelo R et al. PVALB, a new Hürthle adenoma diagnostic marker identified through gene expression. J Clin Endocrinol Metab 2011; 96: E151–160.
5. Lang W, Georgii A, Stauch G et al. The differentiation of atypical adenomas and encapsulated follicular carcinomas in the thyroid gland. Virchows Arch A Pathol Anat Histol 1980; 385: 125–141.
6. Lange D, Sporny S, Sygut J et al. Histopathological diagnosis of thyroid cancer in a multicenter trial. Endokrynol Pol 2006; 57: 336–342.
7. Lange D, Sporny S, Sygut J et al. Criteria for histopathologic diagnosis of thyroid cancer in a quick test of accuracy. Wiad Lek 2001; 54 (Suppl. 1): 54–61.
8. Weber F, Eng C. Update on the molecular diagnosis of endocrine tumors: toward -omics-based personalized healthcare? J Clin Endocrinol Metab 2008; 93: 1097–1104.
9. Weber F, Eng C. Gene-expression profiling in differentiated thyroid cancer – a viable strategy for the practice of genomic medicine? Future Oncol 2005; 1: 497–510.
10. Pfeifer A, Wojtas B, Oczko-Wojciechowska et al. Molecular differential diagnosis of follicular thyroid carcinoma and adenoma based on gene expression profiling by using formalin-fixed paraffin-embedded tissues. BMC Med Genomics 2013; 6: 38.
11. Simek K, Jarząb M. SVD Analysis of Gene Expression Data. Mathematical Modeling of Biological Systems, Vol. I, Modeling and Simulation in Science, Engineering and Technology 2007: 361–372.
12. Baris O, Savagner F, Nasser V et al. Transcriptional profiling reveals coordinated up-regulation of oxidative metabolism genes in thyroid oncocytic tumors. J Clin Endocrinol Metab 2004; 89: 994–1005.
13. Troncone G, Volante M, Iaccarino A et al. Cyclin D1 and D3 overexpression predicts malignant behavior in thyroid fine-needle aspirates suspicious for Hurthle cell neoplasms. Cancer 2009; 117: 522–529.
14. Di Cristofaro J, Silvy M, Lanteaume A et al. Expression of tpo mRNA in thyroid tumors: quantitative PCR analysis and correlation with alterations of ret, Braf, ras and pax8 genes. Endocr Relat Cancer 2006; 13: 485–495.
15. Jarzab B, Wiench M, Fujarewicz K et al. Gene expression profile of papillary thyroid cancer: sources of variability and diagnostic implications. Cancer Res 2005; 65: 1587–1597.
16. Lange D, Sporny S, Sygut J et al. Differential criteria between papillary and follicular thyroid carcinoma – initial conclusions from a multicenter trial. Wiad Lek 2001; 54 (Suppl. 1): 42–53.
17. Chevillard S, Ugolin N, Vielh P et al. Gene expression profiling of differentiated thyroid neoplasms: diagnostic and clinical implications. Clin Cancer Res 2004; 10: 6586–6597.
18. Chudova D, Wilde JI, Wang ET et al. Molecular classification of thyroid nodules using high-dimensionality genomic data. J Clin Endocrinol Metab 2010; 95: 5296–5304.
19. Borup R, Rossing M, Henao R et al. Molecular signatures of thyroid follicular neoplasia. Endocr Relat Cancer 2010; 17: 691–708.
20. Ferraz C, Eszlinger M, Paschke R. Current state and future perspective of molecular diagnosis of fine-needle aspiration biopsy of thyroid nodules. J Clin Endocrinol Metab 2011; 96: 2016–2026.
21. Karger S, Krause K, Gutknecht M et al. ADM3, TFF3 and LGALS3 are discriminative molecular markers in fine-needle aspiration biopsies of benign and malignant thyroid tumours. Br J Cancer 2012; 106: 562–568.
22. Eszlinger M, Krogdahl A, Muenz S et al. Impact of molecular screening for point mutations and rearrangements in routine air-dried Fine Needle Aspiration samples of thyroid nodules. Thyroid 2013; epub ahead of print.
23. Alexander EK, Kennedy GC, Baloch ZW et al. Preoperative diagnosis of benign thyroid nodules with indeterminate cytology. N J Engl Med 2012; 367: 705–715.
24. Kloos RT, Reynolds JD, Walsh PS et al. Does addition of BRAF V600E mutation testing modify sensitivity or specificity of the Afirma Gene Expression Classifier in cytologically indeterminate thyroid nodules? J Clin Endocrinol Metab 2013; 98: E761–768.
25. Fujarewicz K, Jarzab M, Eszlinger M et al. A multi-gene approach to differentiate papillary thyroid carcinoma from benign lesions: gene selection using support vector machines with bootstrapping. Endocr Relat Cancer 2007; 14: 809–826.
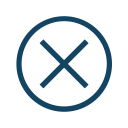