Impact of calcification on Murray law-based quantitative flow ratio for physiological assessment of intermediate coronary stenoses
Abstract
Background: To investigate the influence of coronary calcification on the diagnostic performance of Murray law-based quantitative flow ratio (μQFR) in identifying hemodynamically significant coronary lesions referenced to fractional flow reserve (FFR).
Methods: A total of 571 intermediate lesions from 534 consecutive patients (66.1 ± 10.0 years, 67.2% males) who underwent coronary angiography and simultaneous FFR measurement were included. Calcific deposits were graded by angiography as none or mild (spots), moderate (involving ≤ 50% of the reference vessel diameter), and severe (> 50%). Performance of μQFR to detect functional ischemia (FFR ≤ 0.80) was evaluated, including diagnostic parameters and areas under the receiver-operating curves (AUCs).
Results: The discrimination of ischemia by μQFR was comparable between none/mild and moderate/severe calcification (AUC: 0.91 [95% confidence interval: 0.88–0.93] vs. 0.87 [95% confidence interval: 0.78–0.94]; p = 0.442). No statistically significant difference was observed for μQFR between the two categories in sensitivity (0.70 vs. 0.69, p = 0.861) and specificity (0.94 vs. 0.90, p = 0.192). Moreover, μQFR showed significantly higher AUCs than quantitative coronary angiographic diameter stenosis in both vessels with none/mild (0.91 vs. 0.78, p < 0.001) and moderate/severe calcification (0.87 vs. 0.69, p < 0.001). By multivariable analysis, there was no association between calcification and μQFR-FFR discordance (adjusted odds ratio: 1.529, 95% confidence interval: 0.788–2.968, p = 0.210) after adjustment for other confounding factors.
Conclusions: μQFR demonstrated robust and superior diagnostic performance for lesion-specific ischemia compared with angiography alone regardless of coronary calcification.
Keywords: calcificationfractional flow reservecoronary artery diseasediagnosisquantitative flow ratio
References
- Tonino PAL, Bruyne BDe, Pijls N, et al. Fractional flow reserve versus angiography for guiding percutaneous coronary intervention. N Engl J Med. 2009; 360(3): 213–224.
- De Bruyne B, Fearon W, Pijls N, et al. Fractional flow reserve–guided PCI for stable coronary artery disease. N Engl J Med. 2014; 371(13): 1208–1217.
- Dehmer GJ, Weaver D, Roe MT, et al. A contemporary view of diagnostic cardiac catheterization and percutaneous coronary intervention in the United States: a report from the CathPCI Registry of the National Cardiovascular Data Registry, 2010 through June 2011. J Am Coll Cardiol. 2012; 60(20): 2017–2031.
- Tu S, Westra J, Adjedj J, et al. Fractional flow reserve in clinical practice: from wire-based invasive measurement to image-based computation. Eur Heart J. 2020; 41(34): 3271–3279.
- Xu Bo, Tu S, Qiao S, et al. Diagnostic accuracy of angiography-based quantitative flow ratio measurements for online assessment of coronary stenosis. J Am Coll Cardiol. 2017; 70(25): 3077–3087.
- Jelmer W, Krogsgaard AB, Gianluca C, et al. Diagnostic performance of in-procedure angiography-derived quantitative flow reserve compared to pressure-derived fractional flow reserve: the FAVOR II Europe-Japan study. J Am Heart Assoc. 2018; 7(14): e009603.
- Westra J, Tu S, Winther S, et al. Evaluation of coronary artery stenosis by quantitative flow ratio during invasive coronary angiography: the WIFI II study (Wire-Free Functional Imaging II). Circ Cardiovasc Imaging. 2018; 11(3): e007107.
- Tu S, Ding D, Chang Y, et al. Diagnostic accuracy of quantitative flow ratio for assessment of coronary stenosis significance from a single angiographic view: a novel method based on bifurcation fractal law. Catheter Cardiovasc Interv. 2021; 97 Suppl 2: 1040–1047.
- Karacsonyi J, Karmpaliotis D, Alaswad K, et al. Impact of calcium on chronic total occlusion percutaneous coronary interventions. Am J Cardiol. 2017; 120(1): 40–46.
- Zuo W, Sun R, Zhang X, et al. The association between quantitative flow ratio and intravascular imaging-defined vulnerable plaque characteristics in patients with stable angina and non-ST-segment elevation acute coronary syndrome. Front Cardiovasc Med. 2021; 8: 690262.
- Zuo W, Zhang X, Carvalho A, et al. Impact of diabetes mellitus on the relationship between a Poiseuille-based index and fractional flow reserve in intermediate coronary lesions. Coron Artery Dis. 2021; 32(7): 632–638.
- Hinkle D, Wiersma W, Jurs S. Applied statistics for the behavioral sciences. 2nd ed. Houghton Mifflin Company, Boston 1988.
- Richardson JTE. The analysis of 2 × 2 contingency tables: yet again. Stat Med. 2011; 30(8): 890; author reply 891–890; author reply 892.
- DeLong ER, DeLong DM, Clarke-Pearson DL. Comparing the areas under two or more correlated receiver operating characteristic curves: a nonparametric approach. Biometrics. 1988; 44(3): 837–845.
- Dey D, Lin A. Machine-learning CT-FFR and extensive coronary calcium: overcoming the Achilles heel of coronary computed tomography angiography. JACC Cardiovasc Imaging. 2020; 13(3): 771–773.
- Nørgaard BL, Gaur S, Leipsic J, et al. Influence of coronary calcification on the diagnostic performance of CT angiography derived FFR in coronary artery disease: a substudy of the NXT trial. JACC Cardiovasc Imaging. 2015; 8(9): 1045–1055.
- Tesche C, Otani K, De Cecco CN, et al. Influence of coronary calcium on diagnostic performance of machine learning CT-FFR: results from MACHINE registry. JACC Cardiovasc Imaging. 2020; 13(3): 760–770.
- Di Jiang M, Zhang XL, Liu H, et al. The effect of coronary calcification on diagnostic performance of machine learning-based CT-FFR: a Chinese multicenter study. Eur Radiol. 2021; 31(3): 1482–1493.
- Kobayashi Y, Collet C, Achenbach S, et al. Diagnostic performance of angiography-based fractional flow reserve by patient and lesion characteristics. EuroIntervention. 2021; 17(4): e294–e300.
- Tu S, Westra J, Yang J, et al. Diagnostic accuracy of fast computational approaches to derive fractional flow reserve from diagnostic coronary angiography: the international multicenter FAVOR pilot study. JACC Cardiovasc Interv. 2016; 9(19): 2024–2035.
- Yu M, Li Y, Li W, et al. Calcification remodeling index assessed by cardiac CT predicts severe coronary stenosis in lesions with moderate to severe calcification. J Cardiovasc Comput Tomogr. 2018; 12(1): 42–49.
- Borren NM, Ottervanger JP, Mouden M, et al. Influence of coronary calcification on hyperemic response during fractional flow reserve measurements. Int J Cardiol. 2019; 285: 93–96.
- Zuo W, Zhang R, Yang M, et al. Clinical prediction models of fractional flow reserve: an exploration of the current evidence and appraisal of model performance. Quant Imaging Med Surg. 2021; 11(6): 2642–2657.
- Velangi PS, Maharaj V, Athwal SS, et al. Computed tomography coronary plaque characteristics predict ischemia detected by invasive fractional flow reserve. J Thorac Imaging. 2021; 36(6): 360–366.
- Shi X, Gao J, Lv Q, et al. Calcification in atherosclerotic plaque vulnerability: friend or foe? Front Physiol. 2020; 11: 56.
- Hsu JJ, Tintut Y, Demer LL. Lipids and cardiovascular calcification: contributions to plaque vulnerability. Curr Opin Lipidol. 2021; 32(5): 308–314.
- Kovacic JC, Lee P, Baber U, et al. Inverse relationship between body mass index and coronary artery calcification in patients with clinically significant coronary lesions. Atherosclerosis. 2012; 221(1): 176–182.
- Akobeng AK. Understanding diagnostic tests 1: sensitivity, specificity and predictive values. Acta Paediatr. 2007; 96(3): 338–341.
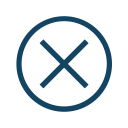