- Original article
Is neural network better than logistic regression in death prediction in patients after ST-segment elevation myocardial infarction?
Jacek T Niedziela1, 2, Daniel Cieśla3, Wojciech Wojakowski4, Marek Gierlotka5, Dariusz Dudek6, Adam Witkowski7, Tomasz Zdrojewski8, Maciej Lesiak9, Paweł Buszman10, Mariusz Gąsior1, 2
13rd Department of Cardiology, Faculty of Medical Sciences in Zabrze, Medical University of Silesia, Katowice, Poland
23rd Department of Cardiology, Silesian Center for Heart Disease, Zabrze, Poland
3Department of Science, Education and New Medical Technologies, Silesian Center for Heart Disease, Zabrze, Poland
4Department of Cardiology and Structural Heart Diseases, Medical University of Silesia, Katowice, Poland
5Department of Cardiology, Institute of Medical Sciences, University of Opole, Opole, Poland
62nd Department of Clinical Cardiology and Cardiovascular Interventions, Institute of Cardiology, Jagiellonian University Medical College, Kraków, Poland
7Department of Interventional Cardiology and Angiology, Institute of Cardiology, Warszawa, Poland
8Department of Preventive Medicine and Education, Medical University of Gdansk, Gdańsk, Poland
9Department of Cardiology, Poznan University of Medical Sciences, Poznań, Poland
10American Heart of Poland, Katowice, Poland
Correspondence to:
3rd Department of Cardiology, Silesian Center for Heart Disease,
M Curie-Skłodowskiej 9, 41–800 Zabrze, Poland,
phone: +48 323 733 860,
e-mail: jacek@niedziela.org
Copyright by the Author(s), 2021
Kardiol Pol. 2021; 79 (12): 1353–1361; DOI: 10.33963/KP.a2021.0142
Received: August 29, 2021
Revision accepted: October 24, 2021
Published online: October 25, 2021
WHAT’S NEW? Mortality rates after myocardial infarction (MI) are high. We aimed to use a modern statistical method based on neural network and machine learning techniques to improve the identification of patients with the highest death risk after MI. The study compared the predictive power of neural network (NN) and logistic regression (LR) related to post-discharge mortality in patients with first anterior ST-segment elevation myocardial infarction (STEMI) treated with the primary percutaneous coronary intervention (pPCI) of the left anterior descending (LAD) artery and discharged alive. Both models had good predictive power, with better results in the NN approach — the area under the receiver operating characteristics curve (AUROC) was 0.8103 vs. 0.7939 for LR (P = 0.037) in the test groups. The NN may have better predictive power than LR in patients after the first STEMI treated with pPCI. |
INTRODUCTION
Ischemic heart disease (IHD) is the single most common cause of death worldwide. It is responsible for 19%–20% of deaths in Europe [1]. The clinical presentation of IHD may vary from stable coronary artery disease to acute coronary syndromes (ACS). The particular type of ACS is ST-segment elevation myocardial infarction (STEMI), characterized by a sudden clinical course and high mortality due to a lack of the appropriate reperfusion therapy. Despite the decrease in incidence and mortality associated with improved in-hospital treatment (mainly reperfusion therapy) and secondary prevention, the one-year mortality after STEMI treated with the primary percutaneous coronary intervention (pPCI) is still high. It varies between 7.3% and 11.4% [2–4]. In the Swedish SWEDEHEART Registry in the years 2006–2015, one-year mortality after STEMI fluctuated at around 15% [5]. For that reason, there is an urgent need to classify STEMI patients at hospital discharge in terms of the risk of death, which may allow adjusting the post-discharge care according to the estimated individual risk [6]. Such an approach may result in post-discharge mortality reduction. Hitherto, the most popular statistical method used in the risk stratification was logistic regression (LR). In the last years, in parallel with the development of data sets and improvement of computer power, an artificial intelligence (AI) with a neural network (NN) and deep learning (DL) approach was implemented as an adjunct or alternative method for LR [7].
Analyzing the material containing a large sample of STEMI patients from the Polish Registry of Acute Coronary Syndromes (PL-ACS) with complete follow-up, we decided to compare the most refined LR with the most advanced NN analysis to assess the accuracy of post-discharge mortality prediction.
METHODS
The PL-ACS Registry is the first national, multicenter, and prospective Polish medical registry, in which clinical and procedural data on ACS patients have been recorded since 2003 [8]. The registry is a joint initiative of the Silesian Center for Heart Disease in Zabrze and the Polish Ministry of Health. The data on more than 700 000 ACS from the whole country have been gathered. The complete follow-up containing all-cause mortality was obtained from the National Health Fund, the only Polish non-commercial medical insurer [9].
Material
The population of 175 895 patients with ACS was analyzed, of which anterior STEMI treated with pPCI in LAD was found in 21 420 individuals discharged alive between 2009 and 2015. Patients with previous ACS and thrombolysis and coronary angioplasty of arteries other than LAD were excluded, and 17 793 patients were included in the analysis.
Statistical analysis
Continuous variables were presented as the means and standard deviations (SD) or medians and interquartile range (IQR). Categorical variables were presented as percentages. The chi-squared, Mann-Whitney U, and Student’s t-tests were used where appropriate to test for differences among the patients who survived and died in a 6-month follow-up after the onset of STEMI. Yates’ correction of the chi-square test was used in the case of minor cell frequency. The multiple imputation method was applied to impute the missing data to minimize the missing data’s impact on LR and NN analyses. None of the variables had more than 2% of missing values. The study sample was randomly divided into three groups in the NN approach: learning (60%), validation (20%), and test (20%). Only learning and test groups were used in the LR approach to provide comparable results between LR and NN. Both methods were tested in the same patient population (test group), which was not used in the learning process. The informative value (IV), an entropy-based parameter established in many analytical fields to calculate a firlds to describe a predictive value of the single variable, was calculated for all the available variables. The covariates used in the stepwise forward LR and NN included all parameters with IV ≥0.02 (Table 1).
Table 1. Baseline characteristics
|
Survived (n = 16 735) |
Died (n = 1 058) |
P-value |
IV |
Age, years, mean (SD) |
62.9 (12.3) |
72.6 (11.7) |
<0.01 |
0.43 |
Female gender, n (%) |
5 297 (31.7) |
458 (43.3) |
<0.01 |
0.06 |
SBP, mm Hg, mean (SD) |
137.5 (25.2) |
128.3 (28.3) |
<0.01 |
0.12 |
DBP, mm Hg, mean (SD) |
82.9 (14.2) |
78.4 (15.6) |
<0.01 |
0.10 |
HR, beats/min, mean (SD) |
81.1 (16.6) |
89.0 (21.1) |
<0.01 |
0.18 |
Heart rhythm at admission, n (%) |
|
|
<0.01 |
0.14 |
Sinus |
16 044 (95.9) |
905 (85.5) |
||
AF |
571 (3.4) |
132 (12.5) |
||
Pacemaker |
21 (0.1) |
5 (0.5) |
||
Other |
99 (0.6) |
16 (1.5) |
||
QRS morphology, n (%) |
|
|
<0.01 |
0.07 |
Normal |
14 982 (89.5) |
857 (81.0) |
||
LBBB |
244 (1.5) |
41 (3.9) |
||
RBBB |
427 (2.5) |
65 (6.1) |
||
Other |
1,082 (6.5) |
95 (9.0) |
||
Weight, kg, mean (SD) |
79.5 (12.9) |
76.3 (12.6) |
<0.01 |
0.13 |
Height, cm, mean (SD) |
169.3 (6.9) |
167.7 (6.6) |
<0.01 |
0.10 |
BMI, kg/m2, mean (SD) |
27.7 (3.7) |
27.2 (3.8) |
<0.01 |
0.05 |
Killip class, n (%) |
|
|
<0.01 |
0.34 |
1 |
13 719 (82.0) |
633 (59.8) |
||
2 |
2 532 (15.1) |
255 (24.1) |
||
3 |
266 (1.6) |
75 (7.1) |
||
4 |
218 (1.3) |
95 (9.0) |
||
Cardiac arrest before admission, n (%) |
198 (1.2) |
(1.9) |
0.11 |
<0.01 |
EF, %, mean (SD) |
44.7 (9.2) |
37.6 (11.1) |
<0.01 |
0.55 |
Length of stay, days, median (IQR) |
5 (2–7) |
5 (2–8) |
0.42 |
0.20 |
Admission from home, n (%) |
9 840 (58.8) |
564 (53.3) |
<0.01 |
0.01 |
Medical history |
||||
Hypertension, n (%) |
10 836 (64.8) |
691 (65.3) |
0.71 |
<0.01 |
Hyperlipidemia, n (%) |
6 337 (37.9) |
358 (33.8) |
<0.01 |
0.01 |
Diabetes, n (%) |
3 362 (20.1) |
334 (31.6) |
<0.01 |
0.07 |
Obesity, n (%) |
3 230 (19.3) |
182 (17.2) |
0.09 |
<0.01 |
Smoking, n (%) |
10 494 (62.7) |
528 (49.9) |
<0.01 |
0.07 |
Coronary artery disease, n (%) |
878 (5.2) |
92 (8.7) |
<0.01 |
0.02 |
Coronary angioplasty, n (%) |
301 (1.8) |
20 (1.9) |
0.88 |
<0.01 |
CABG, n (%) |
167 (0.1) |
32 (0.3) |
0.11 |
<0.01 |
Heart failure, n (%) |
739 (4.4) |
120 (11.3) |
<0.01 |
0.07 |
Stroke, n (%) |
348 (2.1) |
65 (6.1) |
<0.01 |
0.05 |
Chronic kidney disease, n (%) |
469 (2.8) |
107 (10.1) |
<0.01 |
0.10 |
Peripheral artery disease, n (%) |
366 (2.2) |
54 (5.1) |
<0.01 |
0.03 |
Pulmonary disease, n (%) |
384 (2.3) |
58 (5.5) |
<0.01 |
0.03 |
Abbreviations: AF, atrial fibrillation; BMI, body mass index; bpm, beats per minute; CABG, coronary artery bypass graft; DBP, diastolic blood pressure; EF, ejection fraction; HR, heart rate; IQR, interquartile range; IV, information value; LBBB, left bundle branch block; RBBB, right bundle branch block; SBP, systolic blood pressure; SD, standard deviation
The selection of representatives was performed, and only one variable from each correlated parameter group was included. The discretization of continuous variables was performed, and all the continuous variables were categorized. Eventually, 42 variables were included in each analysis: age, sex, body mass index, systolic blood pressure on admission, time from the hospital admission to PCI, heart rhythm on admission, bundle branch block, the principal symptom of ACS, the Killip class on admission, a history of diabetes, coronary artery disease, heart failure, stroke, chronic kidney disease, peripheral artery disease, chronic obstructive pulmonary disease or smoking, in-hospital treatment (β-blockers, angiotensin-converting enzyme inhibitors [ACEI], diuretics, insulin, inotropes, glycoprotein (GP) IIb/IIIa, intra-aortic balloon pump use, type of stent — metal, drug-eluting stent, or none), thrombolysis in myocardial infarction (TIMI) score after the PCI, in-hospital complications (pulmonary edema, cardiogenic shock, bleeding requiring blood transfusion, stroke, cardiac arrest), the length of hospital stay, the latest available New York Heart Association class, left ventricular ejection fraction, and treatment recommended at discharge (β-blockers, ACEI, statins, diuretics, insulin, acetylsalicylic acid, second antiplatelet drug, low-molecular-weight heparin). The significance level of P <0.05 was used to retain a stepwise forward LR model variable. The odds ratios and 95% confidence intervals were calculated. The classification feedforward fully-connected NN with one hidden layer and Multilayer Perceptrons was implemented. In the input layer, all 42 variables included in the LR model were added. During the learning process, each patient was presented as a new learning case. The algorithm repeatedly tried to match the variables’ weights to obtain the outcome’s best prediction (categorical variable in the output layer — death or alive). The hidden layer was constructed between the input and output layer, which allowed testing many configurations of variables and their weights (Figure 1).
Figure 1. The architecture of the classifying neural network. Each connection between the input and hidden layer and between hidden and the output layers has its unique weight (only selected variables are named in the figure) established in the learning process
Abbreviations: BFGS, Broyden-Fletcher-Goldfarb-Shanno algorithm; EF, ejection fraction
Different activation (linear, logistic, tanh, exponential, sinus) and error (sum-of-squares, cross-entropy) functions were tested with the Broyden-Fletcher-Goldfarb-Shanno algorithm. General sensitivity analysis of variables included in the NN model was performed, and parameters with marginal influence on the outcome (mean <1.001) were removed from the model one at a time, starting from the least relevant.
Clinical potential of the analyzed models was evaluated with Area Under the Receiver Operating Characteristics Curve (AUROC), which is not sensitive to class imbalance. AUROC is the area under the curve plotted for all possible cut-off thresholds for sensitivity as a function of 1-specificity. It ranges between 0 and 1, where 0.5 is random guessing, and 1 is a perfect classifier. Values >0.7 are considered as a clinically useful classifier [10]. The NN with the highest AUROC was selected and compared with the AUROC of the LR model [10]. All analyses were performed with the Statsoft Statistica 13.1 software (TIBCO Software, Palo Alto, CA, USA) using the STATISTICA Automated Neural Networks (SANN) module.
Ethics
All procedures were undertaken according to Helsinki Declaration, and the protocol was reviewed and accepted by the local Ethical Committee. All patients have expressed their informed consent.
RESULTS
The analysis included 17 793 patients with anterior STEMI without previous ACS treated with pPCI of LAD and discharged alive. The learning group included 10 675 individuals, while the validation and test groups comprised 3 559 patients each. Baseline characteristics are presented in Table 1. Characteristics of treatment and events during hospitalization and treatment recommended at hospital discharge are shown in Table 2.
Table 2. Treatment and events during hospitalization and treatment recommended at hospital discharge
|
Survived (n = 16 735) |
Died (n = 1 058) |
P-value |
IV |
Treatment during hospitalization |
||||
ASA, n (%) |
14 844 (88.7) |
919 (86.9) |
<0.01 |
<0.01 |
Other antiplatelet drug, n (%) |
16 518 (98.7) |
1 033 (97.6) |
0.05 |
0.01 |
GP IIb/IIIa inhibitors, n (%) |
|
|
<0.01 |
0.04 |
None |
10 550 (63.0) |
742 (70.1) |
||
Abciximab |
3 013 (18.0) |
121 (11.4) |
||
Eptifibatide |
3 154 (18.9) |
194 (18.3) |
||
Tirofiban |
18 (0.1) |
1 (0.1) |
||
Beta-adrenolytic, n (%) |
12 978 (77.6) |
747 (70.6) |
<0.01 |
0.03 |
ACEI or ARB, n (%) |
12 083 (72.2) |
658 (62.2) |
<0.01 |
0.05 |
Statin, n (%) |
13 622 (81.40 |
817 (77.2) |
<0.01 |
0.01 |
Calcium blocker, n (%) |
1 038 (6.2) |
59 (5.6) |
0.41 |
<0.01 |
Nitrate, n (%) |
1 824 (10.9) |
136 (12.9) |
0.051 |
<0.01 |
MRA, n (%) |
1 690 (10.1) |
141 (13.3) |
<0.01 |
0.01 |
Diuretic, n (%) |
3 425 (20.5) |
400 (37.5) |
<0.01 |
0.15 |
Inotropes, n (%) |
289 (1.7) |
125 (11.8) |
<0.01 |
0.20 |
Insulin, n (%) |
1 341 (8.8) |
157 (14.8) |
<0.01 |
0.05 |
TIMI before PCI, n (%) |
|
|
<0.01 |
0.01 |
0 |
9 907 (59.2) |
682 (64.5) |
||
1 |
2 694 (16.1) |
162 (15.3) |
||
2 |
1 494 (14.9) |
129 (12.2) |
||
3 |
1 640 (9.8) |
85 (8.0) |
||
TIMI after PCI, n (%) |
|
|
<0.01 |
0.11 |
0 |
193 (1.2) |
32 (3.0) |
||
1 |
145 (0.9) |
34 (3.2) |
||
2 |
727 (4.3) |
109 (10.3) |
||
3 |
15 670 (93.6) |
883 (83.5) |
||
CABG, n (%) |
|
|
0.81 |
<0.01 |
Not planned |
16 283 (97.3) |
1 026 (97.0) |
||
During hospitalization |
134 (0.8) |
10 (0.9) |
||
Planned after discharge |
318 (1.9) |
22 (2.1) |
||
Number of PCI (in LAD), n (%) |
|
|
0.4 |
<0.01 |
1 |
15 965 (95.4) |
1 000 (94.5) |
||
2 |
636 (3.8) |
48 (4.5) |
||
3 |
134 (0.8) |
11 (1.0) |
||
Time from symptom onset to PCI, min, median (IQR) |
293 (33–554) |
372 (122–623) |
<0.01 |
0.04 |
Time from admission to PCI, min, median (IQR) |
42 (23–61) |
47 (23–71) |
<0.01 |
0.04 |
IABP, n (%) |
93 (0.6) |
46 (4.3) |
<0.01 |
0.08 |
Events during hospitalization |
||||
Pulmonary odema, n (%) |
99 (0.6) |
37 (3.5) |
<0.01 |
0.05 |
Shock, n (%) |
88 (0.5) |
60 (5.7) |
<0.01 |
0.13 |
Stroke, n (%) |
25 (0.1) |
11 (1.0) |
<0.01 |
0.02 |
Cardiac arrest, n (%) |
161 (1.0) |
53 (5.00 |
<0.01 |
0.07 |
Bleeding requiring blood transfusion, n (%) |
61 (0.4) |
21 (2.0) |
<0.01 |
0.03 |
Treatment at discharge |
||||
ASA, n (%) |
15 954 (95.3) |
964 (91.1) |
<0.01 |
0.03 |
Second antiplatelet drug, n (%) |
15 497 (92.6) |
929 (87.8) |
<0.01 |
0.02 |
Anticoagulation, n (%) |
904 (5.4) |
140 (13.2) |
<0.01 |
0.08 |
β-adrenolytic, n (%) |
14 841 (88.7) |
824 (77.9) |
<0.01 |
0.09 |
ACEI or ARB, n (%) |
14 325 (85.6) |
726 (68.6) |
<0.01 |
0.14 |
Statin, n (%) |
15 393 (92.0) |
907 (85.7) |
<0.01 |
0.04 |
Calcium blocker, n (%) |
1 038 (6.2) |
68 (6.4) |
0.77 |
<0.01 |
Nitrate, n (%) |
1 422 (8.5) |
81 (7.7) |
0.37 |
<0.01 |
Diuretic, n (%) |
4 413 (26.4) |
459 (43.4) |
<0.01 |
0.13 |
Insulin, n (%) |
1 122 (6.7) |
132 (12.5) |
<0.01 |
0.04 |
Abbreviations: ACEI, angiotensin-converting enzyme inhibitor; ARB, angiotensin II receptor blocker; ASA, acetylsalicylic acid; GP IIb/IIIa, glycoprotein IIb/IIIa; IABP, intra-aortic balloon pump; LAD, left anterior descending artery; MRA, mineralocorticoid receptor antagonist; PCI, percutaneous coronary intervention; TIMI, thrombolysis in myocardial infarction; other — see Table 1
Six-month mortality since the onset of STEMI was 5.9%. The results of the LR analysis are presented in Table 3.
Table 3. The results of logistic regression analysis – predictors of mortality in 6-month follow-up after the first anterior STEMI treated with pPCI of LAD
|
OR (95% CI) |
P-value |
Age 53–61 years (vs. <53) |
2.78 (1.80–4.28) |
<0.001 |
Age 62–74 years (vs. <53) |
3.72 (2.45–5.64) |
<0.0001 |
Age >74 years (vs. <53) |
9.18 (6.09–13.86) |
<0.0001 |
BMI 23–31 kg/m2 (vs. <23 kg/m2) |
0.67 (0.51–0.87) |
0.003 |
BMI >31 kg/m2 (vs. <23 kg/m2) |
0.48 (0.31–0.74) |
0.001 |
History of stroke |
1.72 (1.12–2.65) |
0.013 |
History of pulmonary disease |
1.56 (1.04–2.34) |
0.032 |
History of peripheral artery disease |
1.87 (1.23–2.85) |
0.003 |
History of heart failure |
1.40 (1.02–1.92) |
0.036 |
AF on admission ECG (vs. sinus) |
1.50 (1.10–2.05) |
0.01 |
Pacing on admission ECG (vs. sinus) |
1.03 (0.19–5.66) |
0.98 |
Other rhythm in admission ECG (vs. sinus) |
2.08 (0.96–4.49) |
0.06 |
LBBB on admission ECG (vs. normal) |
1.55 (0.94–1.56) |
0.086 |
RBBB on admission ECG (vs. normal) |
1.61 (1.07–2.45) |
0.024 |
Other intraventricular conduction delay on admission ECG (vs. normal) |
0.92 (0.65–1.29) |
0.62 |
SBP <110 mm Hg (vs. ≥130 mm Hg) |
1.49 (1.16–1.92) |
0.002 |
SBP 110–129 mm Hg (vs. ≥130 mm Hg) |
1.51 (1.23–1.85) |
<0.0001 |
Killip 2 vs. 1 |
1.18 (0.94–1.48) |
0.16 |
Killip 3 vs. 1 |
2.44 (1.60–3.70) |
<0.0001 |
Killip 4 vs. 1 |
3.27 (2.09–5.13) |
<0.0001 |
Inotropes during hospitalization |
1.54 (1.04–2.27) |
0.029 |
IABP during hospitalization |
2.38 (1.27–4.45) |
0.007 |
Shock during hospitalization |
1.99 (1.11–3.57) |
0.021 |
Stroke during hospitalization |
3.87 (1.22–12.31) |
0.022 |
Bleeding requiring blood transfusion |
2.66 (1.25–5.68) |
0.011 |
Cardiac arrest during hospitalization |
2.94 (1.73–4.99) |
<0.0001 |
EF <30% (vs. ≥55%) |
4.30 (2.53–7.32) |
<0.0001 |
EF 30%–39% (vs. ≥55%) |
2.09 (1.24–3.54) |
0.006 |
EF 40%–47% (vs. ≥55%) |
1.52 (0.90–2.56) |
0.12 |
EF 48–54 (vs. ≥55%) |
1.47 (0.84–2.57) |
0.18 |
Time from admission to PCI 37–59 min (vs. <37 min) |
1.06 (0.84–1.33) |
0.63 |
Time from admission to PCI ≥60 min (vs. <37 min) |
1.45 (1.17–1.81) |
0.001 |
TIMI after PCI 0 vs. 3 |
1.24 (0.66–2.33) |
0.51 |
TIMI after PCI 1 vs. 3 |
1.70 (0.94–3.08) |
0.08 |
TIMI after PCI 2 vs. 3 |
1.51 (1.09–2.10) |
0.014 |
LOS 3–4 days (vs. <3 days) |
0.46 (0.36–0.59) |
<0.0001 |
LOS 5–9 days (vs. <3 days) |
0.50 (0.39–0.65) |
<0.0001 |
LOS >9 days (vs. <3 days) |
0.57 (0.42–0.78) |
<0.0001 |
ACEI at discharge |
0.51 (0.41–0.64) |
<0.0001 |
β-blockers at discharge |
0.75 (0.58–0.97) |
0.027 |
Insulin at discharge |
1.67 (1.27–2.25) |
<0.0001 |
LMWH at discharge |
1.73 (1.25–2.41) |
0.001 |
Abbreviations: BMI, body mass index, CI, confidence interval; ECG, electrocardiogram; LMWH, low-molecular-weight heparin; LOS, length of stay; OR, odds ratio; pPCI, primary percutaneous coronary intervention; STEMI, ST-segment elevation myocardial infarction; other — see Tables 1 and 2
The best NN had 97 neurons in the hidden layer and used sinus activation and cross-entropy error functions. The prediction values for 6-month mortality after the onset of STEMI were good in both approaches. Better results were obtained in the NN approach regarding the statistical quality of the models— AUROC 0.8422 vs. 0.8137 for LR (P <0.0001). AUROC in the test groups were 0.8103 and 0.7939, respectively (P = 0.037) (Figure 2). However, the difference seems to be too small to implement the NN approach in clinical practice.
Figure 2. Receiver Operating Characteristic (ROC) Curve and area under ROC (AUROC) for Neural Network and Logistic Regression in the learning (A) and test (B) groups developed for the prediction of 6-month all-cause mortality after STEMI
DISCUSSION
In our study, a better predictive value of NN in comparison with LR was shown in terms of 6-month mortality in patients with the first anterior STEMI treated with pPCI of LAD. Nevertheless, LR had some advantages, such as a more negligible difference between learning and test groups and a possibility of interpretation of particular parameters included in the model. Moreover, the NN model is more challenging to build and does not provide data on the particular risk factor included in the analysis. To our best knowledge, it is the first study that compared these two statistical methods in STEMI patients. Hitherto, analyses comparing LR with models based on machine learning (ML), but not with NN, were conducted, and no advantage of ML over LR was shown [11–13]. The difference was not documented even though a predictive value of LR models involving more than 20 variables decreased while in ML models, it was improved [13]. Thus, it may be assumed that the predictive value of modern statistical approaches (ML, NN) might be better in analyses involving a more significant number of parameters. Another potential advantage of NN is the possibility of including other than categorical or continuous variables in the models, i.e., diagnostic images or videos [14]. The NN was examined in patients with ACS to predict the outcomes based on electronic health records. The model included all the electronic health records variables and had a similar predictive value to classical statistical methods [15]. NN’s advantages over previously used methods were documented in analyzing ST-T changes on electrocardiograms and mortality prediction on echocardiography imaging [16, 17]. The NN was utilized in analysis of magnetic resonance or coronary angiography; both approaches are still under scientific research [18–20]. Therefore, it may be concluded that the increase of data sets and the use of more complex variables, such as digital electrocardiograms or diagnostic images, may reveal the superiority of NN over the LR approach [14].
One of the primary purposes of analyses performed in the PL-ACS registry is to identify the clinically significant predictors of unfavorable events and patients with high mortality risk [21–23]. The post-STEMI risk stratification is an important clinical issue highlighted in the “Gaps in the evidence and areas for future research” section of the European Society of Cardiology guidelines for the management of acute myocardial infarction in patients presenting with ST-segment elevation [24]. In the same document, the Global Registry of Acute Coronary Events (GRACE) risk score was recommended for risk assessment and adjustment without the class of recommendation [24]. Other risk scales mentioned in the guidelines were TIMI-STEMI, Zwolle, and PAMI-II. Although they might help distinguish between low- and high-risk individuals, they were developed for patients managed in an old-fashioned way. The TIMI risk scale was worked out for patients treated with thrombolysis. Among patients included in the Zwolle risk scale development, only about half had a stent implanted during coronary angioplasty, while in the PAMI-II cohort, only balloon angioplasty was performed without stent implantation [25–27].
Moreover, it may be assumed that patients with STEMI and NSTEMI differ in many aspects, including pathophysiology, baseline characteristics of therapeutic management, and survival [24, 28]. For that reason, it seems to be reasonable to develop separate risk scores for STEMI and NSTEMI. Besides, baseline characteristics and therapeutic methods in STEMI/NSTEMI patients have changed, so risk scales should be modified and updated in the most recent population.
In our study, patients who died in the 6-month follow-up after STEMI were older than survivors, had a higher heart rate and the Killip class, lower blood pressure and ejection fraction, more often had diabetes, heart failure, and chronic kidney disease. However, they less often received optimal treatment at discharge. When patients with worse clinical characteristics are treated with lower intensity, this relation has been described and is known as the treatment-risk paradox [29]. It is not proof of the cause-and-effect relation, and the direction of the potential relations is also not established. The worse clinical status could be a contraindication for the use of ACEI/ARB or β-blockers (cardiogenic shock or hypotension). On the other hand, the link between mortality and lower doses of statin or ASA use could not be excluded. Another possible explanation could be postponing therapy due to a planned transfer to another unit or hospital. The protective effect of ACE-I and β-blocker administration at discharge should be highlighted (multivariate LR analysis results are shown in Table 3).
Study strengths and limitations
Among the advantages, a large sample of patients, whole-country coverage, and the registry’s prospective design may be mentioned. Moreover, the data were collected directly from the hospitals, and complete follow-up was obtained.
The study also has some limitations. As no data on cardiovascular mortality was available, all-cause mortality was used. Secondly, there is no information on biochemical parameters, such as complete blood count or creatinine concentration. Moreover, no data on post-discharge treatment and environmental factors, such as compliance, were available. Thirdly, we could not gather information on whether the patient was discharged home or transferred to another hospital, which may have lowered the in-hospital mortality and increased mortality after discharge. However, these limitations affect both LR and NN approaches in the same way and probably are not relevant in comparing these two statistical methods. The most relevant limitation in terms of clinical implications is a restriction in inclusion criteria. The study included only STEMI patients treated with pPCI of LAD.
CONCLUSIONS
To conclude, the neural network may have a better predictive value than logistic regression in patients after the first STEMI treated with primary percutaneous coronary intervention in the left anterior descending artery. However, the statistical difference is slight and may not be relevant from the clinical point of view. Further studies on larger data sets containing different data types are required to verify NN’s clinical usefulness in the prediction of death risk after myocardial infarction.
Article information
Conflict of interest: None declared.
Open access: This article is available in open access under Creative Common Attribution-Non-Commercial-No Derivatives 4.0 International (CC BY-NC-ND 4.0) license, allowing to download articles and share them with others as long as they credit the authors and the publisher, but without permission to change them in any way or use them commercially. For commercial use, please contact the journal office at kardiologiapolska@ptkardio.pl.
How to cite: Niedziela JT, Cieśla D, Wojakowski W, et al. Is neural network better than logistic regression in death prediction in patients after ST-segment elevation myocardial infarction? Kardiol Pol. 2021; 79(12): 1353–1361, doi: 10.33963/KP.a2021.0142.
REFERENCES
- 1. Timmis A, Townsend N, Gale C, et al. European Society of Cardiology: cardiovascular disease statistics 2017. Eur Heart J. 2018; 39(7): 508–579, doi: 10.1093/eurheartj/ehx628, indexed in Pubmed: 29190377.
- 2. Doost Hosseiny A, Moloi S, Chandrasekhar J, et al. Mortality pattern and cause of death in a long-term follow-up of patients with STEMI treated with primary PCI. Open Heart. 2016; 3(1): e000405, doi: 10.1136/openhrt-2016-000405, indexed in Pubmed: 27099764.
- 3. Pedersen F, Butrymovich V, Kelbæk H, et al. Short- and long-term cause of death in patients treated with primary PCI for STEMI. J Am Coll Cardiol. 2014; 64(20): 2101–2108, doi: 10.1016/j.jacc.2014.08.037, indexed in Pubmed: 25457398.
- 4. Wojtyniak B, Gierlotka M, Opolski G, et al. Observed and relative survival and 5-year outcomes of patients discharged after acute myocardial infarction: the nationwide AMI-PL database. Kardiol Pol. 2020; 78(10): 990–998, doi: 10.33963/kp.15465, indexed in Pubmed: 32631026.
- 5. Jernberg T. Swedeheart Annual Report 2017. Stockholm, 2018.
- 6. Polańska-Skrzypczyk M, Karcz M, Rużyłło W, et al. Bedside prediction of 9-year mortality after STsegment elevation myocardial infarction treated with primary percutaneous coronary intervention. Kardiol Pol. 2019; 77(7-8): 703–709, doi: 10.33963/KP.14892, indexed in Pubmed: 31290481.
- 7. Hinton G. Deep learning — a technology with the potential to transform health care. JAMA. 2018; 320(11): 1101–1102, doi: 10.1001/jama.2018.11100, indexed in Pubmed: 30178065.
- 8. Poloński L, Gasior M, Gierlotka M, et al. Polish Registry of Acute Coronary Syndromes (PL-ACS). Characteristics, treatments and outcomes of patients with acute coronary syndromes in Poland. Kardiol Pol. 2007; 65(8): 861–874, indexed in Pubmed: 17853315.
- 9. Kocayigit I, Yaylaci S, Osken A, et al. Comparison of effects of thrombolytic therapy and primary percutaneous coronary intervention in elderly patients with acute ST-segment elevation myocardial infarction on in-hospital, six-month, and one-year mortality. Arch Med Sci Atheroscler Dis. 2019; 4: e82–e88, doi: 10.5114/amsad.2019.85378, indexed in Pubmed: 31211274.
- 10. Hanley JA, McNeil BJ. The meaning and use of the area under a receiver operating characteristic (ROC) curve. Radiology. 1982; 143(1): 29–36, doi: 10.1148/radiology.143.1.7063747, indexed in Pubmed: 7063747.
- 11. Wallert J, Tomasoni M, Madison G, et al. Predicting two-year survival versus non-survival after first myocardial infarction using machine learning and Swedish national register data. BMC Med Inform Decis Mak. 2017; 17(1): 99, doi: 10.1186/s12911-017-0500-y, indexed in Pubmed: 28679442.
- 12. Mansoor H, Elgendy IY, Segal R, et al. Risk prediction model for in-hospital mortality in women with ST-elevation myocardial infarction: a machine learning approach. Heart Lung. 2017; 46(6): 405–411, doi: 10.1016/j.hrtlng.2017.09.003, indexed in Pubmed: 28992993.
- 13. Shouval R, Hadanny A, Shlomo N, et al. Machine learning for prediction of 30-day mortality after ST elevation myocardial infraction: an Acute Coronary Syndrome Israeli Survey data mining study. Int J Cardiol. 2017; 246: 7–13, doi: 10.1016/j.ijcard.2017.05.067, indexed in Pubmed: 28867023.
- 14. Naylor CD. On the prospects for a (deep) learning health care system. JAMA. 2018; 320(11): 1099–1100, doi: 10.1001/jama.2018.11103, indexed in Pubmed: 30178068.
- 15. Huang Z, Dong W, Duan H, et al. A regularized deep learning approach for clinical risk prediction of acute coronary syndrome using electronic health records. IEEE Trans Biomed Eng. 2018; 65(5): 956–968, doi: 10.1109/TBME.2017.2731158, indexed in Pubmed: 28742027.
- 16. Xiao R, Xu Y, Pelter MM, et al. A deep learning approach to examine ischemic ST changes in ambulatory ECG recordings. AMIA Jt Summits Transl Sci Proc. 2018; 2017: 256–262, indexed in Pubmed: 29888083.
- 17. Kwon JM, Kim KH, Jeon KH, et al. Deep learning for predicting in-hospital mortality among heart disease patients based on echocardiography. Echocardiography. 2019; 36(2): 213–218, doi: 10.1111/echo.14220, indexed in Pubmed: 30515886.
- 18. Zreik M, van Hamersvelt RW, Wolterink JM, et al. A recurrent CNN for automatic detection and classification of coronary artery plaque and stenosis in coronary CT angiography. IEEE Trans Med Imaging. 2019; 38(7): 1588–1598, doi: 10.1109/TMI.2018.2883807, indexed in Pubmed: 30507498.
- 19. Zreik M, Lessmann N, van Hamersvelt RW, et al. Deep learning analysis of the myocardium in coronary CT angiography for identification of patients with functionally significant coronary artery stenosis. Med Image Anal. 2018; 44: 72–85, doi: 10.1016/j.media.2017.11.008, indexed in Pubmed: 29197253.
- 20. Avendi MR, Kheradvar A, Jafarkhani H, et al. A combined deep-learning and deformable-model approach to fully automatic segmentation of the left ventricle in cardiac MRI. Med Image Anal. 2016; 30(6): 108–119, doi: 10.1016/j.media.2016.01.005, indexed in Pubmed: 26917105.
- 21. Piątek Ł, Wilczek K, Kurzawski J, et al. Gender-related disparities in the treatment and outcomes in patients with non-ST-segment elevation myocardial infarction: results from the Polish Registry of Acute Coronary Syndromes (PL-ACS) in the years 2012–2014. Arch Med Sci. 2020; 16(4): 781–788, doi: 10.5114/aoms.2018.76112, indexed in Pubmed: 32542078.
- 22. Zandecki L, Janion M, Sadowski M, et al. Associations of changes in patient characteristics and management with decrease in mortality rates of men and women with ST-elevation myocardial infarction — a propensity score-matched analysis. Arch Med Sci. 2020; 16(4): 772–780, doi: 10.5114/aoms.2020.93458, indexed in Pubmed: 32542077.
- 23. Trzeciak P, Sierpiński R, Niedziela J, et al. Comparison of clinical characteristics, treatment, in-hospital and 12-month outcomes in patients after myocardial infarction with ejection fraction <40% with or without atrial fibrillation. Arch Med Sci. 2020, doi: 10.5114/aoms.2020.100366.
- 24. Ibanez B, James S, Agewall S, et al. ESC Scientific Document Group. 2017 ESC Guidelines for the management of acute myocardial infarction in patients presenting with ST-segment elevation: The Task Force for the management of acute myocardial infarction in patients presenting with ST-segment elevation of the European Society of Cardiology (ESC). Eur Heart J. 2018; 39(2): 119–177, doi: 10.1093/eurheartj/ehx393, indexed in Pubmed: 28886621.
- 25. Morrow DA, Antman EM, Charlesworth A, et al. TIMI risk score for st-elevation myocardial infarction: a convenient, bedside, clinical score for risk assessment at presentation: an intravenous nPA for treatment of infarcting myocardium early II trial substudy. Circulation. 2000; 102(17): 2031–2037, doi: 10.1161/01.cir.102.17.2031, indexed in Pubmed: 11044416.
- 26. Luca GDe, Suryapranata H, Hof Av, et al. Prognostic assessment of patients with acute myocardial infarction treated with primary angioplasty. Circulation. 2004; 109(22): 2737–2743, doi: 10.1161/01.cir.0000131765.73959.87.
- 27. Grines CL, Marsalese DL, Brodie B, et al. Safety and cost-effectiveness of early discharge after primary angioplasty in low risk patients with acute myocardial infarction . J Am Coll Cardiol. 1998; 31(5): 967–972, doi: 10.1016/s0735-1097(98)00031-x, indexed in Pubmed: 9561995.
- 28. Collet JP, Roffi M, Mueller C, et al. ESC Scientific Document Group. 2015 ESC Guidelines for the management of acute coronary syndromes in patients presenting without persistent ST-segment elevation: Task Force for the Management of Acute Coronary Syndromes in Patients Presenting without Persistent ST-Segment Elevation of the European Society of Cardiology (ESC). Eur Heart J. 2016; 37(3): 267–315, doi: 10.1093/eurheartj/ehv320, indexed in Pubmed: 26320110.
- 29. Roffi M, Mukherjee D. Treatment-risk paradox in acute coronary syndromes. Eur Heart J. 2018; 39(42): 3807–3809, doi: 10.1093/eurheartj/ehy577, indexed in Pubmed: 30247536.
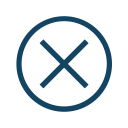