How automated image analysis techniques help scientists in species identification and classification?
Abstract
Identification of taxonomy at a specific level is time consuming and reliant upon expert ecologists. Hence the demand for automated species identification increased over the last two decades. Automation of data classification is primarily focussed on images while incorporating and analysing image data has recently become easier due to developments in computational technology. Research efforts on identification of species include specimens’ image processing, extraction of identical features, followed by classifying them into correct categories. In this paper, we discuss recent automated species identification systems, mainly for categorising and evaluating their methods. We reviewed and compared different methods in step by step scheme of automated identification and classification systems of species images. The selection of methods is influenced by many variables such as level of classification, number of training data and complexity of images. The aim of writing this paper is to provide researchers and scientists an extensive background study on work related to automated species identification, focusing on pattern recognition techniques in building such systems for biodiversity studies. (Folia Morphol 2018; 77, 2: 179–193)
Keywords: automated image recognitiondigital image processingspecies imagesspecies classificationlife data technology
References
- Abu A, Lim S, Sidhu A, et al. Biodiversity image retrieval framework for monogeneans. Syst Biodivers. 2013; 11(1): 19–33.
- Ahmed F, Kabir MH, Bhuyan S, et al. Automated weed classification with local pattern-based texture descriptors. Int Arab J Inf Technol. 2014; 11: 87–94.
- Ali R, Hussain A, Bron J, et al. Multi-stage classification of Gyrodactylus species using machine learning and feature selection techniques. IEEE. 2011: 457–462.
- Ali R, Hussain A, Bron JE, Shinn AP: The Use of ASM Feature Extraction and Machine Learning for the Discrimination of Members of the Fish Ectoparasite Genus Gyrodactylus; in Huang T, Zeng Z, Li C, Leung CS (eds): Neural Information Processing. Springer Berlin Heidelberg, 2012, [cited 2016 Sep 30], pp 256–263.
- Anton A, Duthie H. Use of cluster analysis in the systematics of the algal genus Cryptomonas. Can J Bot. 1981; 59(6): 992–1002.
- Arbuckle T, Schröder S, Steinhage V, Wittmann D: Biodiversity Informatics in Action: Identification and Monitoring of Bee Species Using ABIS 2001.
- Arce SH, Wu PH, Tseng Y. Fast and accurate automated cell boundary determination for fluorescence microscopy. Sci Rep. 2013; 3: 2266.
- Avci D, Varol A. An expert diagnosis system for classification of human parasite eggs based on multi-class SVM. Expert Syst Appl. 2009; 36(1): 43–48.
- Benfield M, Grosjean P, Culverhouse P, et al. RAPID: research on automated plankton identification. Oceanography. 2007; 20(2): 172–187.
- Bovik AC, Huang TS, Munson DC. The effect of median filtering on edge estimation and detection. IEEE Trans Pattern Anal Mach Intell. 1987; 9(2): 181–194.
- Bradbury MH, Bracegirdle DB. Introduction to Light Microscopy. 2 ed. Oxford, Garland Science. 1998.
- Bunte K, Biehl M, Jonkman M, et al. Learning effective color features for content based image retrieval in dermatology. Pattern Recognition. 2011; 44(9): 1892–1902.
- Canny J. A Computational Approach to Edge Detection. IEEE Trans Pattern Anal Mach Intell. 1986; PAMI-8(6): 679–698.
- Castañón C, Fraga J, Fernandez S, et al. Biological shape characterization for automatic image recognition and diagnosis of protozoan parasites of the genus Eimeria. Pattern Recognition. 2007; 40(7): 1899–1910.
- Cho J, Choi J, Qiao, et al. Automatic Identification of Tobacco Whiteflies, Aphids and Thrips in Greenhouse Using Image Processing TechniquesMexico, 2008, [cited 2016 Sep 30], pp 46–53.
- Choras RS: Image feature extraction techniques and their applications for CBIR and biometrics systems. International journal of biology and biomedical engineering 2007; 1: 6–16.
- Coltelli P, Barsanti L, Evangelista V, et al. Water monitoring: automated and real time identification and classification of algae using digital microscopy. Environ Sci Process Impacts. 2014; 16(11): 2656–2665.
- Culverhouse PF, Williams R, Reguera B, et al. Do experts make mistakes? A comparison of human and machine identification of dinoflagellates. Mar Ecol Prog Ser. 2003; 247: 17–25.
- Dalal N, Triggs B: Histograms of Oriented Gradients for Human Detection; in : Proceedings of the 2005 IEEE Computer Society Conference on Computer Vision and Pattern Recognition (CVPR’05) - Volume 1 - Volume 01. Washington, DC, USA, IEEE Computer Society, 2005, pp 886–893.
- Ruberto CDi, Dempster A, Khan S, et al. Automatic thresholding of infected blood images using granulometry and regional extrema. IEEE Comput. Soc. 2000: 441–444.
- Dietrich CH, Pooley CD. Automated Identification of Leafhoppers (Homoptera: Cicadellidae: Draeculacephala Ball). Ann Entomol Soc Am. 1994; 87(4): 412–423.
- Doncic A, Eser U, Atay O, et al. An algorithm to automate yeast segmentation and tracking. PLoS One. 2013; 8(3): e57970.
- Duda RO, Hart PE, Stork DG. Pattern Classification. John Wiley & Sons. 2012.
- Feng L, Bhanu B, Heraty J. A software system for automated identification and retrieval of moth images based on wing attributes. Pattern Recognition. 2016; 51: 225–241.
- Feng L, Bhanu B. Automated identification and retrieval of moth images with semantically related visual attributes on the wings. IEEE. 2013: 2577–2581.
- Flusser J, Suk T. Pattern recognition by affine moment invariants. Pattern Recognition. 1993; 26(1): 167–174.
- Francoy T, Wittmann D, Drauschke M, et al. Identification of Africanized honey bees through wing morphometrics: two fast and efficient procedures. Apidologie. 2008; 39(5): 488–494.
- Gaston KJ, O'Neill MA. Automated species identification: why not? Philos Trans R Soc Lond B Biol Sci. 2004; 359(1444): 655–667.
- Gomez A, Salazar A: Towards Automatic Wild Animal Monitoring: Identification of Animal Species in Camera-trap Images using Very Deep Convolutional Neural Networks. ArXiv Prepr ArXiv160306169 2016. http://arxiv.org/abs/1603.06169 (cited 2016 Oct 2).
- Gonzalez RC, Woods RE. Digital Image Processing. 3 edition Upper Saddle River. N.J. Pearson. 2007.
- Grigorescu SE, Petkov N, Kruizinga P. Comparison of texture features based on Gabor filters. IEEE Trans Image Process. 2002; 11(10): 1160–1167.
- Hanqing Z, Zuorui S, University YXA, (China) B, Protection) C of P: On computer-aided insect identification through math-morphology features. Zhonggou Nongye Daxue Xuebao China 2002. http://agris.fao.org/agris-search/search.do?recordID=CN2003080710 (cited 2016 Sep 30).
- Haralick R, Shanmugam K, Dinstein I. Textural Features for Image Classification. IEEE Trans Syst Man Cybern. 1973; SMC-3(6): 610–621.
- Haralick RM, Shapiro LG. Computer and Robot Vision. ed 1st Boston, MA, USA, Addison-Wesley Longman Publishing Co. , Inc. 1992.
- Hernández-Serna A, Jiménez-Segura LF. Automatic identification of species with neural networks. Peer J. 2014; 2: e563.
- Huddar SR, Gowri S, Keerthana K, et al. Novel algorithm for segmentation and automatic identification of pests on plants using image processing. IEEE. 2012: 1–5.
- Islam M, Dengsheng Z, Guojun L. A geometric method to compute directionality features for texture images. IEEE. 2008: 1521–1524.
- Jain A, Zongker D. Feature selection: evaluation, application, and small sample performance. IEEE Trans Pattern Anal Mach Intell. 1997; 19(2): 153–158.
- Jain AK, Duin PW. Statistical pattern recognition: a review. IEEE Trans Pattern Anal Mach Intell. 2000; 22(1): 4–37.
- Jalba A, Wilkinson M, Roerdink J, et al. Automatic diatom identification using contour analysis by morphological curvature scale spaces. Mach Vis Appl. 2005; 16(4): 217–228.
- Jimenez L, Landgrebe D. High dimensional feature reduction via projection pursuit. ECE Tech Rep. 1996: 103.
- Jin T, Hou X, Li P, et al. A novel method of automatic plant species identification using sparse representation of leaf tooth features. PLoS One. 2015; 10(10): e0139482.
- Jolliffe I: Principal component analysis. Wiley Online Library, 2002. http://onlinelibrary.wiley.com/doi/10.1002/9781118445112.stat06472/full (cited 2016 Oct 3).
- Jonker R, Groben R, Tarran G, et al. Automated identification and characterisation of microbial populations using flow cytometry: the AIMS project. Scientia Marina. 2000; 64(2): 225–234.
- Kalafi EY, Tan WB, Town C, et al. Automated identification of Monogeneans using digital image processing and K-nearest neighbour approaches. BMC Bioinformatics. 2016; 17(Suppl 19): 511.
- Kang WX, Yang QQ, Liang RP. The Comparative Research on Image Segmentation Algorithms. IEEE. 2009: 703–707.
- Karagiannopoulos M, Anyfantis D, Kotsiantis SB, Pintelas PE: Feature selection for regression problems; in : Proceedings of the 8th Hellenic European Research on Computer Mathematics & its Applications, Athens, Greece. 2007. http://www.math.upatras.gr/~dany/Downloads/hercma07.pdf (cited 2016 Oct 5).
- Kay JW, Shinn AP, Sommerville C. Towards an automated system for the identification of notifiable pathogens: using Gyrodactylus salaris as an example. Parasitol Today. 1999; 15(5): 201–206.
- Kaya Y, Kayci L, Uyar M. Automatic identification of butterfly species based on local binary patterns and artificial neural network. Applied Soft Comput. 2015; 28: 132–137.
- Khan W. Image Segmentation Techniques: A Survey. J Image Graph. 2014: 166–170.
- Kiranyaz S, Ince T, Pulkkinen J, et al. Classification and retrieval on macroinvertebrate image databases. Comput Biol Med. 2011; 41(7): 463–472.
- Kudo M, Sklansky J. Comparison of algorithms that select features for pattern classifiers. Pattern Recognition. 2000; 33(1): 25–41.
- Larios N, Deng H, Zhang W, et al. Automated insect identification through concatenated histograms of local appearance features: feature vector generation and region detection for deformable objects. Mach Vis Appl. 2007; 19(2): 105–123.
- Lei Z, Liao S, Li SZ: Efficient feature selection for linear discriminant analysis and its application to face recognition. IEEE, 2012, [cited 2016 Oct 2], pp 1136–1139.
- Leow LK, Chew LL, Chong VC, et al. Automated identification of copepods using digital image processing and artificial neural network. BMC Bioinformatics. 2015; 16 Suppl 18: S4.
- Le-Qing Z, Zhen Z. Automatic insect classification based on local mean colour feature and Supported Vector Machines. Oriental Insects. 2012; 46(3-4): 260–269.
- Li J, Tseng KK, Hsieh ZuYi, et al. Staining pattern classification of antinuclear autoantibodies based on block segmentation in indirect immunofluorescence images. PLoS One. 2014; 9(12): e113132.
- Liu F, Shen ZR, Zhang JW, et al. Automatic insect identification based on color characters. Chin Bull Entomol. 2008; 45: 150–153.
- Loke KS, Egerton S, Cristofaro D, Clementson S: Automated real-time dynamic identification of flying and resting butterfly species in the natural environment; in : 2011 International Conference on Environment Science and Engineering (ICESE 2011)(Saji Baby and Richard Haynes 1 April 2011 to 3 April 2011). Institute of Electrical and Electronics Engineers, 2011, [cited 2016 Oct 1], pp. 179–183.
- Loncaric S. A survey of shape analysis techniques. Pattern Recognition. 1998; 31(8): 983–1001.
- Loos A, Ernst A. An automated chimpanzee identification system using face detection and recognition. EURASIP J Image Video Process. 2013; 2013(1): 49.
- Lowe D. Distinctive Image Features from Scale-Invariant Keypoints. Int J Comput Vis. 2004; 60(2): 91–110.
- Lu An, Hou X, Lin C, et al. Insect Species Recognition using Sparse Representation. Br Machine Vision Association. 2010: 108.1–108.10.
- Luo Q, Gao Y, Luo J, et al. Automatic Identification of Diatoms with Circular Shape using Texture Analysis. J Software. 2011; 6(3).
- Mansoor H, Sorayya M, Aishah S, Mogeeb A, Mosleh A: Automatic recognition system for some cyanobacteria using image processing techniques and ANN approach; In: Int Conf on Envir and Comp Science IPCBEE. 2011, pp 73–78.
- Martins J, Oliveira LS, Nisgoski S, et al. A database for automatic classification of forest species. Mach Vis Appl. 2012; 24(3): 567–578.
- Mayo M, Watson A. Automatic species identification of live moths. Knowledge-Based Systems. 2007; 20(2): 195–202.
- Mehdipour Ghazi M, Yanikoglu B, Aptoula E. Plant identification using deep neural networks via optimization of transfer learning parameters. Neurocomputing. 2017; 235: 228–235.
- Ming-Kuei H. Visual pattern recognition by moment invariants. IEEE Transactions on Information Theory. 1962; 8(2): 179–187.
- Moyo T, Bangay S, Foster G. The identification of mammalian species through the classification of hair patterns using image pattern recognition. Proceedings of the 4th international conference on Computer graphics, virtual reality, visualisation and interaction in Africa - Afrigaph '06. 2006.
- Mythili C, Kavitha V. Efficient technique for color image noise reduction. Res Bull Jordan ACM. 2011; 1: 41–44.
- Natchimuthu S, Natchimuthu S, Chinnaraj P, et al. Automatic Identification of Algal Community from Microscopic Images. Bioinforma Biol Insights. 2013: 327.
- O’Neill MA, Gauld ID, Gaston KJ, Weeks PJD: Daisy: an automated invertebrate identification system using holistic vision techniques; in : Proceedings of the Inaugural Meeting BioNET-INTERNATIONAL Group for Computer-Aided Taxonomy (BIGCAT). 2000, pp 13–22.
- Ojala T, Pietikainen M, Maenpaa T. Multiresolution gray-scale and rotation invariant texture classification with local binary patterns. IEEE Trans Pattern Anal Mach Intell. 2002; 24(7): 971–987.
- Parisi-Baradad V, Manjabacas A, Lombarte A, et al. Automated Taxon Identification of Teleost fishes using an otolith online database: AFORO. Fisheries Res. 2010; 105(1): 13–20.
- Perre P, Faria FA, Jorge LR, et al. Toward an Automated Identification of Anastrepha Fruit Flies in the fraterculus group (Diptera, Tephritidae). Neotrop Entomol. 2016; 45(5): 554–558.
- Phil C, Simpson RG, Rob E, et al. Automatic classification of field-collected dinoflagellates by artificial neural network. Mar Ecol Prog Ser. 1996; 139: 281–287.
- Ping Tian D. A review on image feature extraction and representation techniques. Int J Multimed Ubiquitous Eng. 2013; 8: 385–396.
- Quinlan JR: C4. 5: programs for machine learning. Elsevier, 2014. https://books.google.com/books?hl=en&lr=&id=b3ujBQAAQBAJ&oi=fnd&pg=PP1&dq=C4.+5:+programs+for+machine+learning.+Morgan+Kaufmann&ots=sP8rULDoD6&sig=Yv1JBLnQGgoc5y8JfzcwOYvBqVw (cited 2016 Oct 1).
- Quivy CH, Kumazawa I. Normalization of Active Appearance Models for Fish Species Identification. ISRN Signal Processing. 2011; 2011: 1–16.
- Ranzato M, Taylor PE, House JM, et al. Automatic recognition of biological particles in microscopic images. Pattern Recognition Lett. 2007; 28(1): 31–39.
- Riggs LA. Curvature as a feature of pattern vision. Science. 1973; 181(4104): 1070–1072.
- Rother C, Kolmogorov V, Blake A. Grabcut: Interactive foreground extraction using iterated graph cuts. ACM Transactions on Graphics (TOG). 2004; 23(3): 309–314.
- Russell KN, Do MT, Huff JC, Platnick NI, MacLeod N: Introducing SPIDA-web: wavelets, neural networks and Internet accessibility in an image-based automated identification system. Autom Taxon Identif Syst Theory Approaches Appl 2007;131–152.
- Salimi N, Loh KH, Dhillon SK, et al. Fully-automated identification of fish species based on otolith contour: using short-time Fourier transform and discriminant analysis (STFT-DA). PeerJ. 2016; 4: e1664.
- Sang-Hee L. A Novel Approach to Shape Recognition Using Shape Outline. J Korean Phys Soc. 2010; 56(3(1)): 1016–1019.
- Saraswat M, Arya KV. Automated microscopic image analysis for leukocytes identification: a survey. Micron. 2014; 65: 20–33.
- Savkare SS, Narote SP. Automated system for malaria parasite identification. IEEE. 2015: 1–4.
- Savkare SS, Narote SP. Automatic detection of malaria parasites for estimating parasitemia. Int J Comput Sci Secur IJCSS. 2011; 5: 310.
- Savkare SS, Narote SP. Automatic System for Classification of Erythrocytes Infected with Malaria and Identification of Parasite's Life Stage. Procedia Technology. 2012; 6: 405–410.
- Schroder S, Wittmann D, Drescher W, Roth V, Steinhage V, Cremers AB: The new key to bees: automated identification by image analysis of wings. Pollinating Bees– Conserv Link Agric Nat Minist Environ Bras 2002. https://www.researchgate.net/profile/Volker_Roth/publication/241318944_THE_NEW_KEY_TO_BEES_AUTOMATED_IDENTIFICATION_BY_IMAGE_ANALYSIS_OF_WINGS/links/0046352a5957f5816d000000.pdf (cited 2016 Oct 2).
- Shih TK, Huang J-Y, Wang C-S, Hung JC, Kao C-H: An intelligent content-based image retrieval system based on color, shape and spatial relations; in: Proceedings-National Science Council Republic Of China Part A Physical Science And Engineering. 2001, [cited 2016 Oct 2], pp 232–243.
- Singh HK, Tomar SK, Maurya PK. Thresholding Techniques applied for Segmentation of RGB and multispectral images. Proc Publ Int J Comput Appl. 2012: 975–8887.
- Singhai N, Shandilya S. A Survey On: “Content Based Image Retrieval Systems”. Int J Comput Appl. 2010; 4(2): 22–26.
- Soberón J, Peterson AT. Biodiversity informatics: managing and applying primary biodiversity data. Philos Trans R Soc Lond B Biol Sci. 2004; 359(1444): 689–698.
- Thiel SU, Wiltshire RJ, Davies LJ. Automated object recognition of blue-green algae for measuring water quality-a preliminary study. Oceanogr Lit Rev. 1996; 1: 85.
- Trattner S, Greenspan H, Tepper G, et al. Automatic identification of bacterial types using statistical imaging methods. IEEE Trans Med Imaging. 2004; 23(7): 807–820.
- Underwood J, Dahlberg A, FitzPatrick S, et al. A STILE Project case study: The evaluation of a computer-based visual key for fossil identification. Assoc Learn Technol J. 1996; 4(2): 40–47.
- Ververidis D, Kotropoulos C. Fast and accurate sequential floating forward feature selection with the Bayes classifier applied to speech emotion recognition. Signal Processing. 2008; 88(12): 2956–2970.
- Vogt A, Cholewinski A, Shen X, et al. Automated image-based phenotypic analysis in zebrafish embryos. Dev Dyn. 2009; 238(3): 656–663.
- Wang J, Lin C, Ji L, et al. A new automatic identification system of insect images at the order level. Knowledge-Based Systems. 2012; 33: 102–110.
- Weeks P, O'Neill MA, Gaston KJ, et al. Species–identification of wasps using principal component associative memories. Image Vis Comp. 1999; 17(12): 861–866.
- Wen C, Guyer D, Li W. Local feature-based identification and classification for orchard insects. Biosystems Eng. 2009; 104(3): 299–307.
- Witten IH, Frank E: Data Mining: Practical machine learning tools and techniques. Morgan Kaufmann, 2005. https://books.google.com/books?hl=en&lr=&id=QTnOcZJzlUoC&oi=fnd&pg=PR17&dq=Data+Mining:+Practical+machine+learning+tools+and+techniques&ots=3hnBcpZlQe&sig=jDee4hf43_u_bRcYZ7yoNzZtuZQ (cited 2016 Oct 2).
- Yang M, Kpalma K, Ronsin J. A Survey of Shape Feature Extraction Techniques. Pattern Recognition Techniques, Technology and Applications. 2008: 43–90.
- Yang YS, Park DK, Kim HC, et al. Automatic identification of human helminth eggs on microscopic fecal specimens using digital image processing and an artificial neural network. IEEE Trans Biomed Eng. 2001; 48(6): 718–730.
- Yu L, Liu H: Feature Selection for High-Dimensional Data: A Fast Correlation-Based Filter Solution; in : Proceedings of the 20th International Conference on Machine Learning (ICML-03). 2003, [cited 2016 Oct 2], pp 856–863.
- Yu X, Wang J, Kays R, et al. Automated identification of animal species in camera trap images. EURASIP J Image Video Process. 2013; 2013(1): 52.
- Yuan G, Hasler N, Klette R, Rosenhahn B: Understanding Tracks of Different Species of Rats (2006). CITR, The University of Auckland, New Zealand, 2006. https://researchspace.auckland.ac.nz/handle/2292/2783 (cited 2016 Oct 2).
- Yue J, Li Z, Liu Lu, et al. Content-based image retrieval using color and texture fused features. Math Comput Model. 2011; 54(3-4): 1121–1127.
- Zhan M, Crane MM, Entchev EV, et al. Automated Processing of Imaging Data through Multi-tiered Classification of Biological Structures Illustrated Using Caenorhabditis elegans. PLoS Comput Biol. 2015; 11(4): e1004194.
- Zhang D, Lu G. Review of shape representation and description techniques. Pattern Recognition. 2004; 37(1): 1–19.
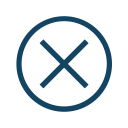