Artificial intelligence and automation in valvular heart diseases
Abstract
Artificial intelligence (AI) is gradually changing every aspect of social life, and healthcare is no exception. The clinical procedures that were supposed to, and could previously only be handled by human experts can now be carried out by machines in a more accurate and efficient way. The coming era of big data and the advent of supercomputers provides great opportunities to the development of AI technology for the enhancement of diagnosis and clinical decision-making. This review provides an introduction to AI and highlights its applications in the clinical flow of diagnosing and treating valvular heart diseases (VHDs). More specifically, this review first introduces some key concepts and subareas in AI. Secondly, it discusses the application of AI in heart sound auscultation and medical image analysis for assistance in diagnosing VHDs. Thirdly, it introduces using AI algorithms to identify risk factors and predict mortality of cardiac surgery. This review also describes the state-of-the-art autonomous surgical robots and their roles in cardiac surgery and intervention.
Keywords: artificial intelligencemachine learningvalvular heart diseasesmedical image analysistranscathether aortic valve implantation
References
- Topol EJ. High-performance medicine: the convergence of human and artificial intelligence. Nat Med. 2019; 25(1): 44–56.
- Zhang J, Gajjala S, Agrawal P, et al. Fully automated echocardiogram interpretation in clinical practice. Circulation. 2018; 138(16): 1623–1635.
- Benjamin EJ, Muntner P, Alonso A, et al. Heart Disease and Stroke Statistics — 2019 Update: A Report From the American Heart Association. Vol. 139, Circulation. 2019.
- Schwartz WB, Patil RS, Szolovits P. Artificial intelligence in medicine. Where do we stand? N Engl J Med. 1987; 316(11): 685–688.
- Shortliffe EHA. rule-based comuter rogram for advising hysicians regarding antimicrobial theray selection. In: Proceedings of the Annual ACM Conference. ACM. 1974; 739.
- Szolovits P, Pauker SG. Categorical and probabilistic reasoning in medical diagnosis. Artif Intell [Internet]. 1978 Aug 1; 11(1–2):115–44. https://www.sciencedirect.com/science/article/abs/pii/0004370278900140 (cited 2019 Aug 20).
- Beam AL, Kohane IS. Big data and machine learning in health care. JAMA. 2018; 319(13): 1317–1318.
- Ton J. Cleophas AHZ. Machine Learning in Medicine. 2013;1920–30. https://link.springer.com/content/pdf/10.1007%2F978-94-007-5824-7.pdf.
- LeCun Y, Bengio Y, Hinton G. Deep learning. Nature. 2015; 521(7553): 436–444.
- Ernande L, Audureau E, Jellis CL, et al. Clinical implications of echocardiographic phenotypes of patients with diabetes mellitus. J Am Coll Cardiol. 2017; 70(14): 1704–1716.
- Zhao Y, Zeng D, Socinski MA, et al. Reinforcement learning strategies for clinical trials in nonsmall cell lung cancer. Biometrics. 2011; 67(4): 1422–1433.
- Hinton GE, Osindero S, Teh YW. A fast learning algorithm for deep belief nets. Neural Comput. 2006; 18(7): 1527–1554.
- Chen C. Computer vision in medical imaging. Vol. 2. World Scientific. 2014.
- Puppe F. Systematic introduction to expert systems: Knowledge representations and problem-solving methods. Springer Science & Business Media. 2012.
- Tavel ME. Cardiac auscultation. A glorious past -- but does it have a future? Circulation. 1996; 93(6): 1250–1253.
- Wang A, Bashore TM. Valvular Heart Disease. 2009.
- Thompson WR, Reinisch AJ, Unterberger MJ, et al. Artificial intelligence-assisted auscultation of heart murmurs: validation by virtual clinical trial. Pediatr Cardiol. 2019; 40(3): 623–629.
- Dwivedi AK, Imtiaz SA, Rodriguez-Villegas E. Algorithms for automatic analysis and classification of heart sounds-A systematic review. IEEE Access. 2019; 7(c): 8316–45.
- Lehner RJ, Rangayyan RM. A three-channel microcomputer system for segmentation and characterization of the phonocardiogram. IEEE Trans Biomed Eng. 1987; 34(6): 485–489.
- Iwata A, Ishii N, Suzumura N, et al. Algorithm for detecting the first and the second heart sounds by spectral tracking. Med Biol Eng Comput. 1980; 18(1): 19–26.
- Liang H, Lukkarinen S, Hartimo I. Heart sound segmentation algorithm based on heart sound envelogram. 1997; 24: 105–108.
- Johnson CR, Sethares WA, Klein AG. Software Receiver Design: Build Your Own Digital Communication System in Five Easy Steps. 2011.
- Jiang Z, Choi SA. cardiac sound characteristic waveform method for in-home heart disorder monitoring with electric stethoscope. Expert Syst Appl. 2006; 31(2): 286–98.
- Sun S. An innovative intelligent system based on automatic diagnostic feature extraction for diagnosing heart diseases. Knowledge-Based Syst. 2015; 75: 224–238.
- Sun S, Jiang Z, Wang H, et al. Automatic moment segmentation and peak detection analysis of heart sound pattern via short-time modified Hilbert transform. Comput Methods Programs Biomed. 2014; 114(3): 219–230.
- Gill D, Gavrieli N, Intrator N. Detection and identification of heart sounds using homomorphic envelogram and self-organizing probabilistic model. Comput Cardiol. 2005; 32: 957–60.
- Springer DB, Tarassenko L, Clifford GD. Logistic Regression-HSMM-Based Heart Sound Segmentation. IEEE Trans Biomed Eng. 2016; 63(4): 822–832.
- Schmidt SE, Holst-Hansen C, Graff C, et al. Segmentation of heart sound recordings by a duration-dependent hidden Markov model. Physiol Meas. 2010; 31(4): 513–529.
- Potes C, Parvaneh S, Rahman A, et al. Ensemble of feature-based and deep learning-based classifiers for detection of abnormal heart sounds. Comput Cardiol. 2016; 43: 621–4.
- Chen TE, Yang SI, Ho LT, et al. S1 and S2 Heart Sound Recognition Using Deep Neural Networks. IEEE Trans Biomed Eng. 2017; 64(2): 372–380.
- Zhang W, Han J, Deng S. Heart sound classification based on scaled spectrogram and tensor decomposition. Expert Syst Appl. 2017; 84: 220–231.
- Khalid S, Khalil T, Nasreen SA. survey of feature selection and feature extraction techniques in machine learning. Proc 2014 Sci Inf Conf SAI. 2014; 2014: 372–8.
- Zhang W, Guo X, Yuan Z, et al. Heart sound classification and recognition based on eemd and correlation dimension. J Mech Med Biol. 2014; 14(04): 1450046.
- Safara F, Doraisamy S, Azman A, et al. Multi-level basis selection of wavelet packet decomposition tree for heart sound classification. Comput Biol Med. 2013; 43(10): 1407–1414.
- Kwak C. Kwon O-W. Cardiac disorder classification by heart sound signals using murmur likelihood and hidden Markov model state likelihood. IET Signal Process. 2012; 6(4): 326.
- Kumar D, Carvalho P, Antunes M, et al. Heart murmur classification with feature selection. Conf Proc IEEE Eng Med Biol Soc. 2010; 2010: 4566–4569.
- Uğuz H. A biomedical system based on artificial neural network and principal component analysis for diagnosis of the heart valve diseases. J Med Syst. 2012; 36(1): 61–72.
- Olmez T, Zumray D. Classification of heart sounds using an artificial neural network. Pattern Recognit Lett. 2003; 24: 617–629.
- Fahad HM, Ghani Khan MU, Saba T, et al. Microscopic abnormality classification of cardiac murmurs using ANFIS and HMM. Microsc Res Tech. 2018; 81(5): 449–457.
- Falk V, Baumgartner H, Bax JJ, et al. ESC/EACTS Guidelines for the management of valvular heart disease. Eur J Cardio-thoracic Surg. 2017; 52: 616–664.
- Noble JA, Boukerroui D. Ultrasound image segmentation: a survey. IEEE Trans Med Imaging. 2006; 25(8): 987–1010.
- Mikić I, Krucinski S, Thomas JD. Segmentation and tracking in echocardiographic sequences: active contours guided by optical flow estimates. IEEE Trans Med Imaging. 1998; 17(2): 274–284.
- Martin S, Daanen V, Troccaz J, et al. Tracking of the mitral valve leaflet in echocardiography images. 3rd IEEE Int Symp Biomed Imaging Nano to Macro. 2006; 2006: 181–184.
- Schneider RJ, Perrin DP, Vasilyev NV, et al. Mitral annulus segmentation from 3D ultrasound using graph cuts. IEEE Trans Med Imaging. 2010; 29(9): 1676–1687.
- Zhou X, Yang C, Yu W. Automatic mitral leaflet tracking in echocardiography by outlier detection in the low-rank representation. Proc IEEE Comput Soc Conf Comput Vis Pattern Recognit. 2012: 972–979.
- Liu X, Cheung Y, Ming Y, et al. Automatic mitral valve leaflet tracking in Echocardiography via constrained outlier pursuit and region-scalable active contours. Neurocomputing. 2014; 144: 47–57.
- Siefert AW, Icenogle DA, Rabbah JPM, et al. Accuracy of a mitral valve segmentation method using J-splines for real-time 3D echocardiography data. Ann Biomed Eng. 2013; 41(6): 1258–1268.
- Pouch AM, Wang H, Takabe M, et al. Fully automatic segmentation of the mitral leaflets in 3D transesophageal echocardiographic images using multi-atlas joint label fusion and deformable medial modeling. Med Image Anal. 2014; 18(1): 118–129.
- Ionasec RI, Voigt I, Georgescu B, et al. Patient-specific modeling and quantification of the aortic and mitral valves from 4-D cardiac CT and TEE. IEEE Trans Med Imaging. 2010; 29(9): 1636–1651.
- Costa E, Martins N, Sultan MS, et al. Mitral valve leaflets segmentation in echocardiography using convolutional neural networks. 6th IEEE Port Meet Bioeng ENBENG 2019 - Proc. 2019: 1–4.
- Shang Y, Yang X, Zhu L, et al. Region competition based active contour for medical object extraction. Comput Med Imaging Graph. 2008; 32(2): 109–117.
- Sultan MS, Martins N, Costa E, et al. Virtual M-Mode for Echocardiography: A New Approach for the Segmentation of the Anterior Mitral Leaflet. IEEE J Biomed Health Inform. 2019; 23(1): 305–313.
- Thavendiranathan P, Phelan D, Thomas JD, et al. Quantitative assessment of mitral regurgitation: validation of new methods. J Am Coll Cardiol. 2012; 60(16): 1470–1483.
- de Agustín JA, Marcos-Alberca P, Fernandez-Golfin C, et al. Direct measurement of proximal isovelocity surface area by single-beat three-dimensional color Doppler echocardiography in mitral regurgitation: a validation study. J Am Soc Echocardiogr. 2012; 25(8): 815–823.
- Grady L, Datta S, Kutter O, et al. Regurgitation Quantification Using 3D PISA in Volume Echocardiograhy. In: Fichtinger G, editors. pMedical Image Comuting and Comuter-AssisteIntervention -- MICCAI 2011. Berlin, Heidelberg: Sringer Berlin Heidelberg. 2011: 512–519.
- Cobey FC, McInnis JA, Gelfand BJ, et al. A method for automating 3-dimensional proximal isovelocity surface area measurement. J Cardiothorac Vasc Anesth. 2012; 26(3): 507–511.
- Wang Y, Georgescu B, Datta S, et al. Automatic cardiac flow quantification on 3D volume color Doppler data. Proc - Int Symp Biomed Imaging. 2011; C(Lv): 1688–1691.
- Mediratta A, Addetia K, Medvedofsky D, et al. 3D echocardiographic analysis of aortic annulus for transcatheter aortic valve replacement using novel aortic valve quantification software: Comparison with computed tomography. Echocardiography. 2017; 34(5): 690–699.
- Aquila I, Fernández-Golfín C, Rincon LM, et al. Fully automated software for mitral annulus evaluation in chronic mitral regurgitation by 3-dimensional transesophageal echocardiography. Medicine (Baltimore). 2016; 95(49): e5387.
- Kagiyama N, Toki M, Hara M, et al. Efficacy and Accuracy of Novel Automated Mitral Valve Quantification: Three-Dimensional Transesophageal Echocardiographic Study. Echocardiography. 2016; 33(5): 756–763.
- Calleja A, Thavendiranathan P, Ionasec RI, et al. Automated quantitative 3-dimensional modeling of the aortic valve and root by 3-dimensional transesophageal echocardiography in normals, aortic regurgitation, and aortic stenosis: comparison to computed tomography in normals and clinical implications. Circ Cardiovasc Imaging. 2013; 6(1): 99–108.
- de Agustin JA, Viliani D, Vieira C, et al. Proximal isovelocity surface area by single-beat three-dimensional color Doppler echocardiography applied for tricuspid regurgitation quantification. J Am Soc Echocardiogr. 2013; 26(9): 1063–1072.
- Choi J, Hong GR, Kim M, et al. Automatic quantification of aortic regurgitation using 3D full volume color doppler echocardiography: a validation study with cardiac magnetic resonance imaging. Int J Cardiovasc Imaging. 2015; 31(7): 1379–1389.
- Thavendiranathan P, Liu S, Datta S, et al. Quantification of chronic functional mitral regurgitation by automated 3-dimensional peak and integrated proximal isovelocity surface area and stroke volume techniques using real-time 3-dimensional volume color doppler echocardiography: In vitro and clini. Circ Cardiovasc Imaging. 2013; 6(1): 125–133.
- Ionasec RI, Georgescu B, Gassner E, et al. Dynamic Model-Driven Quantitative and Visual Evaluation of the Aortic Valve from 4D CT. In: Metaxas D, editors. Medical Image Comuting and Comuter-Assisted Intervention. MICCAI 2008. Berlin, Heidelberg: Sringer Berlin Heidelberg. 2008: 686–694.
- Swee JKY, Grbić S. Advanced transcatheter aortic valve implantation (TAVI) planning from CT with ShapeForest. Lect Notes Comput Sci. (including Subser Lect Notes Artif Intell Lect Notes Bioinformatics). 2014; 8674 LNCS(Part 2): 17–24.
- Waechter I, Kneser R, Korosoglou G, et al. Patient Secific Models for Planning and Guidance of Minimally Invasive Aortic Valve Imlantation. In: Jiang T, Navab N, Pluim JPW, Viergever MA, editors. Medical Image Comuting and Comuter-Assisted Intervention -- MICCAI 2010. . Berlin, Heidelberg: Sringer Berlin Heidelberg. 2010: 526–533.
- Grbic S, Ionasec R, Vitanovski D, et al. Complete valvular heart apparatus model from 4D cardiac CT. Med Image Anal. 2012; 16(5): 1003–1014.
- Grbic S, Ionasec R, Mansi T, et al. Advanced intervention planning for Transcatheter Aortic Valve Implantations (TAVI) from CT using volumetric models. Proc - Int Symp Biomed Imaging. 2013: 1424–1427.
- Koos R, Mahnken AH, Dohmen G, et al. Association of aortic valve calcification severity with the degree of aortic regurgitation after transcatheter aortic valve implantation. Int J Cardiol. 2011; 150(2): 142–145.
- Samim M, Stella PR, Agostoni P, et al. Automated 3D analysis of pre-procedural MDCT to predict annulus plane angulation and C-arm positioning: benefit on procedural outcome in patients referred for TAVR. JACC Cardiovasc Imaging. 2013; 6(2): 238–248.
- Zheng Y, John M, Liao R, et al. Automatic aorta segmentation and valve landmark detection in C-arm CT for transcatheter aortic valve implantation. IEEE Trans Med Imaging. 2012; 31(12): 2307–2321.
- Elattar M, Wiegerinck E, van Kesteren F, et al. Automatic aortic root landmark detection in CTA images for preprocedural planning of transcatheter aortic valve implantation. Int J Cardiovasc Imaging. 2016; 32(3): 501–511.
- Elattar MA, Wiegerinck EM, Planken RN, et al. Automatic segmentation of the aortic root in CT angiography of candidate patients for transcatheter aortic valve implantation. Med Biol Eng Comput. 2014; 52(7): 611–618.
- Lalys F, Esneault S, Castro M, et al. Automatic aortic root segmentation and anatomical landmarks detection for TAVI procedure planning. Minim Invasive Ther Allied Technol. 2019; 28(3): 157–164.
- Al WA, Jung HoY, Yun IlD, et al. Automatic aortic valve landmark localization in coronary CT angiography using colonial walk. PLoS One. 2018; 13(7): e0200317.
- Watanabe Y, Morice MC, Bouvier E, et al. Automated 3-dimensional aortic annular assessment by multidetector computed tomography in transcatheter aortic valve implantation. JACC Cardiovasc Interv. 2013; 6(9): 955–964.
- Delgado V, Ng ACT, Schuijf JD, et al. Automated assessment of the aortic root dimensions with multidetector row computed tomography. Ann Thorac Surg. 2011; 91(3): 716–723.
- Dankerl P, Hammon M, Seuss H, et al. Computer-aided evaluation of low-dose and low-contrast agent third-generation dual-source CT angiography prior to transcatheter aortic valve implantation (TAVI). Int J Comput Assist Radiol Surg. 2017; 12(5): 795–802.
- Guez D, Boroumand G, Ruggiero NJ, et al. Automated and Manual Measurements of the Aortic Annulus with ECG-Gated Cardiac CT Angiography Prior to Transcatheter Aortic Valve Replacement: Comparison with 3D-Transesophageal Echocardiography. Acad Radiol. 2017; 24(5): 587–593.
- Baeßler B, Mauri V, Bunck AC, et al. Software-automated multidetector computed tomography-based prosthesis-sizing in transcatheter aortic valve replacement: Inter-vendor comparison and relation to patient outcome. Int J Cardiol. 2018; 272: 267–272.
- Pennell DJ Cardiovascular magnetic resonance Circulation. 2010; 121(5): 692–705.
- Bernard O, Cervenansky F, Lalande A, et al. Deep learning techniques for automatic MRI cardiac multi-structures segmentation and diagnosis: is the problem solved? IEEE Trans Med Imaging. 2018; 37(11): 2514–2525.
- Petitjean C, Dacher JN. A review of segmentation methods in short axis cardiac MR images. Med Image Anal. 2011; 15(2): 169–184.
- Backhaus SJ, Staab W, Steinmetz M, et al. Fully automated quantification of biventricular volumes and function in cardiovascular magnetic resonance: applicability to clinical routine settings. J Cardiovasc Magn Reson. 2019; 21(1): 24.
- Fries JA, Varma P, Chen VS, et al. Weakly supervised classification of rare aortic valve malformations using unlabeled cardiac MRI sequences. bioRxiv. 2018; 2019: 1–25.
- Nashef SAM, Roques F, Sharples LD, et al. EuroSCORE II. Eur J Cardiothorac Surg. 2012; 41(4): 734–44; discussion 744.
- Nilsson J, Ohlsson M, Thulin L, et al. Risk factor identification and mortality prediction in cardiac surgery using artificial neural networks. J Thorac Cardiovasc Surg. 2006; 132(1): 12–19.
- Celi LA, Galvin S, Davidzon G, et al. A database-driven decision support system: customized mortality prediction. J Pers Med. 2012; 2(4): 138–148.
- Allyn J, Allou N, Augustin P, et al. A Comparison of a Machine Learning Model with EuroSCORE II in Predicting Mortality after Elective Cardiac Surgery: A Decision Curve Analysis. PLoS One. 2017; 12(1): e0169772.
- Hernandez-Suarez DF, Kim Y, Villablanca P, et al. Machine Learning Prediction Models for In-Hospital Mortality After Transcatheter Aortic Valve Replacement. JACC Cardiovasc Interv. 2019; 12(14): 1328–1338.
- LaFaro RJ, Pothula S, Kubal KP, et al. Neural Network Prediction of ICU Length of Stay Following Cardiac Surgery Based on Pre-Incision Variables. PLoS One. 2015; 10(12): e0145395.
- Thottakkara P, Ozrazgat-Baslanti T, Hupf BB, et al. Application of Machine Learning Techniques to High-Dimensional Clinical Data to Forecast Postoperative Complications. PLoS One. 2016; 11(5): e0155705.
- Nilsson J, Ohlsson M, Höglund P, et al. The International Heart Transplant Survival Algorithm (IHTSA): a new model to improve organ sharing and survival. PLoS One. 2015; 10(3): e0118644.
- Moustris GP, Hiridis SC, Deliparaschos KM, et al. Evolution of autonomous and semi-autonomous robotic surgical systems: a review of the literature. Int J Med Robot. 2011; 7(4): 375–392.
- Grespan L, Fiorini P, Colucci G. Looking Ahead: The Future of Robotic Surgery. In: The Route to Patient Safety in Robotic Surgery [Internet]. Cham: Springer International Publishing. 2019: 157–162.
- Panesar S, Cagle Y, Chander D, et al. Artificial Intelligence and the Future of Surgical Robotics. Ann Surg. 2019; 270(2): 223–226.
- Garcia-Ruiz A, Gagner M, Miller JH, et al. Manual vs robotically assisted laparoscopic surgery in the performance of basic manipulation and suturing tasks. Arch Surg. 1998; 133(9): 957–961.
- Mayer H, Gomez F, Wierstra D, et al. system for robotic heart surgery that learns to tie knots using recurrent neural networks. Adv Robot. 2008; 13(14): 1521–1537.
- Fagogenis G, Mencattelli M, Machaidze Z, et al. Autonomous Robotic Intracardiac Catheter Navigation Using Haptic Vision. Sci Robot. 2019; 4(29).
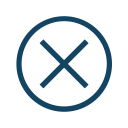