Advancements in artificial intelligence-driven techniques for interventional cardiology
Abstract
This paper aims to thoroughly discuss the impact of artificial intelligence (AI) on clinical practice in interventional cardiology (IC) with special recognition of its most recent advancements. Thus, recent years have been exceptionally abundant in advancements in computational tools, including the development of AI. The application of AI development is currently in its early stages, nevertheless new technologies have proven to be a promising concept, particularly considering IC showing great impact on patient safety, risk stratification and outcomes during the whole therapeutic process. The primary goal is to achieve the integration of multiple cardiac imaging modalities, establish online decision support systems and platforms based on augmented and/or virtual realities, and finally to create automatic medical systems, providing electronic health data on patients. In a simplified way, two main areas of AI utilization in IC may be distinguished, namely, virtual and physical. Consequently, numerous studies have provided data regarding AI utilization in terms of automated interpretation and analysis from various cardiac modalities, including electrocardiogram, echocardiography, angiography, cardiac magnetic resonance imaging, and computed tomography as well as data collected during robotic-assisted percutaneous coronary intervention procedures. Thus, this paper aims to thoroughly discuss the impact of AI on clinical practice in IC with special recognition of its most recent advancements.
Keywords: artificial intelligence (AI)interventional cardiology (IC)cardiac modalitiesaugmented and/or virtual realitiesautomatic medical systems
References
- Langlais ÉL, Thériault-Lauzier P, Marquis-Gravel G, et al. Novel artificial intelligence applications in cardiology: current landscape, limitations, and the road to real-world applications. J Cardiovasc Transl Res. 2023; 16(3): 513–525.
- Jaltotage B, Ihdayhid AR, Lan NSR, et al. Artificial intelligence in cardiology: an australian perspective. Heart Lung Circ. 2023; 32(8): 894–904.
- Lindholm D, Holzmann M. Machine learning for improved detection of myocardial infarction in patients presenting with chest pain in the emergency department. J Am Coll Cardiol. 2018; 71(11, Supl): A225.
- Azzalini L, Vilca LM, Lombardo F, et al. Incidence of contrast-induced acute kidney injury in a large cohort of all-comers undergoing percutaneous coronary intervention: Comparison of five contrast media. Int J Cardiol. 2018; 273: 69–73.
- Nakamura T, Sasano T. Artificial intelligence and cardiology: Current status and perspective. J Cardiol. 2022; 79(3): 326–333.
- Fearon WF, Achenbach S, Engstrom T, et al. Accuracy of fractional flow reserve derived from coronary angiography. Circulation. 2019; 139(4): 477–484.
- Du T, Xie L, Zhang H, et al. Training and validation of a deep learning architecture for the automatic analysis of coronary angiography. EuroIntervention. 2021; 17(1): 32–40.
- Garcia EV, Klein JL, Moncayo V, et al. Diagnostic performance of an artificial intelligence-driven cardiac-structured reporting system for myocardial perfusion SPECT imaging. J Nucl Cardiol. 2020; 27(5): 1652–1664.
- Zhang Q, Burrage MK, Lukaschuk E, et al. Toward replacing late gadolinium enhancement with artificial intelligence virtual native enhancement for gadolinium-free cardiovascular magnetic resonance tissue characterization in hypertrophic cardiomyopathy. Circulation. 2021; 144(8): 589–599.
- van de Leur RR, van Sleuwen MT, Zwetsloot PP, et al. Automatic triage of twelve-lead electrocardiograms using deep convolutional neural networks: a first implementation study. Eur Heart J - Digital Health. 2023.
- Sardar P, Abbott JD, Kundu A, et al. Impact of artificial intelligence on interventional cardiology: from decision-making aid to advanced interventional procedure assistance. JACC Cardiovasc Interv. 2019; 12(14): 1293–1303.
- Hashimoto D, Rosman G, Rus D, et al. Artificial intelligence in surgery: promises and perils. Ann Surg. 2018; 268(1): 70–76.
- Liu Z, Li W, Li H, et al. Automated deep neural network-based identification, localization, and tracking of cardiac structures for ultrasound-guided interventional surgery. J Thorac Dis. 2023; 15(4): 2129–2140.
- Giannis K, Thon C, Yang G, et al. Predicting 3D particles shapes based on 2D images by using convolutional neural network. Pow Tech. 2024; 432: 119122.
- Proniewska K, Khokhar AA, Dudek D. Advanced imaging in interventional cardiology: mixed reality to optimize preprocedural planning and intraprocedural monitoring. Kardiol Pol. 2021; 79(3): 331–335.
- Boopathiraja S, Punitha V, Kalavathi P, et al. Computational 2D and 3D medical image data compression models. Arch Comput Methods Eng. 2022; 29(2): 975–1007.
- PRISMA transparent reporting of systematic reviews and meta-analyses. http://www.prisma-statement.org/PRISMAStatement/PRISMAStatement?AspxAutoDetectCookieSupport=1.
- Yoneyama H, Nakajima K, Taki J, et al. Ability of artificial intelligence to diagnose coronary artery stenosis using hybrid images of coronary computed tomography angiography and myocardial perfusion SPECT. Eur J Hybrid Imaging. 2019; 3(1): 4.
- Kumar N, Kumar D. Machine learning based heart disease diagnosis using non-invasive methods: a review. J Physics: Conference Series. 2021; 1950(1): 012081.
- Koulaouzidis G, Jadczyk T, Iakovidis DK, et al. Artificial intelligence in cardiology-a narrative review of current status. J Clin Med. 2022; 11(13).
- Agatonovic-Kustrin S, Beresford R. Basic concepts of artificial neural network (ANN) modeling and its application in pharmaceutical research. J Pharm Biomed Anal. 2000; 22(5): 717–727.
- Knackstedt C, Bekkers SC, Schummers G, et al. Fully automated versus standard tracking of left ventricular ejection fraction and longitudinal strain: The FAST-EFs multicenter study. J Am Coll Cardiol. 2015; 66(13): 1456–1466.
- Narula S, Shameer K, Salem Omar AM, et al. Machine-learning algorithms to automate morphological and functional assessments in 2D echocardiography. J Am Coll Cardiol. 2016; 68(21): 2287–2295.
- Diller GP, Kempny A, Babu-Narayan SV, et al. Machine learning algorithms estimating prognosis and guiding therapy in adult congenital heart disease: data from a single tertiary centre including 10 019 patients. Eur Heart J. 2019; 40(13): 1069–1077.
- Ballinger B, Hsieh J, Singh A, et al. DeepHeart: semi-supervised sequence learning for cardiovascular risk prediction. Proceedings of the AAAI Conference on Artificial Intelligence. 2018; 32(1).
- Bahrami N, Retson T, Blansit K, et al. Automated selection of myocardial inversion time with a convolutional neural network: Spatial temporal ensemble myocardium inversion network (STEMI-NET). Magn Reson Med. 2019; 81(5): 3283–3291.
- Hasbullah S, Zahid MM, Mandala S. Detection of myocardial infarction using hybrid models of convolutional neural network and recurrent neural network. BioMedInformatics. 2023; 3(2): 478–492.
- Du T, Xie L, Zhang H, et al. Training and validation of a deep learning architecture for the automatic analysis of coronary angiography. EuroIntervention. 2021; 17(1): 32–40.
- Masuda Y, Ishikawa R, Tanaka T, et al. CNN-based fully automatic mitral valve extraction using CT images and existence probability maps. Phys Med Biol. 2024; 69(3).
- Seetharam K, Shrestha S, Sengupta PP. Cardiovascular imaging and intervention through the lens of artificial intelligence. Interv Cardiol. 2021; 16: e31.
- Madani A, Arnaout R, Mofrad M, et al. Fast and accurate view classification of echocardiograms using deep learning. NPJ Digit Med. 2018; 1.
- Cuocolo R, Perillo T, De Rosa E, et al. Current applications of big data and machine learning in cardiology. J Geriatr Cardiol. 2019; 16(8): 601–607.
- Akinyemi T, Omisore O, Du W, et al. Interventionalist hand motion recognition with convolutional neural network in robot-assisted coronary interventions. IEEE Sensors J. 2023; 23(15): 17725–17736.
- Maji P, Patra R, Dhibar K, et al. SNN based neuromorphic computing towards healthcare applications. IFIP Advances in Information and Communication Technology. 2023: 261–271.
- Chu H, Yan Y, Gan L, et al. A neuromorphic processing system with spike-driven SNN processor for wearable ECG classification. IEEE Trans Biomed Circuits Syst. 2022; 16(4): 511–523.
- Corradi F, Pande S, Stuijt J, et al. ECG-based heartbeat classification in neuromorphic hardware. 2019 International Joint Conference on Neural Networks (IJCNN). 2019.
- Kovacs P, Samiee K. Arrhythmia detection using spiking variable projection neural networks. Computing in Cardiology Conference (CinC). 2022: 4–7.
- Feng Y, Geng S, Chu J, et al. Building and training a deep spiking neural network for ECG classification. Biomedical Signal Processing and Control. 2022; 77: 103749.
- Miikkulainen R, Liang J, Meyerson E, et al. Artificial intelligence in the age of neural networks and brain computing. Academic Press. 2024: 269–287.
- Subhan S, Malik J, Haq AUl, et al. Role of artificial intelligence and machine learning in interventional cardiology. Curr Probl Cardiol. 2023; 48(7): 101698.
- Rajkomar A, Oren E, Chen K, et al. Scalable and accurate deep learning with electronic health records. NPJ Digit Med. 2018; 1: 18.
- Itu L, Rapaka S, Passerini T, et al. A machine-learning approach for computation of fractional flow reserve from coronary computed tomography. J Appl Physiol (1985). 2016; 121(1): 42–52.
- Coenen A, Lubbers MM, Kurata A, et al. Fractional flow reserve computed from noninvasive CT angiography data: diagnostic performance of an on-site clinician-operated computational fluid dynamics algorithm. Radiology. 2015; 274(3): 674–683.
- Joloudari JH, Saadatfar H, GhasemiGol M, et al. FCM-DNN: diagnosing coronary artery disease by deep accuracy fuzzy C-means clustering model. Math Biosci Eng. 2022; 19(4): 3609–3635.
- Bello GA, Dawes TJW, Duan J, et al. Deep learning cardiac motion analysis for human survival prediction. Nat Mach Intell. 2019; 1: 95–104.
- Ben Ali W, Pesaranghader A, Avram R, et al. Implementing machine learning in interventional cardiology: the benefits are worth the trouble. Front Cardiovasc Med. 2021; 8: 711401.
- Wolterink JM, Leiner T, Viergever MA, et al. Generative adversarial networks for noise reduction in low-dose CT. IEEE Trans Med Imaging. 2017; 36(12): 2536–2545.
- Kang E, Koo HJ, Yang DH, et al. Cycle-consistent adversarial denoising network for multiphase coronary CT angiography. Med Phys. 2019; 46(2): 550–562.
- Isin A, Ozdalili S. Cardiac arrhythmia detection using deep learning. Procedia Computer Science. 2017; 120: 268–275.
- Bavani B, Nirmala Sugirtha RS, Selvaraj NSR, et al. Design engineering classification of arrhythmia disease using enhanced RNN model. Design Engineering (Toronto). 2021; 2021: 4062–4072.
- Singh S, Pandey S, Pawar U, et al. Classification of ECG Arrhythmia using Recurrent Neural Networks. Procedia Computer Science. 2018; 132: 1290–1297.
- Sujadevi VG, Soman KP, Vinayakumar R. (editors). Real-Time Detection of Atrial Fibrillation from Short Time Single Lead ECG Traces Using Recurrent Neural Networks. Intelligent Systems Technologies and Applications. Springer International Publishing, Cham 2018.
- Ohta Y, Yunaga H, Kitao S, et al. Detection and classification of myocardial delayed enhancement patterns on MR images with deep neural networks: a feasibility study. Radiol Artif Intell. 2019; 1(3): e180061.
- Attia ZI, Kapa S, Lopez-Jimenez F, et al. Screening for cardiac contractile dysfunction using an artificial intelligence-enabled electrocardiogram. Nat Med. 2019; 25(1): 70–74.
- Zhao Y, Xiong J, Hou Y, et al. Early detection of ST-segment elevated myocardial infarction by artificial intelligence with 12-lead electrocardiogram. Int J Cardiol. 2020; 317: 223–230.
- Shaker A, Tantawi M, Shedeed H, et al. Generalization of convolutional neural networks for ECG classification using generative adversarial networks. IEEE Access. 2020; 8: 35592–35605.
- Yıldırım Ö, Pławiak P, Tan RS, et al. Arrhythmia detection using deep convolutional neural network with long duration ECG signals. Comput Biol Med. 2018; 102: 411–420.
- Acharya UR, Oh SL, Hagiwara Y, et al. A deep convolutional neural network model to classify heartbeats. Comput Biol Med. 2017; 89: 389–396.
- Mousavi S, Fotoohinasab A, Afghah F. Single-modal and multi-modal false arrhythmia alarm reduction using attention-based convolutional and recurrent neural networks. PLoS One. 2020; 15(1): e0226990.
- Oh SL, Ng EYK, Tan RuS, et al. Automated diagnosis of arrhythmia using combination of CNN and LSTM techniques with variable length heart beats. Comput Biol Med. 2018; 102: 278–287.
- Liu W, Huang Q, Chang S, et al. Multiple-feature-branch convolutional neural network for myocardial infarction diagnosis using electrocardiogram. Biomedical Signal Processing and Control. 2018; 45: 22–32.
- Acharya U, Fujita H, Lih O, et al. Automated detection of coronary artery disease using different durations of ECG segments with convolutional neural network. Knowledge-Based Syst. 2017; 132: 62–71.
- Santini G, Latta DD, Martini N, Valvano G, Gori A, Ripoli A. An automatic deep learning approach for coronary artery calcium segmentation. EMBEC & NBC 2017. Springer, Singapore 2018.
- Zreik M, Lessmann N, van Hamersvelt RW, et al. Deep learning analysis of the myocardium in coronary CT angiography for identification of patients with functionally significant coronary artery stenosis. Med Image Anal. 2018; 44: 72–85.
- Moon JH, Lee DaY, Cha WC, et al. Automatic stenosis recognition from coronary angiography using convolutional neural networks. Comput Methods Programs Biomed. 2021; 198: 105819.
- Ciusdel C, Turcea A, Puiu A, et al. Deep neural networks for ECG-free cardiac phase and end-diastolic frame detection on coronary angiographies. Comput Med Imaging Graph. 2020; 84: 101749.
- Ovalle-Magallanes E, Avina-Cervantes JG, Cruz-Aceves I, et al. Improving convolutional neural network learning based on a hierarchical bezier generative model for stenosis detection in X-ray images. Comput Methods Programs Biomed. 2022; 219: 106767.
- Liu X, Du T, Zhang H, et al. Detection and classification of chronic total occlusion lesions using deep learning. Annu Int Conf IEEE Eng Med Biol Soc. 2019; 2019: 828–831.
- Danilov VV, Klyshnikov KYu, Gerget OM, et al. Real-time coronary artery stenosis detection based on modern neural networks. Sci Rep. 2021; 11(1): 7582.
- Izci E, Ozdemir M, Degirmenci M, et al. Cardiac arrhythmia detection from 2D ECG images by using deep learning technique. 2019 Medical Technologies Congress (TIPTEKNO). 2019.
- Kaouter K, Mohamed T, Sofiene D, et al. Full training convolutional neural network for ECG signals classification. AIP Conference Proceedings. 2019.
- AL-Huseiny M, Abbas N, Sajit A. Diagnosis of arrhythmia based on ECG analysis using CNN. Bulletin of Electrical Engineering and Informatics. 2020; 9(3): 988–995.
- Kachuee M, Fazeli S, Sarrafzadeh M. ECG heartbeat classification: a deep transferable representation. 2018 IEEE International Conference on Healthcare Informatics (ICHI). 2018.
- Kamaleswaran R, Mahajan R, Akbilgic O. A robust deep convolutional neural network for the classification of abnormal cardiac rhythm using single lead electrocardiograms of variable length. Physiol Meas. 2018; 39(3): 035006.
- Baloglu U, Talo M, Yildirim O, et al. Classification of myocardial infarction with multi-lead ECG signals and deep CNN. Pattern Recognition Letters. 2019; 122: 23–30.
- Amirshahi A, Hashemi M. ECG Classification Algorithm Based on STDP and R-STDP Neural Networks for Real-Time Monitoring on Ultra Low-Power Personal Wearable Devices. IEEE Trans Biomed Circuits Syst. 2019; 13(6): 1483–1493.
- Xu SS, Mak MW, Cheung CC. Towards end-to-end ECG classification with raw signal extraction and deep neural networks. IEEE J Biomed Health Inform. 2019; 23(4): 1574–1584.
- Kwon JM, Kim KH, Jeon KiH, et al. Development and validation of deep-learning algorithm for electrocardiography-based heart failure identification. Korean Circ J. 2019; 49(7): 629–639.
- Pregowska Agnieszka and Perkins Mark. Artificial Intelligence in Medical Education: Technology and Ethical Risk. Available at SSRN. https://ssrn.com/abstract=4643763 or http://dx.doi.org/10.2139/ssrn.4643763 (November 24, 2023).
- Leonelli S. Learning from Data Journeys. In: Leonelli S, Temini N, editors. Data Journeys in the Sciences. Sringer International Publishing, Cham 2020: 1–24.
- Thirumalaikolundusubramanian P, Meenakshisundaram R, Senthilkumaran S. Ethics, legality, and education in the practice of cardiology. Heart Toxins. 2015: 595–623.
- Zicari R, Brusseau J, Blomberg S, et al. On assessing trustworthy AI in healthcare. Machine learning as a supportive tool to recognize cardiac arrest in emergency calls. Frontiers in Human Dynamics. 2021; 3.
- Coorey G, Figtree GA, Fletcher DF, et al. The health digital twin to tackle cardiovascular disease-a review of an emerging interdisciplinary field. NPJ Digit Med. 2022; 5(1): 126.
- Ledziński Ł, Grześk G. Artificial Intelligence as an Emerging Tool for Cardiologists. The 2nd International Electronic Conference on Biomedicines. Medical Sciences Forum. 2023; 21(1).
- Monlezun DJ, Sinyavskiy O, Peters N, et al. Artificial intelligence-augmented propensity score, cost effectiveness and computational ethical analysis of cardiac arrest and active cancer with novel mortality predictive score. Medicina (Kaunas). 2022; 58(8).
- Aqel S, Syaj S, Al-Bzour A, et al. Artificial intelligence and machine learning applications in sudden cardiac arrest prediction and management: a comprehensive review. Curr Cardiol Rep. 2023; 25(11): 1391–1396.
- Braun M. Represent me: please! Towards an ethics of digital twins in medicine. J Med Ethics. 2021 [Epub ahead of print].
- Chamola V, Hassija V, Sulthana A, et al. A review of trustworthy and explainable artificial intelligence (XAI). IEEE Access. 2023; 11: 78994–79015.
- Rachovitsa A, Johann N. The human rights implications of the use of AI in the digital welfare state: lessons learned from the Dutch SyRI Case. Human Rights Law Review. 2022; 22(2): 1–15.
- Assessment List for Trustworthy Artificial Intelligence (ALTAI) for self-assessment.
- Artificial intelligence ac.
- Floridi L. Establishing the rules for building trustworthy AI. Nature Machine Intelligence. 2019; 1(6): 261–262.
- The three challenges of AI regulation. https://www.brookings.edu/articles/the-three-challenges-of-ai-regulation.
- Zhang K, Gao Y, Lv J, et al. Artificial intelligence-based spiral CT 3D reconstruction in transcatheter aortic valve implantation. Comput Math Methods Med. 2022; 2022: 5794681.
- Banerjee A, Camps J, Zacur E, et al. A completely automated pipeline for 3D reconstruction of human heart from 2D cine magnetic resonance slices. Philos Trans A Math Phys Eng Sci. 2021; 379(2212): 20200257.
- Baur C, Milletari F, Belagiannis V, et al. Automatic 3D reconstruction of electrophysiology catheters from two-view monoplane C-arm image sequences. Int J Comput Assist Radiol Surg. 2016; 11(7): 1319–1328.
- Mørup SD, Stowe J, Precht H, et al. Design of a 3D printed coronary artery model for CT optimization. Radiography (Lond). 2022; 28(2): 426–432.
- Jiang B, Guo N, Ge Y, et al. Development and application of artificial intelligence in cardiac imaging. Br J Radiol. 2020; 93(1113): 20190812.
- Yasmin F, Shah SM, Naeem A, et al. Artificial intelligence in the diagnosis and detection of heart failure: the past, present, and future. Rev Cardiovasc Med. 2021; 22(4): 1095–1113.
- Kanjee Z, Crowe B, Rodman A. Accuracy of a generative artificial intelligence model in a complex diagnostic challenge. JAMA. 2023; 330(1): 78–80.
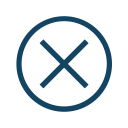