Impact of non-cardiovascular disease burden on thirty-day hospital readmission in heart failure patients
Abstract
Background: Little is known about the impact of non-cardiovascular disease (CVD) burden on 30- -day readmission in heart failure (HF) patients. The aim of the study was to assess the role of non-CVD burden on 30-day readmission in HF patients. \
Methods: We analyzed the effect of non-CVD burden by frequency of ICD-9 code categories on readmissions of patients discharged with a primary diagnosis of HF. We first modeled the probability of readmission within 30 days as a function of demographic and clinical covariates in a randomly selected training dataset of the total cohort. Variable selection was carried out using a bootstrap LASSO procedure with 1000 bootstrap samples, the final model was tested on a validation dataset. Adjusted odds ratios and confidence intervals were reported in the validation dataset.
Results: There were a total of 6228 HF hospitalizations, 1523 (24%) with readmission within 30 days of discharge. The strongest predictor for 30-day readmissions was any hospital admission in the prior year (p < 0.001). Cardiovascular risk factors did not enter the final model. However, digestive system diseases increased the risk for readmission by 17% for each diagnosis (p = 0.046), while respiratory diseases and genitourinary diseases showed a trend toward a higher risk of readmission (p = 0.07 and p = 0.09, respectively). Non-CVDs out-competed cardiovascular covariates previously reported to predict readmission.
Conclusions: In patients with HF hospitalization, prior admissions predicted 30-day readmission. Diseases of the digestive system also increase 30-day readmission rates. Assessment of non-CVD burden in HF patients could serve as an important risk marker for 30-day readmissions.
Keywords: heart failure readmissionnon-cardiovascular disease burdenpredictive model
References
- Go A, Mozaffarian D, Roger V, et al. Heart disease and stroke statistics — 2014 update. Circulation. 2014; 129(3): e28–e292.
- Ross JS, Chen J, Lin Z, et al. Recent national trends in readmission rates after heart failure hospitalization. Circ Heart Fail. 2010; 3(1): 97–103.
- Dharmarajan K, Wang Y, Lin Z, et al. Association of changing hospital readmission rates with mortality rates after hospital discharge. JAMA. 2017; 318(3): 270–278.
- Krumholz H, Lin Z, Keenan P, et al. Relationship between hospital readmission and mortality rates for patients hospitalized with acute myocardial infarction, heart failure, or pneumonia. JAMA. 2013; 309(6): 587–593.
- Rathore SS, Masoudi FA, Wang Y, et al. Socioeconomic status, treatment, and outcomes among elderly patients hospitalized with heart failure: findings from the National Heart Failure Project. Am Heart J. 2006; 152(2): 371–378.
- Kutyifa V, Moss AJ, Klein H, et al. Use of the wearable cardioverter defibrillator in high-risk cardiac patients: data from the Prospective Registry of Patients Using the Wearable Cardioverter Defibrillator (WEARIT-II Registry). Circulation. 2015; 132(17): 1613–1619.
- Team RCR. A Language and Environment for Statistical Computing. Vienna, Austria: R Foundation for Statistical Computing. 2015.
- Fitzmaurice GM, Laird NM, Ware H. Applied longitudinal analysis Hoboken, N J. 2004.
- Efron B. Bootstrap Methods: Another Look at the Jackknife. Ann Statist. 1979; 7(1): 1–26.
- Tibshirani R. Regression shrinkage and selection via the Lasso. J Roy Stat Soc B Met. 1996; 58: 267–88.
- Bristow M, Saxon L, Boehmer J, et al. Cardiac-Resynchronization therapy with or without an implantable defibrillator in advanced chronic heart failure. N Engl J Med. 2004; 350(21): 2140–2150.
- Keenan PS, Normand SLT, Lin Z, et al. An administrative claims measure suitable for profiling hospital performance on the basis of 30-day all-cause readmission rates among patients with heart failure. Circ Cardiovasc Qual Outcomes. 2008; 1(1): 29–37.
- Krumholz HM, Chen YT, Wang Y, et al. Predictors of readmission among elderly survivors of admission with heart failure. Am Heart J. 2000; 139(1 Pt 1): 72–77.
- Davis JD, Olsen MA, Bommarito K, et al. All-Payer analysis of heart failure hospitalization 30-day readmission: comorbidities matter. Am J Med. 2017; 130(1): 93.e9–93.e28.
- Chen LM, Jha AK, Guterman S, et al. Hospital cost of care, quality of care, and readmission rates: penny wise and pound foolish? Arch Intern Med. 2010; 170(4): 340–346.
- Tokatli A, Ural D. Previous hospitalizations predict both hospital readmissions and mortality in patients with heart failure. Cardiol J. 2016; 23(2): 224–224.
- Swindle JP, Chan WW, Waltman Johnson K, et al. Evaluation of mortality and readmissions following hospitalization with heart failure. Curr Med Res Opin. 2016 [Epub ahead of print]: 1–11.
- Desai A, Stevenson L. Rehospitalization for heart failure. Circulation. 2012; 126(4): 501–506.
- Sherer AP, Crane PB, Abel WM, et al. Predicting heart failure readmissions. J Cardiovasc Nurs. 2016; 31(2): 114–120.
- Rigolli M, Whalley GA. Heart failure with preserved ejection fraction. J Geriatr Cardiol. 2013; 10(4): 369–376.
- Sharma K, Kass DA. Heart failure with preserved ejection fraction: mechanisms, clinical features, and therapies. Circ Res. 2014; 115(1): 79–96.
- Vasko MR, Cartwright DB, Knochel JP, et al. Furosemide absorption altered in decompensated congestive heart failure. Ann Intern Med. 1985; 102(3): 314–318.
- Sica DA, Sica DA. Drug absorption in congestive heart failure: impact on management. Prog Cardiovasc Nurs. 1999; 14(1): 30–32.
- Smilde TDJ, Hillege HL, Voors AA, et al. Prognostic importance of renal function in patients with early heart failure and mild left ventricular dysfunction. Am J Cardiol. 2004; 94(2): 240–243.
- Lang CC, Mancini DM. Non-cardiac comorbidities in chronic heart failure. Heart. 2007; 93(6): 665–671.
- Liang KV, Williams AW, Greene EL, et al. Acute decompensated heart failure and the cardiorenal syndrome. Crit Care Med. 2008; 36(1 Suppl): S75–S88.
- Ross JS, Mulvey GK, Stauffer B, et al. Statistical models and patient predictors of readmission for heart failure: a systematic review. Arch Intern Med. 2008; 168(13): 1371–1386.
- Kansagara D, Englander H, Salanitro A, et al. Risk prediction models for hospital readmission: a systematic review. JAMA. 2011; 306(15): 1688–1698.
- Frizzell JD, Liang Li, Schulte PJ, et al. Prediction of 30-day all-cause readmissions in patients hospitalized for heart failure: comparison of machine learning and other statistical approaches. JAMA Cardiol. 2017; 2(2): 204–209.
- Krumholz H, Bernheim S. The Role of Socioeconomic Status in Hospital Outcomes Measures. Ann Intern Med. 2015; 162(9): 670.
- Priori SG, Blomstrom-Lundqvist C, Mazzanti A, et al. 2015 ESC Guidelines for the management of patients with ventricular arrhythmias and the prevention of sudden cardiac death: The Task Force for the Management of Patients with Ventricular Arrhythmias and the Prevention of Sudden Cardiac Death of the European Society of Cardiology (ESC). Endorsed by: Association for European Paediatric and Congenital Cardiology (AEPC). Eur Heart J. 2015; 36: 2793–2867.
- Huynh Q, Negishi K, Blizzard L, et al. Predictive score for 30-day readmission or death in heart failure. JAMA Cardiol. 2016; 1(3): 362–364.
- Calvillo-King L, Arnold D, Eubank KJ, et al. Impact of social factors on risk of readmission or mortality in pneumonia and heart failure: systematic review. J Gen Intern Med. 2013; 28(2): 269–282.
- Mesquita ET, Cruz LN, Mariano BM, et al. Post-Hospital syndrome: a new challenge in cardiovascular practice. Arq Bras Cardiol. 2015; 105(5): 540–544.
- Krumholz HM. Post-hospital syndrome--an acquired, transient condition of generalized risk. N Engl J Med. 2013; 368(2): 100–102.
- Khan H, Kalogeropoulos AP, Georgiopoulou VV, et al. Frailty and risk for heart failure in older adults: the health, aging, and body composition study. Am Heart J. 2013; 166(5): 887–894.
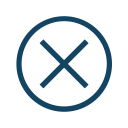